Novel hybrid approaches based on evolutionary strategy for streamflow forecasting in the Chellif River, Algeria
Acta Geophysica(2019)
摘要
In this study, the feedforward neural networks (FFNNs) were proposed to forecast the multi-day-ahead streamflow. The parameters of FFNNs model were optimized utilizing genetic algorithm (GA). Moreover, discrete wavelet transform was utilized to enhance the accuracy of FFNNs model’s forecasting. Therefore, the wavelet-based feedforward neural networks (WFFNNs-GA) model was developed for the multi-day-ahead streamflow forecasting based on three evolutionary strategies [i.e., multi-input multi-output (MIMO), multi-input single-output (MISO), and multi-input several multi-output (MISMO)]. In addition, the developed models were evaluated utilizing five different statistical indices including root mean squared error, signal-to-noise ratio, correlation coefficient, Nash–Sutcliffe efficiency, and peak flow criteria. Results provided that the statistical values of WFFNNs-GA model based on MISMO evolutionary strategy were superior to those of WFFNNs-GA model based on MISO and MIMO evolutionary strategies for the multi-day-ahead streamflow forecasting. Results indicated that the performance of WFFNNs-GA model based on MISMO evolutionary strategy provided the best accuracy. Results also explained that the hybrid model suggested better performance compared with stand-alone model based on the corresponding evolutionary strategies. Therefore, the hybrid model can be an efficient and robust implement to forecast the multi-day-ahead streamflow in the Chellif River, Algeria.
更多查看译文
关键词
Daily streamflow forecasting, Wavelet transform, Feed forward neural networks, Multi-input multi-output, Multi-input single-output, Multi-input several multi-output, Chellif River
AI 理解论文
溯源树
样例
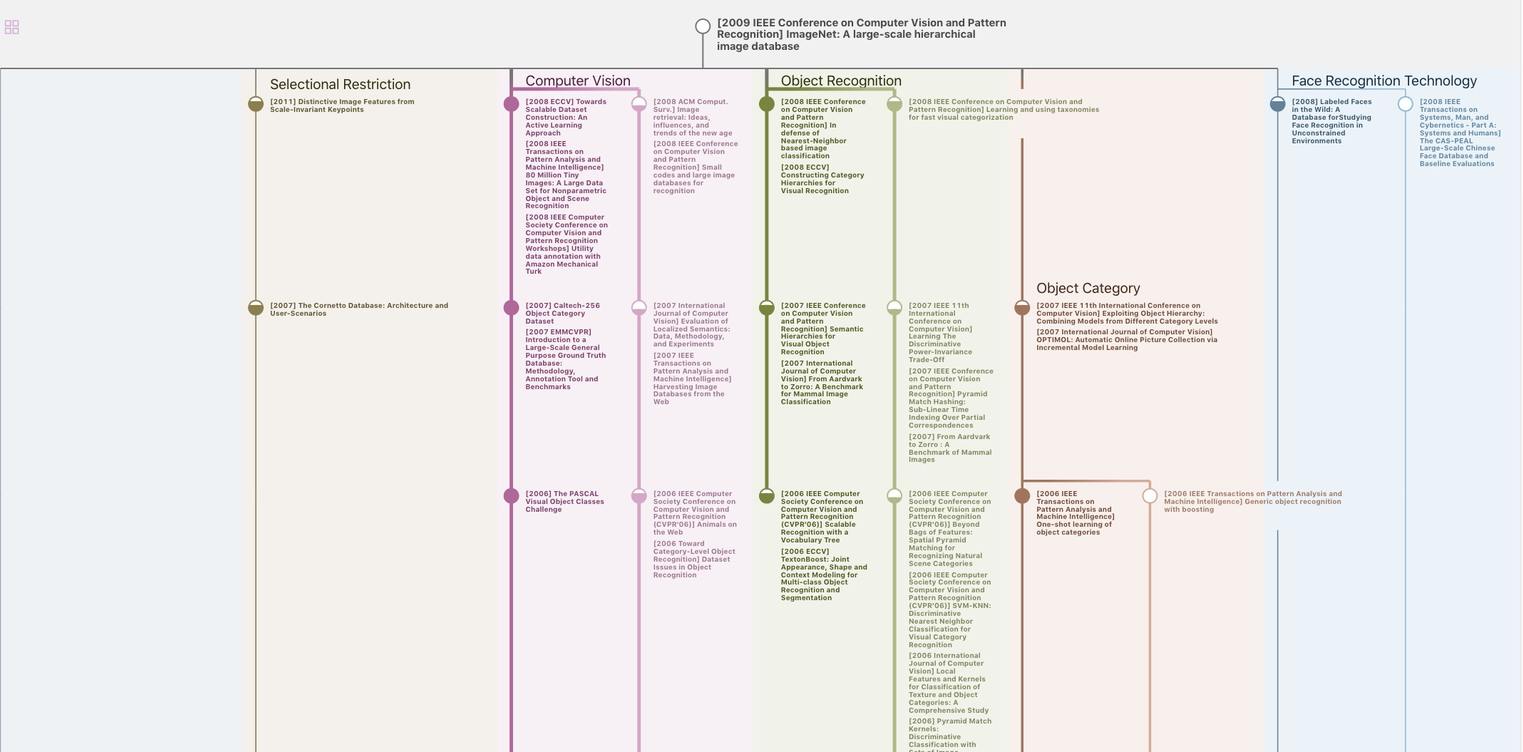
生成溯源树,研究论文发展脉络
Chat Paper
正在生成论文摘要