Sequential Prediction For Imbalanced Data Stream Via Weighted Os-Elm And Dynamic Gan
INTELLIGENT DATA ANALYSIS(2019)
摘要
This paper focuses on the problem of sequential prediction for imbalanced data streams. A novel hybrid algorithm called Weighted OS-ELM and Dynamic Generative Adversarial Nets (GAN-WOSELM) is presented for handling this issue. In the data reconstruction stage, GAN is utilised for generating lifelike minority class samples to equilibrate the data distribution. Then a principal component score threshold helps judge unusual data. In the model update stage, the new constructive ELM is applied to forecast time-varying data chunk. After concerning fitting accuracy and data change, the analytical relationship between the new weight and the shifting imbalance ratio is determined. Therefore, the GAN-WOSELM can update weight quantificationally, and it avoids interactive parameter optimization. According to the suitable weight for the arriving chunk, the proposed method is able to perceive the changeable data distribution and do the adaptation by itself, thus building a reliable model with a low fitting deviation. Numerical experiments are conducted on four different kinds of UCI datasets. The results demonstrate that the proposed algorithm not only has better generalisation performance but also provides higher numerical stability.
更多查看译文
关键词
Sequential prediction, imbalanced data, weighted OS-ELM, dynamic GAN
AI 理解论文
溯源树
样例
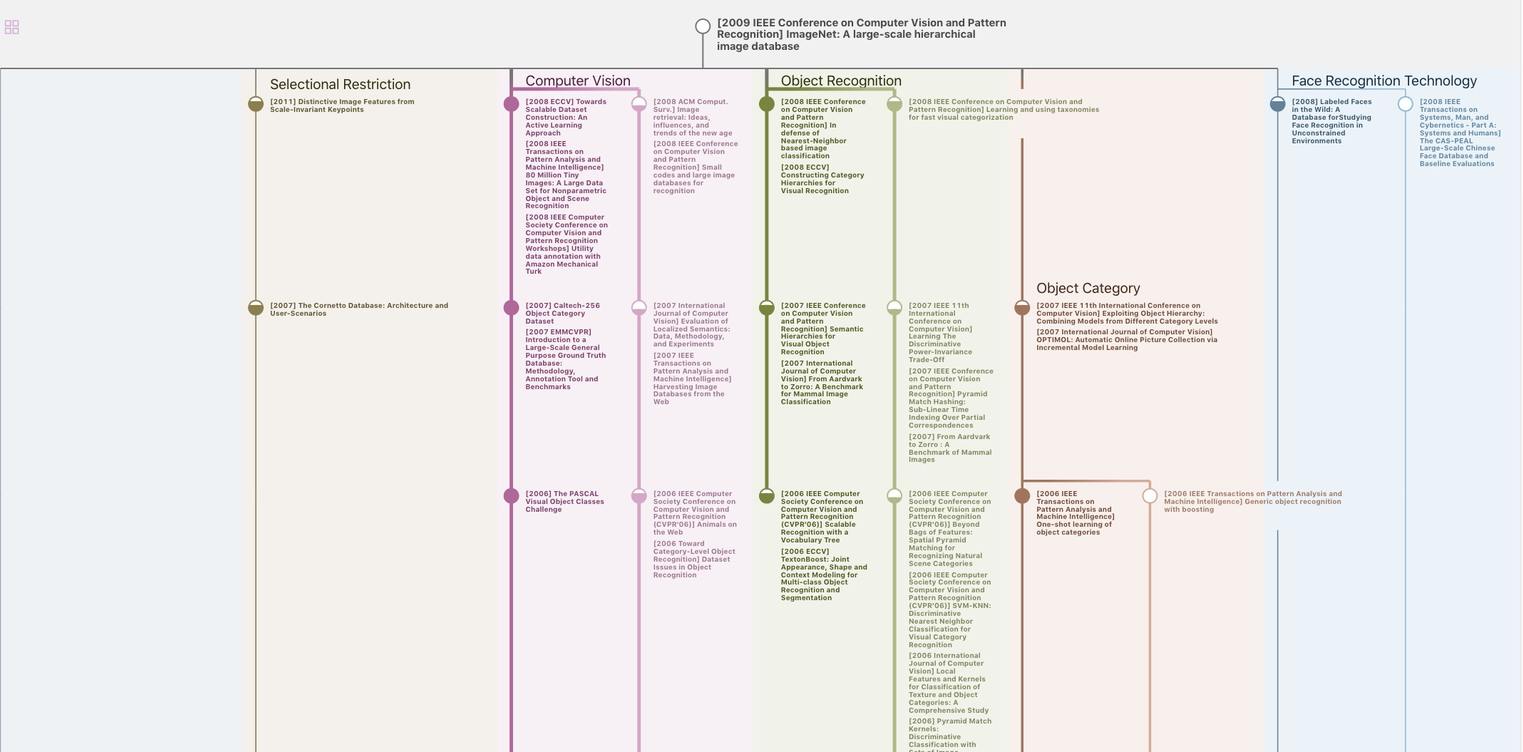
生成溯源树,研究论文发展脉络
Chat Paper
正在生成论文摘要