Online Ecg-Based Features For Cognitive Load Assessment
2019 IEEE INTERNATIONAL CONFERENCE ON SYSTEMS, MAN AND CYBERNETICS (SMC)(2019)
摘要
This study was concerned with the development and testing of online cognitive-load monitoring methods by means of a working-memory experiment using electrocardiogram (ECG) analyses for future applications in mixed-initiative human-machine interaction (HMI). To this end, we first identified potentially reliable cognitive-workload-related cardiac metrics and algorithms for online processing. We then compared our online results to those conventionally obtained with state-of-the-art offline methods. Finally, we evaluated the possibility of classifying low versus high working-memory load using different classification algorithms. Our results show that both offline and online methods reliably estimate the workload associated with a multi-level working-memory task at the group level, whether it is with the heart rhythm or the heart rate variation (standard deviation of the RR interval). Moreover, we found significant working-memory load classification accuracy using both two-dimensional linear discriminant analyses (LDA) or a support vector machine (SVM). We hence argue that our online algorithm is reliable enough to provide online electrocardiographic metrics as a tool for real-life workload evaluation and can be a valuable feature for mixed-initiative systems.
更多查看译文
关键词
online ECG-based features,cognitive load assessment,cognitive-load monitoring methods,electrocardiogram analyses,human-machine interaction,potentially reliable cognitive-workload-related cardiac metrics,multilevel working-memory task,heart rhythm,heart rate variation,two-dimensional linear discriminant analyses,support vector machine,online algorithm,online electrocardiographic metrics,real-life workload evaluation,mixed-initiative systems,working-memory load classification
AI 理解论文
溯源树
样例
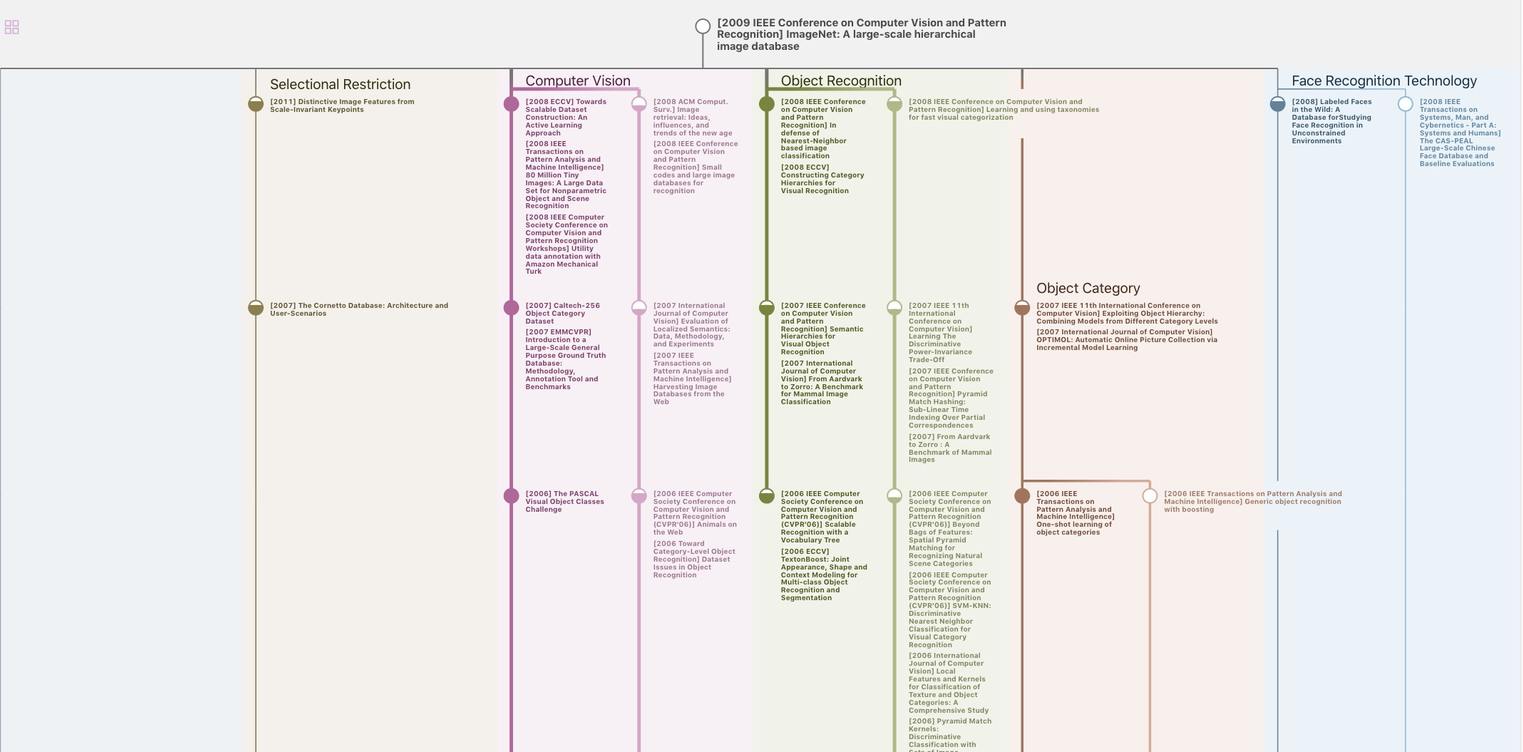
生成溯源树,研究论文发展脉络
Chat Paper
正在生成论文摘要