Grid-Gcn For Fast And Scalable Point Cloud Learning
2020 IEEE/CVF CONFERENCE ON COMPUTER VISION AND PATTERN RECOGNITION (CVPR)(2020)
摘要
Due to the sparsity and irregularity of the point cloud data, methods that directly consume points have become popular. Among all point-based models, graph convolutional networks (GCN) lead to notable performance by fully preserving the data granularity and exploiting point interrelation. However, point-based networks spend a significant amount of time on data structuring (e.g., Farthest Point Sampling (FPS) and neighbor points querying), which limit the speed and scalability. In this paper, we present a method, named Grid-GCN, for fast and scalable point cloud learning. Grid-GCN uses a novel data structuring strategy, Coverage-Aware Grid Query (CAGQ). By leveraging the efficiency of grid space, CAGQ improves spatial coverage while reducing the theoretical time complexity. Compared with popular sampling methods such as Farthest Point Sampling (FPS) and Ball Query, CAGQ achieves up to 50x speed-up. With a Grid Context Aggregation (GCA) module, Grid-GCN achieves state-of-the-art performance on major point cloud classification and segmentation benchmarks with significantly faster runtime than previous studies. Remarkably, Grid-GCN achieves the inference speed of 50fps on ScanNet using 81920 points as input. The supplementary(1) and the code(2) are released.
更多查看译文
关键词
grid context aggregation module,theoretical time complexity,coverage-aware grid query,data structuring strategy,point cloud data models,grid-GCN,FPS,image segmentation,point cloud classification,farthest point sampling,CAGQ,point-based networks,data granularity,graph convolutional networks,point cloud learning
AI 理解论文
溯源树
样例
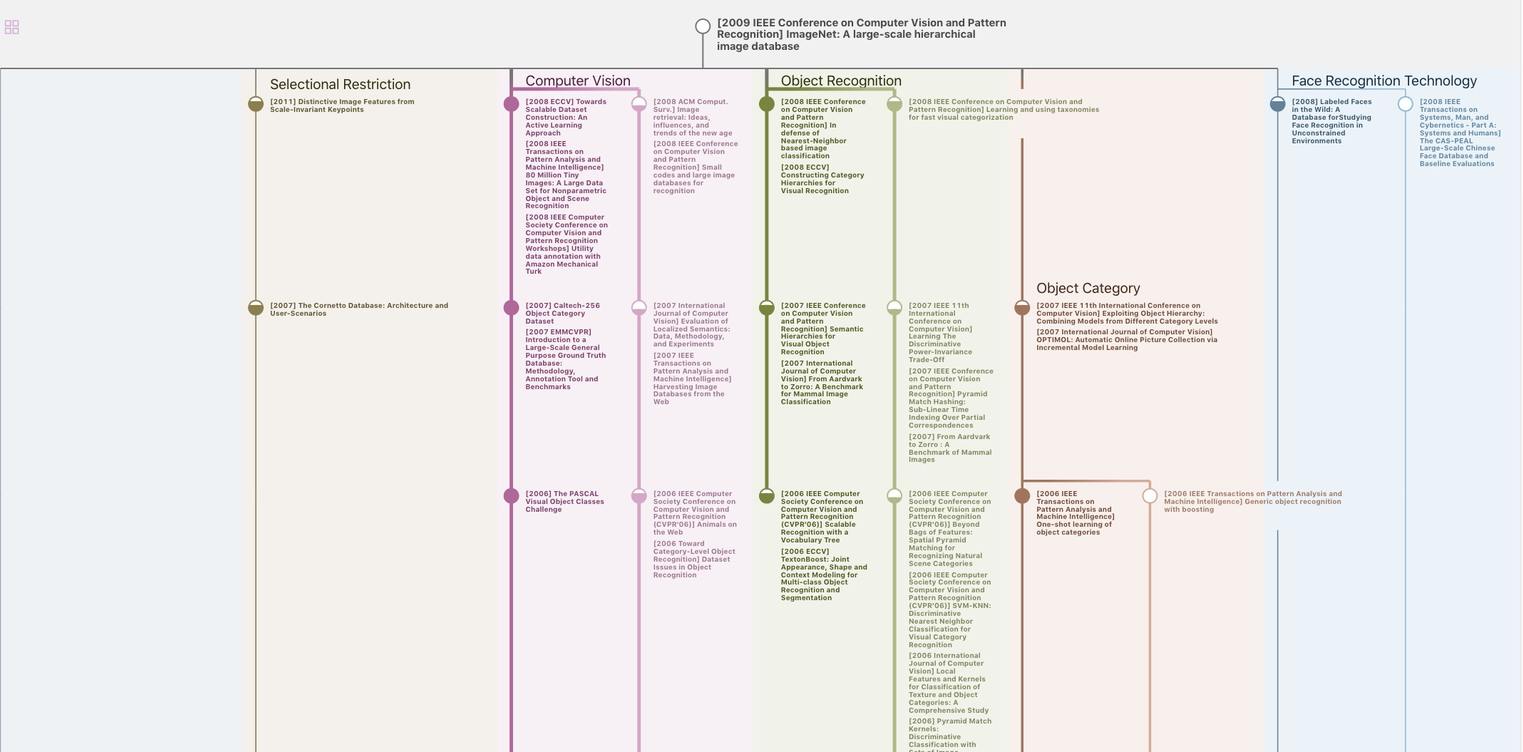
生成溯源树,研究论文发展脉络
Chat Paper
正在生成论文摘要