Unveiling the principle descriptor for predicting the electron inelastic mean free path based on a machine learning framework.
SCIENCE AND TECHNOLOGY OF ADVANCED MATERIALS(2019)
摘要
The TPP-2M formula is the most popular empirical formula for the estimation of the electron inelastic mean free paths (IMFPs) in solids from several simple material parameters. The TPP-2M formula, however, poorly describes several materials because it relies heavily on the traditional least-squares analysis. Herein, we propose a new framework based on machine learning to overcome the weakness. This framework allows a selection from an enormous number of combined terms (descriptors) to build a new formula that describes the electron IMFPs. The resulting framework not only provides higher average accuracy and stability but also reveals the physics meanings of several newly found descriptors. Using the identified principle descriptors, a complete physics picture of electron IMFPs is obtained, including both single and collective electron behaviors of inelastic scattering. Our findings suggest that machine learning is robust and efficient to predict the IMFP and has great potential in building a regression framework for data-driven problems. Furthermore, this method could be applicable to find empirical formula for given experimental data using a series of parameters given a priori, holds potential to find a deeper connection between experimental data and a priori parameters.
更多查看译文
关键词
Surface science,machine learning,inelastic mean free path,the Least Absolute Shrinkage and Selection Operator (LASSO)
AI 理解论文
溯源树
样例
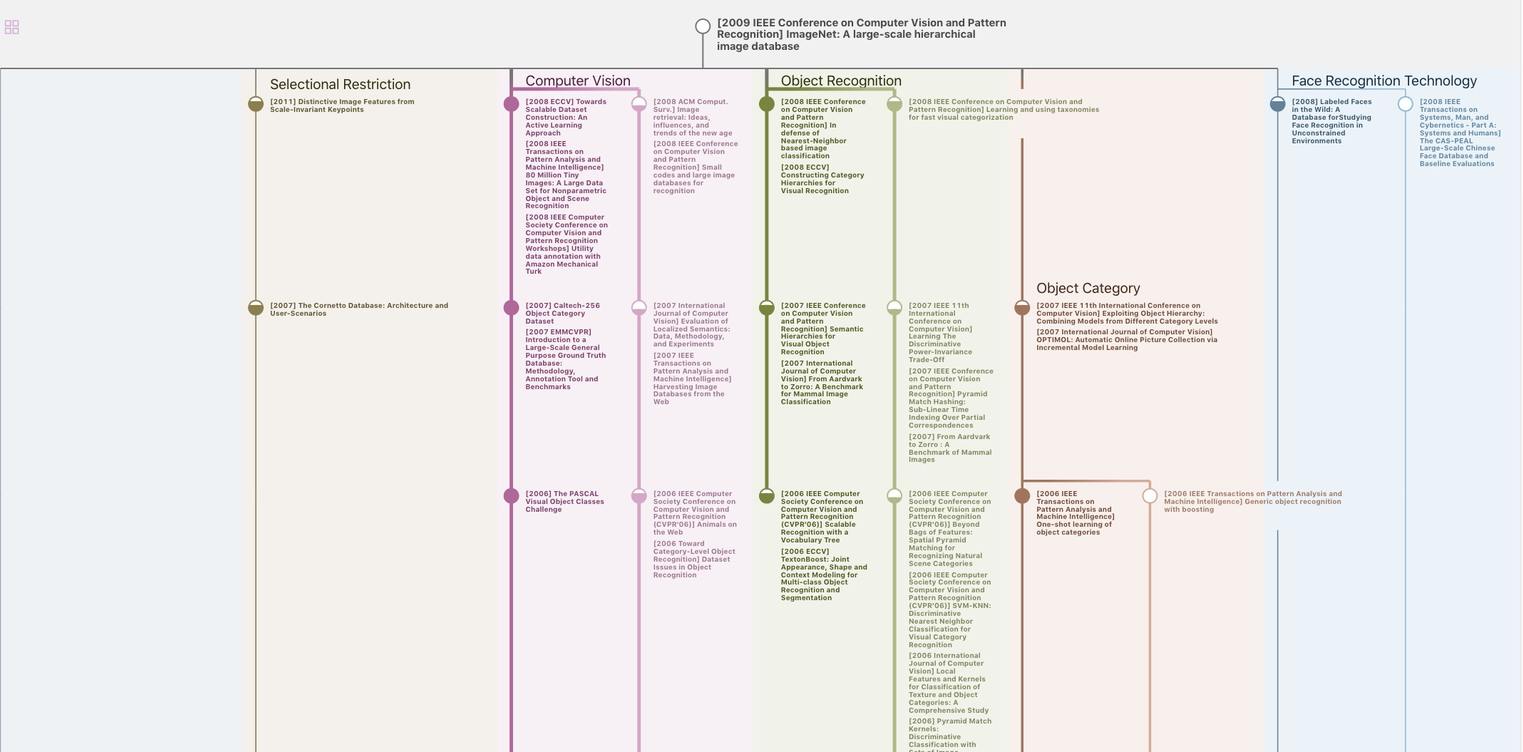
生成溯源树,研究论文发展脉络
Chat Paper
正在生成论文摘要