DeepCarotene -Job Title Classification with Multi-stream Convolutional Neural Network
2019 IEEE INTERNATIONAL CONFERENCE ON BIG DATA (BIG DATA)(2019)
摘要
In online recruitment, job title classification is a fundamental task that enables several downstream applications like job recommendation and ranking for job search. A special case of multi-class text classification, the job title classification problem takes as input two components from a job posting -a short job title and a lengthier job description, and normalizes the raw job title into its closest match from the given taxonomy. Typically, the job title, though shorter in length, contains more targeted signals than the job description, that can contain additional information irrelevant to the context. On the other hand, the job description often provides valuable information that helps steer the classification model towards choosing the best match. Achieving a balance between the two components is not a trivial task. In this paper, we propose a multi-stream CNN based model for job title classification, that learns semantic features on both character and word level. We collected about 15 million data points from one of the largest online job boards, Careerbuilder, to train the model. Due to the universal problem of getting massive labeled data, we adopt a weakly supervised method to efficiently generate noisy labels for this large data set. Compared with the current state-of-the-art job title classification systems, the proposed model, DeepCarotene, shows a significant improvement in performance. This model provides a new direction of CNN based end-to-end approach for job title classification.
更多查看译文
关键词
multistream convolutional neural network,job recommendation,job search,multiclass text classification,job title classification problem,job posting,job description,raw job title,online job boards,DeepCarotene,CNN
AI 理解论文
溯源树
样例
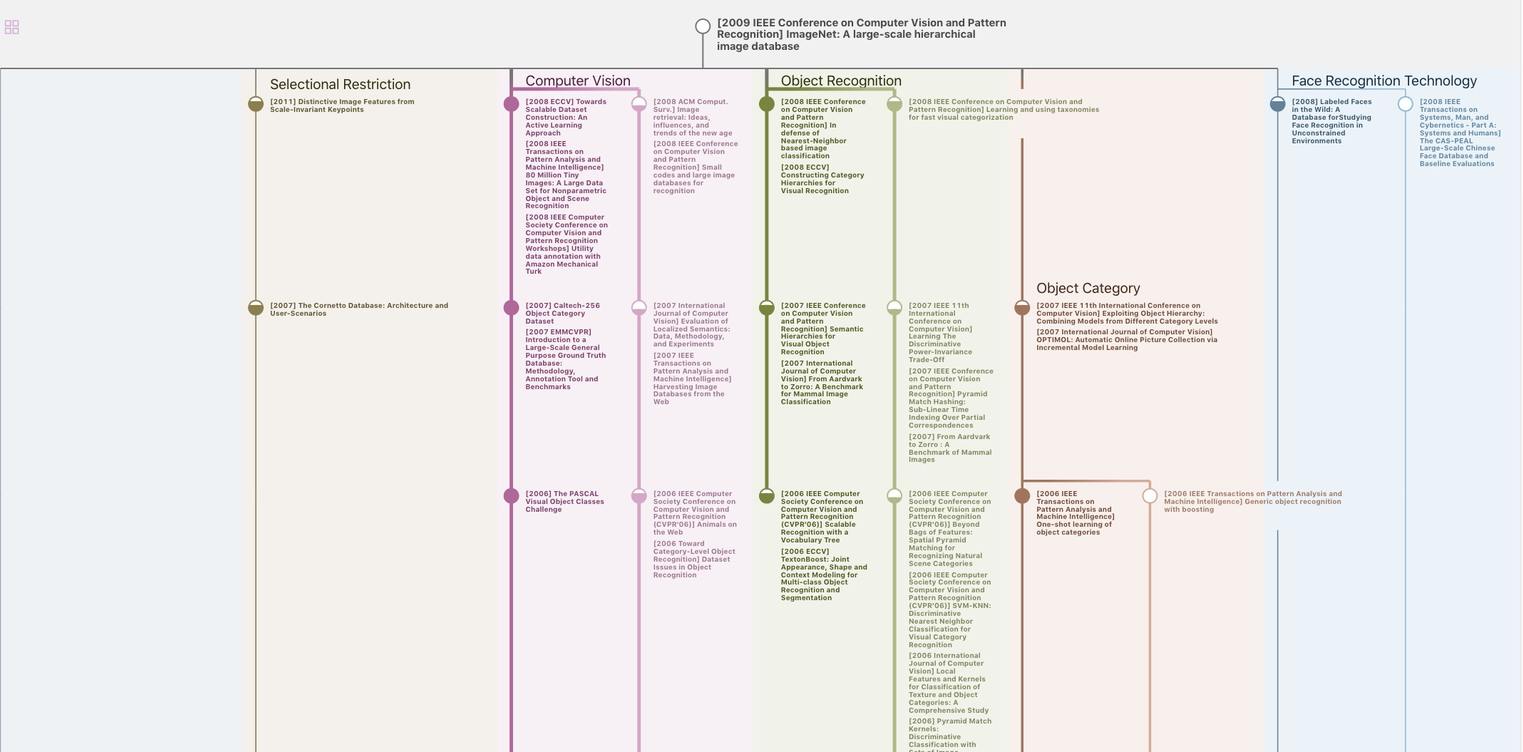
生成溯源树,研究论文发展脉络
Chat Paper
正在生成论文摘要