Improving model drift for robust object tracking
MULTIMEDIA TOOLS AND APPLICATIONS(2020)
Abstract
Discriminative correlation filters show excellent performance in object tracking. However, in complex scenes, the apparent characteristics of the tracked target are variable, which makes it easy to pollute the model and cause the model drift. In this paper, considering that the secondary peak has a greater impact on the model update, we propose a method for detecting the primary and secondary peaks of the response map. Secondly, a novel confidence function which uses the adaptive update discriminant mechanism is proposed, which yield good robustness. Thirdly, we propose a robust tracker with correlation filters, which uses hand-crafted features and can improve model drift in complex scenes. Finally, in order to cope with the current trackers’ multi-feature response merge, we propose a simple exponential adaptive merge approach. Extensive experiments are performed on OTB2013, OTB100 and TC128 datasets. Our approach performs superiorly against several state-of-the-art trackers while runs in real-time.
MoreTranslated text
Key words
Object tracking,Correlation filters,Primary and secondary peaks detection,Confidence function,Adaptive discriminant,Adaptive merge
AI Read Science
Must-Reading Tree
Example
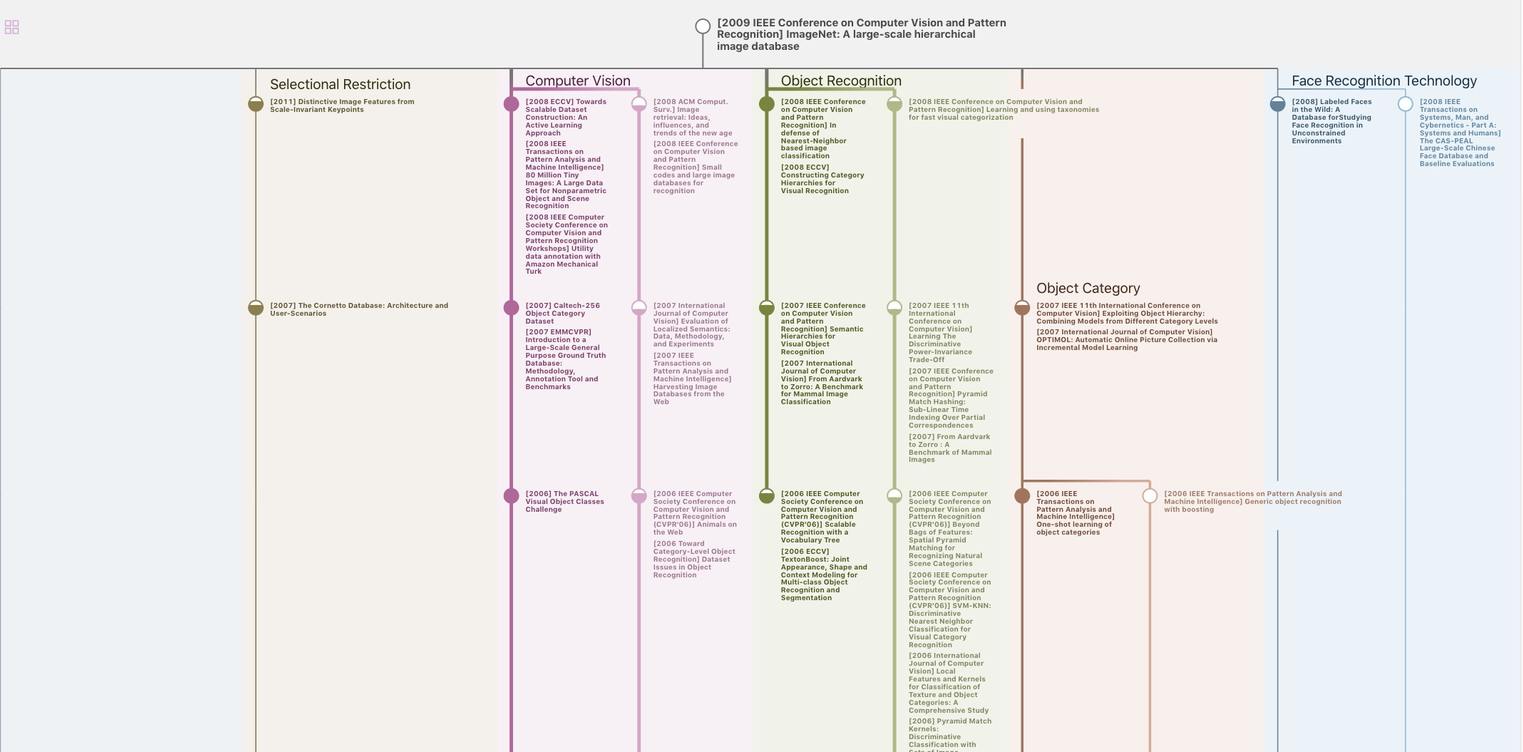
Generate MRT to find the research sequence of this paper
Chat Paper
Summary is being generated by the instructions you defined