Advertiser-Assisted Behaviora Ad-Targeting Via Denoised Distribution Induction
2019 IEEE INTERNATIONAL CONFERENCE ON BIG DATA (BIG DATA)(2019)
摘要
Nowadays, advertising (ad) deliveries are conducted in a targeted manner to improve their effectiveness and efficiency. However, human behavior data in ad-platforms such as Web browsing history is complex and contains a lot of "noise". On the other hand, information in the advertiser's domain (e.g. e-commerce sites) seems to contain less noise (e.g. product browsing history) with respect to ad-targeting. We introduce a new denoising method for behavioral ad-targeting based on the idea of feature distribution alignment induced by the advertiser's domain. This denoised distribution induction can be achieved by employing domain adversarial training with stabilization techniques. We evaluate our model on real world data originating from an e-commerce site and an ad platform. The results of an ablation study have demonstrated the advantage of utilizing an advertiser's domain for denoising human behavior data of an ad-platform domain.
更多查看译文
关键词
behavioral targeting, transfer learning, domain adversarial training, variational discriminator bottleneck, mixup
AI 理解论文
溯源树
样例
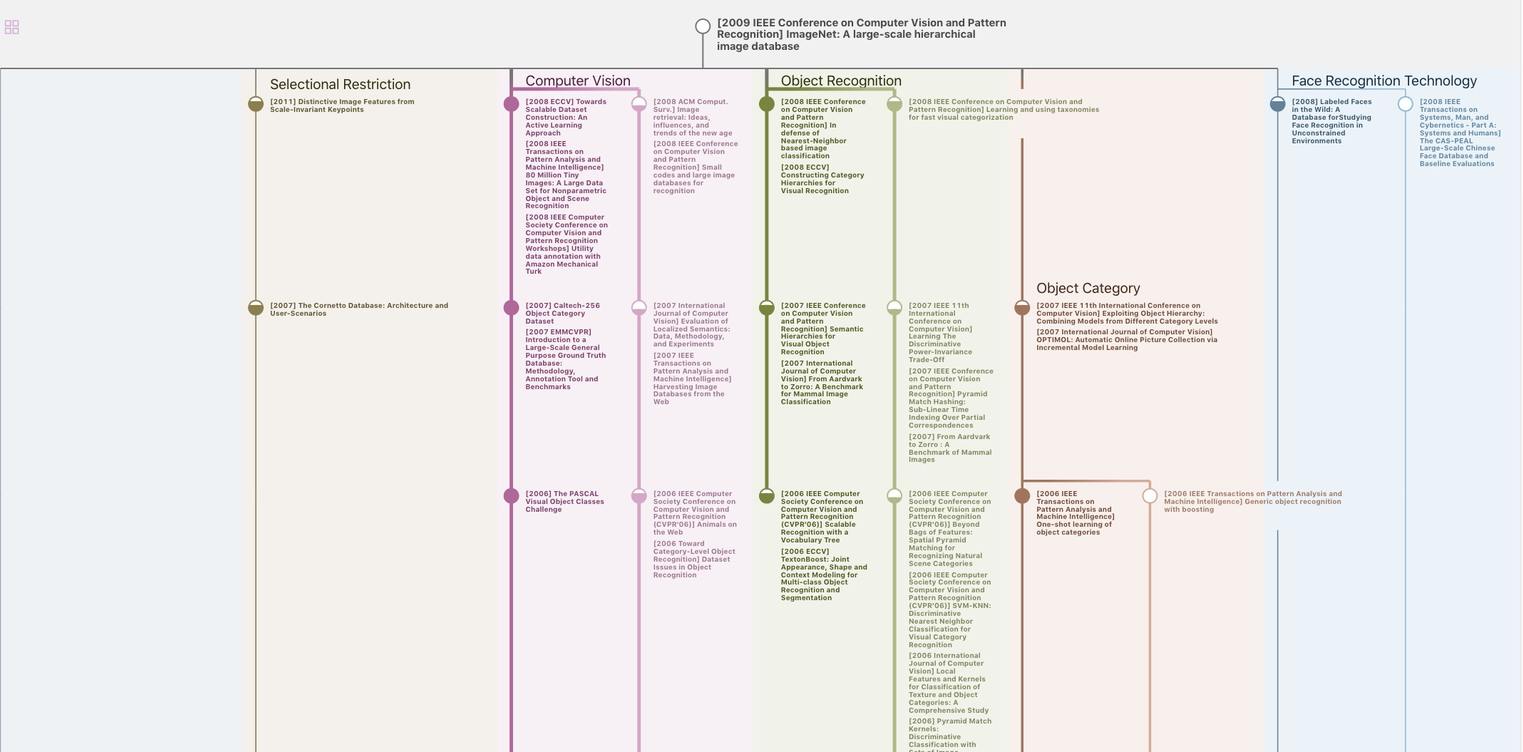
生成溯源树,研究论文发展脉络
Chat Paper
正在生成论文摘要