Tedm-Pu: A Tax Evasion Detection Method Based On Positive And Unlabeled Learning
2019 IEEE INTERNATIONAL CONFERENCE ON BIG DATA (BIG DATA)(2019)
摘要
Tax evasion detection plays a crucial role in reducing tax revenue loss and many efforts have been made to develop detection models based on machine learning techniques. To train an effective model to detect tax evaders, a large amount of data is required, especially sufficient labeled data. However, the expensive and time-consuming annotation process results in small amount of labeled data being available, which makes the development of detection models difficult. To address this issue, we propose a tax evasion detection method based on positive and unlabeled learning (TEDM-PU), to identify tax evasion by utilizing limited annotated tax evasion taxpayers and a large amount of unlabeled data. The TEDM-PU framework consists of three stages: a preprocessing stage extracting taxpayer features based on random forest, a pseudo labeling stage assigning pseudo labels to unlabeled samples based on PUAdapter, and a model training stage based on LightGBM method. To evaluate the effectiveness of our proposed TEDM-PU, we conduct experimental tests on real-world tax data. The results demonstrate that TEDM-PU method can detect tax evaders with higher accuracy and better interpretability than state-of-the-art methods.
更多查看译文
关键词
tax evasion detection, PU learning, interpretability
AI 理解论文
溯源树
样例
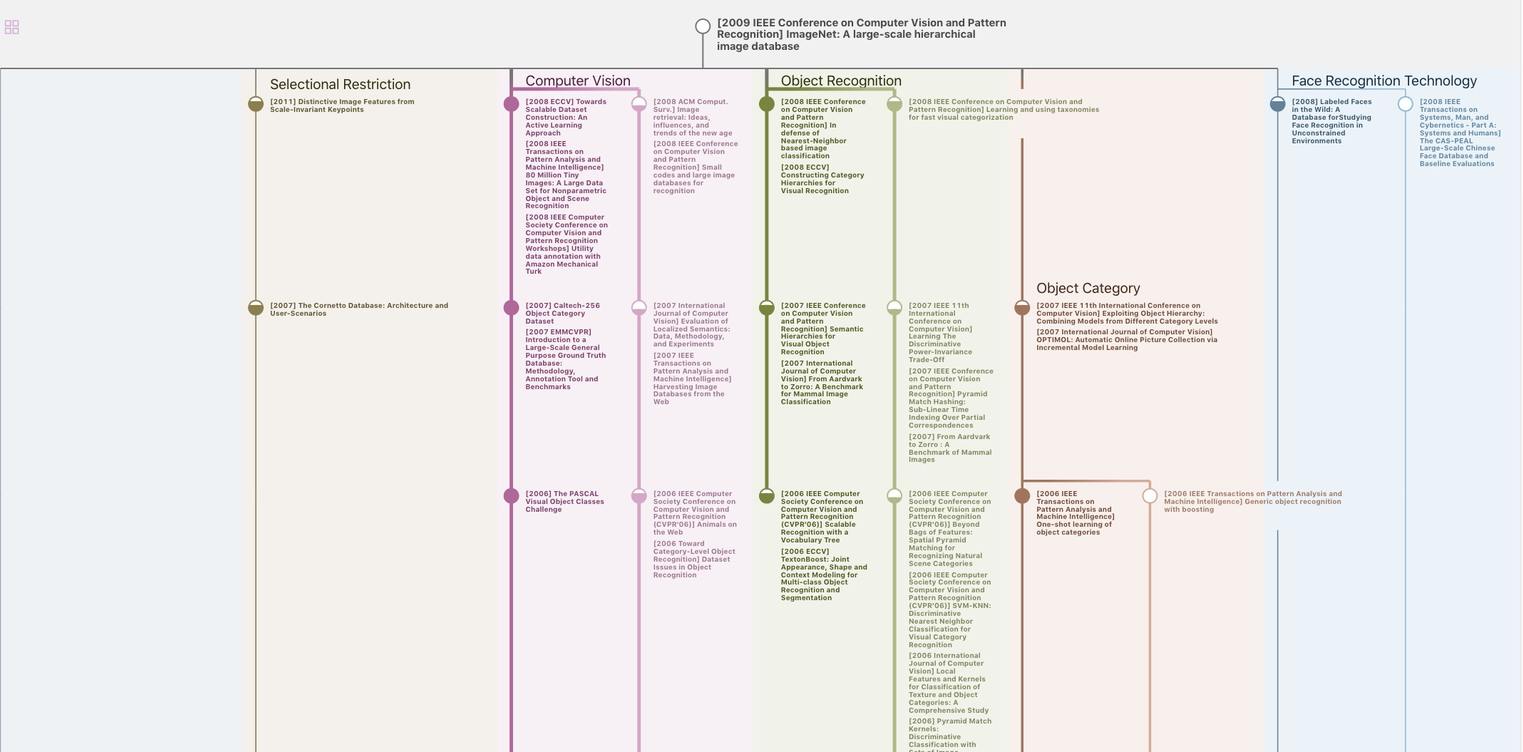
生成溯源树,研究论文发展脉络
Chat Paper
正在生成论文摘要