Domain-Invariant Stereo Matching Networks
European Conference on Computer Vision(2020)
摘要
State-of-the-art stereo matching networks have difficulties in generalizing to new unseen environments due to significant domain differences, such as color, illumination, contrast, and texture. In this paper, we aim at designing a domain-invariant stereo matching network (DSMNet) that generalizes well to unseen scenes. To achieve this goal, we propose i) a novel “domain normalization” approach that regularizes the distribution of learned representations to allow them to be invariant to domain differences, and ii) an end-to-end trainable structure-preserving graph-based filter for extracting robust structural and geometric representations that can further enhance domain-invariant generalizations. When trained on synthetic data and generalized to real test sets, our model performs significantly better than all state-of-the-art models. It even outperforms some deep neural network models (e.g. MC-CNN[61]) fine-tuned with test-domain data. The code is available at https://github.com/feihuzhang/DSMNet.
更多查看译文
关键词
networks,domain-invariant
AI 理解论文
溯源树
样例
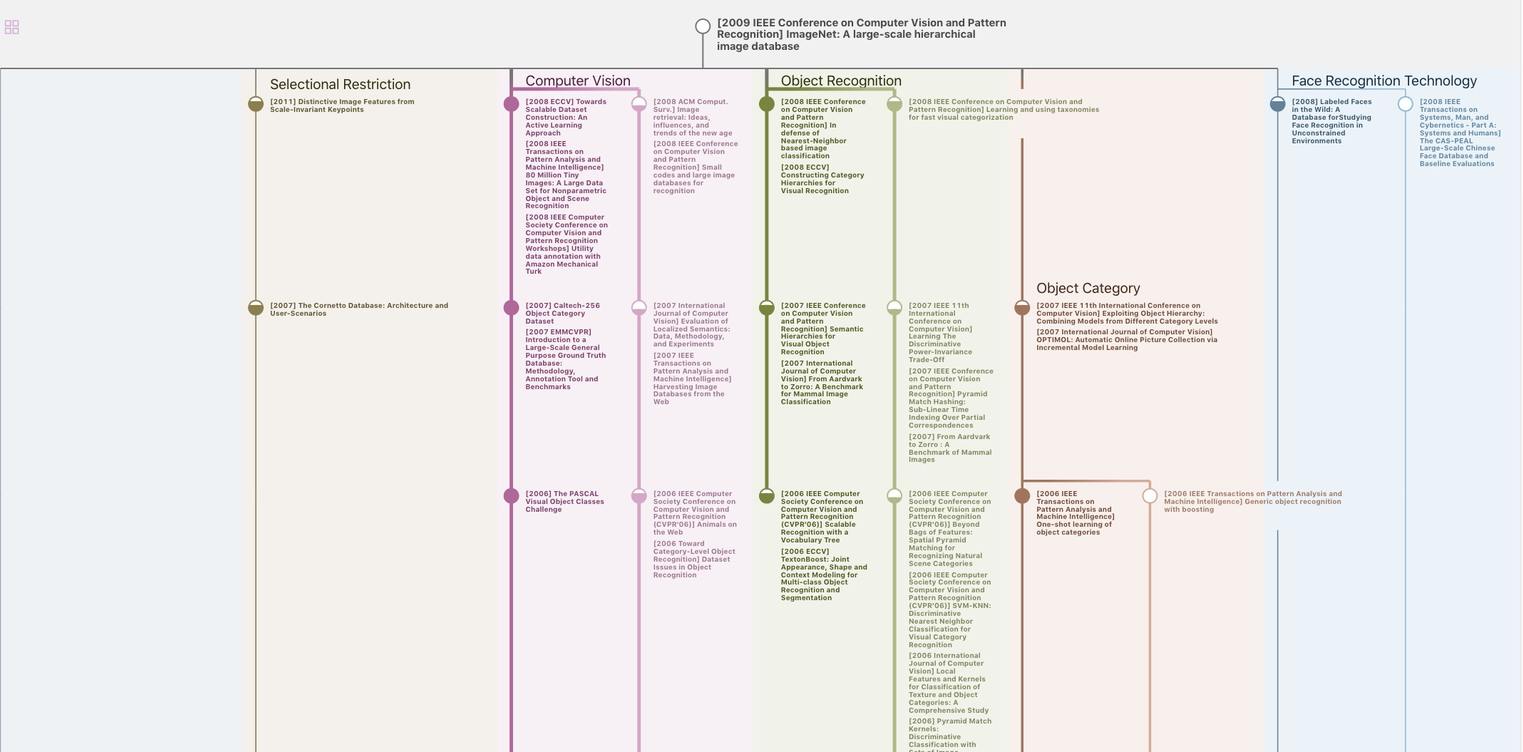
生成溯源树,研究论文发展脉络
Chat Paper
正在生成论文摘要