An efficient active learning method for multi-task learning
Knowledge-Based Systems(2020)
摘要
In multi-task learning, the sharing of information between related tasks affects and promotes the learning of each task. However, the traditional multi-task learning techniques always require sufficient labeled data to improve the learning of each task, and labeling samples is always expensive in practice. In this paper, we propose two variants of active learning methods for multi-task classification. In the uncertainty step, we propose the support vector preservation criterion that evaluates uncertainty at the level of classifier, which is called classifier-level uncertainty (CLU). In the diversity step, we propose two diversity criteria that evaluate diversity by the clustering method and the partition method respectively, which are called clustering-based diversity (CBD) and partition-based diversity (PBD) respectively. Each diversity criterion together with the uncertainty criterion is to form an active learning method for multi-task learning. In addition, the proposed support vector preservation criterion selects local informative samples which determine the hyperplane for each task. Furthermore, in order to maintain the distribution structure of the samples, we put forward the micro-kernel k-means clustering method and partition-based method to select global informative samples from the non-support vectors. By incorporating the local and global informative samples into active learning, we propose the two active learning methods for multi-task problems. We evaluate the effectiveness of the proposed methods by conducting experiments with other active learning methods. The experimental results show that the proposed two methods perform better than other active learning methods.
更多查看译文
关键词
Multi-task classification,Active learning,Support vector machine
AI 理解论文
溯源树
样例
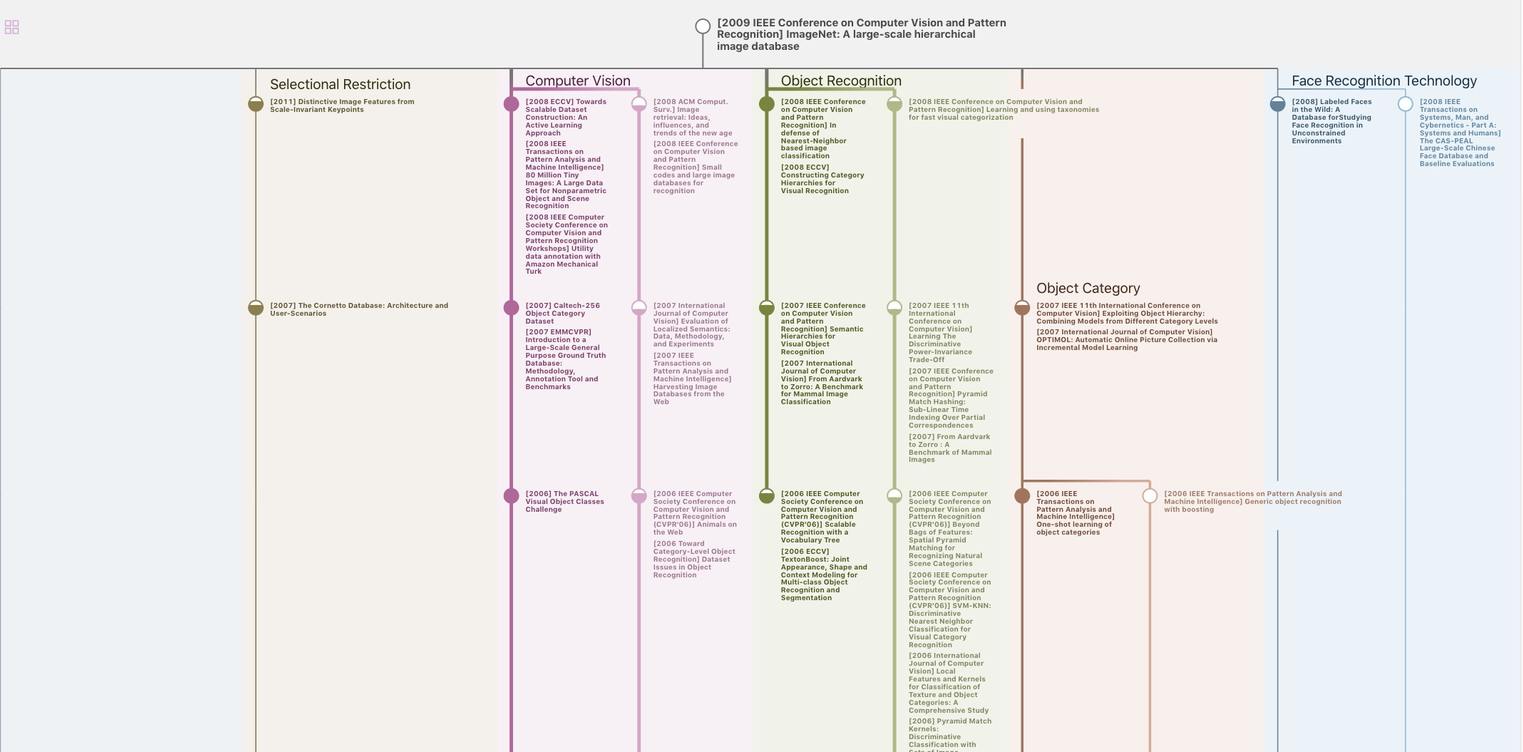
生成溯源树,研究论文发展脉络
Chat Paper
正在生成论文摘要