Deep Contextualized Acoustic Representations For Semi-Supervised Speech Recognition
2020 IEEE INTERNATIONAL CONFERENCE ON ACOUSTICS, SPEECH, AND SIGNAL PROCESSING(2019)
摘要
We propose a novel approach to semi-supervised automatic speech recognition (ASR). We first exploit a large amount of unlabeled audio data via representation learning, where we reconstruct a temporal slice of filterbank features from past and future context frames. The resulting deep contextualized acoustic representations (DeCoAR) are then used to train a CTC-based end-to-end ASR system using a smaller amount of labeled audio data. In our experiments, we show that systems trained on DeCoAR consistently outperform ones trained on conventional filterbank features, giving 42% and 19% relative improvement over the baseline on WSJ eval92 and LibriSpeech test-clean, respectively. Our approach can drastically reduce the amount of labeled data required; unsupervised training on LibriSpeech then supervision with 100 hours of labeled data achieves performance on par with training on all 960 hours directly.
更多查看译文
关键词
speech recognition, acoustic representation learning, semi-supervised learning
AI 理解论文
溯源树
样例
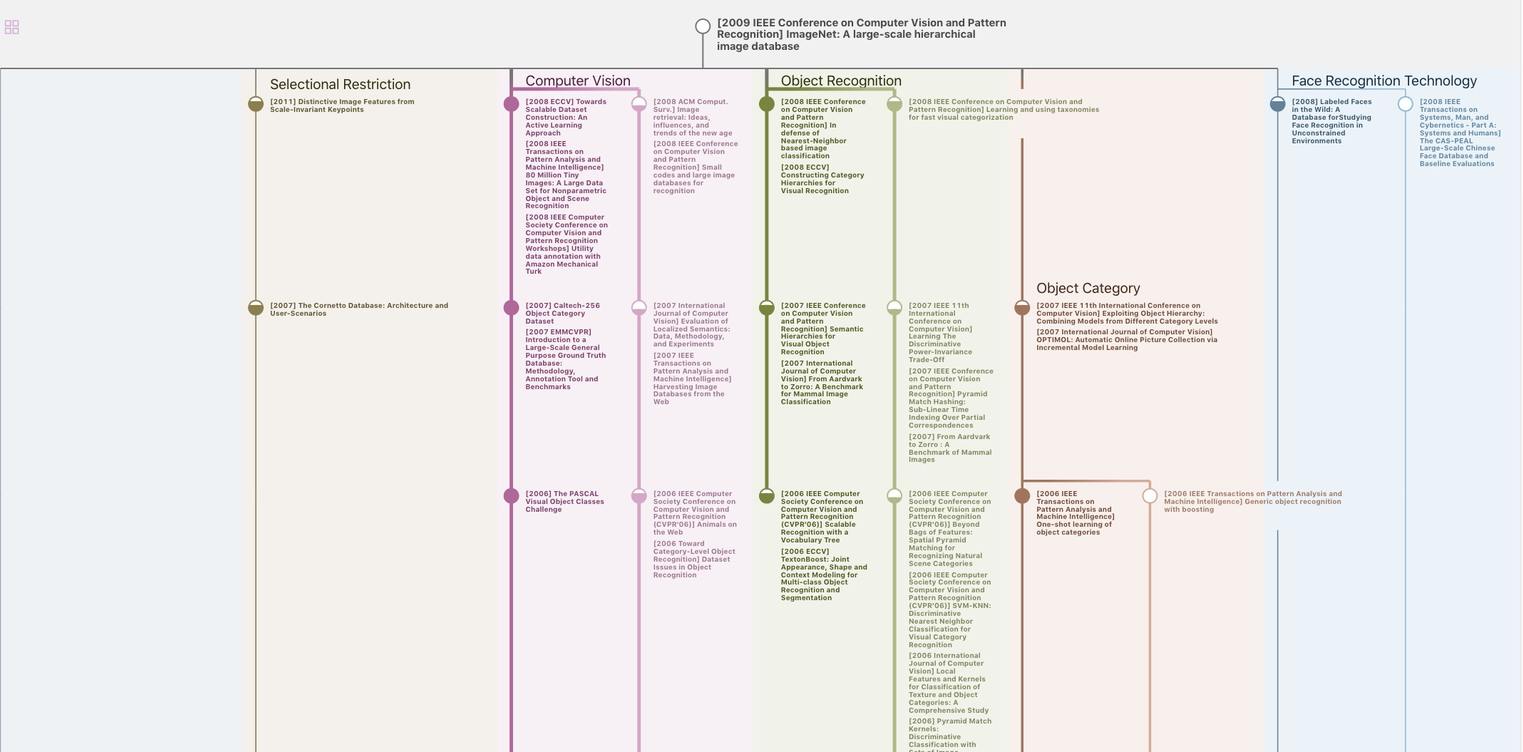
生成溯源树,研究论文发展脉络
Chat Paper
正在生成论文摘要