Unsupervised Conditional Adversarial Networks for Tax Evasion Detection
user-5ca99f0c530c702a92b1df51(2019)
摘要
The identification of tax evasion plays an important role in ensuring tax order, promoting the level of tax collection and management, and reducing tax losses. With the advancements in data mining technology, many machine learning techniques have yielded results in identifying tax evasion. However, to realize satisfactory performance, these models require large amounts of human annotated data. In the tax field, unlabeled tax data are abundant, data annotation in a single region is expensive, and the distributions of characteristics differ among regions; these factors pose substantial difficulties in the development of an identification model. Existing tax evasion detection methods are either trained for single-region tasks, in which case they perform poorly on inter-region tax evasion identification due to the discrepancies in feature distributions, or utilize labeled data from both the target-task field and different but related auxiliary fields to reuse and transfer knowledge of the target domain data, in which case they cannot deal with scenarios in which there are no labeled data in target audit tasks. Although current unsupervised transfer learning techniques can train models in labeled regions for unlabeled regions, large intraclass distribution discrepancies cannot be perfectly minimized in tax evasion detection scenarios. To better address the above challenges, this paper proposes a general architecture, namely, the unsupervised conditional adversarial networks (UCAN) for tax evasion detection, which is the first approach to solve audit tasks in unlabeled target domains via inter-region transfer. Our architecture establishes an adversarial neural network adding label information in the distribution adapter, which can granularly adapt the joint probability distribution (JPD) of the data. We introduce a constraint that is based on the conditional maximum mean discrepancy (CMMD) of the extracted features to align the conditional probability distribution (CPD) of the deep representation. Our model is formed by combining the distribution adapter and the label predictor to realize end-toend learning of unsupervised feature transfer. The experimental results demonstrate the outstanding performance of our model in all migration tasks compared with state-of-the-art approaches.
更多查看译文
关键词
Index Terms-Unsupervised Transductive Transfer Learning,Tax Evasion Detection,Distribution Adaptation,Adversarial Networks
AI 理解论文
溯源树
样例
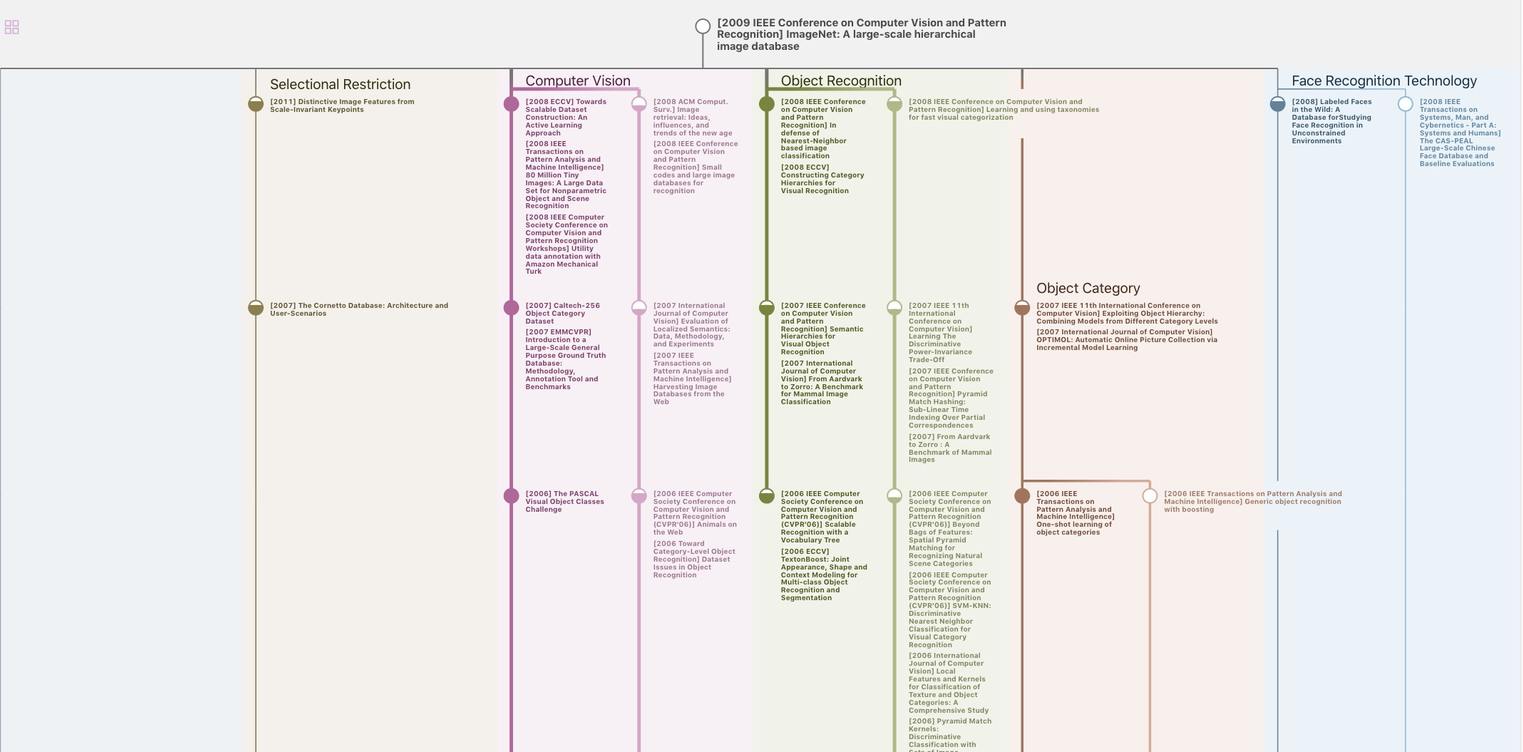
生成溯源树,研究论文发展脉络
Chat Paper
正在生成论文摘要