Deep Regionlets: Blended Representation and Deep Learning for Generic Object Detection.
IEEE TRANSACTIONS ON PATTERN ANALYSIS AND MACHINE INTELLIGENCE(2021)
摘要
In this article, we propose a novel object detection algorithm named "Deep Regionlets" by integrating deep neural networks and a conventional detection schema for accurate generic object detection. Motivated by the effectiveness of regionlets for modeling object deformations and multiple aspect ratios, we incorporate regionlets into an end-to-end trainable deep learning framework. The deep regionlets framework consists of a region selection network and a deep regionlet learning module. Specifically, given a detection bounding box proposal, the region selection network provides guidance on where to select sub-regions from which features can be learned from. An object proposal typically contains three - 16 sub-regions. The regionlet learning module focuses on local feature selection and transformations to alleviate the effects of appearance variations. To this end, we first realize non-rectangular region selection within the detection framework to accommodate variations in object appearance. Moreover, we design a "gating network" within the regionlet leaning module to enable instance dependent soft feature selection and pooling. The Deep Regionlets framework is trained end-to-end without additional efforts. We present ablation studies and extensive experiments on the PASCAL VOC dataset and the Microsoft COCO dataset. The proposed method yields competitive performance over state-of-the-art algorithms, such as RetinaNet and Mask R-CNN, even without additional segmentation labels.
更多查看译文
关键词
Feature extraction,Detectors,Object detection,Proposals,Machine learning,Deformable models,Strain,Object detection,deep learning,deep regionlets,spatial transformation
AI 理解论文
溯源树
样例
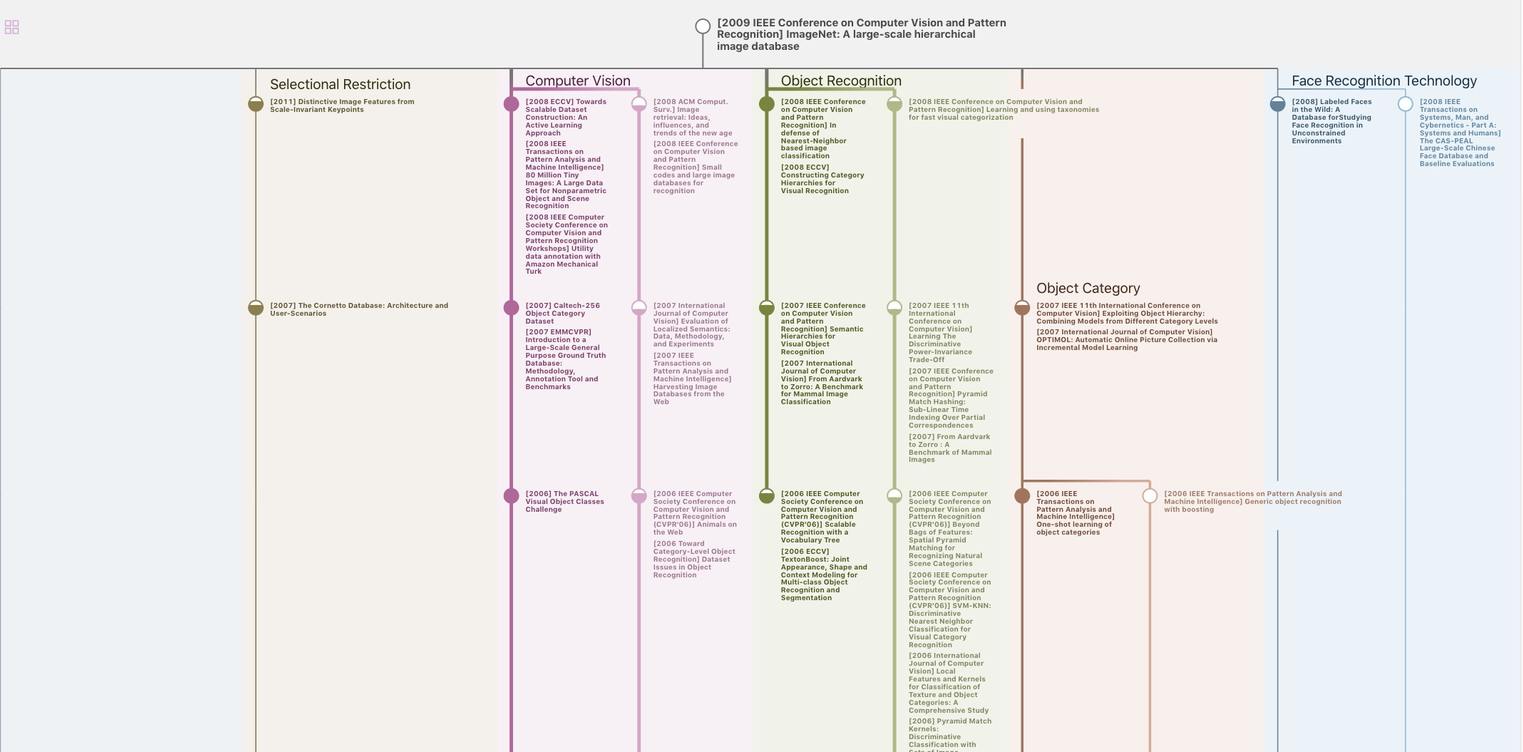
生成溯源树,研究论文发展脉络
Chat Paper
正在生成论文摘要