eXITs - An Ensemble Approach for Imputing Missing EHR Data.
ICHI(2019)
摘要
Missing data points are prevalent in electronic health records (EHRs) and are an impedance to utilizing machine learning for predictive and classification tasks in healthcare. For this challenge, we developed eXITs - a stacked ensemble learner that employs 6 base models to perform imputation on time series data from 13 different laboratory tests across 8, 267 patients in the MIMIC-III database provided in the ICHI 2019 Data Analytics Challenge on Missing Data Imputation (DACMI). The results show that our ensemble model (avg. nRMSE = 0.200) outperforms the reference model, 3D-MICE (avg. nRMSE = 0.222) by 9.69%.
更多查看译文
关键词
data points,electronic health records,predictive tasks,classification tasks,stacked ensemble learner,base models,time series data,MIMIC-III database,ICHI 2019 Data Analytics Challenge,ensemble approach,eXIT,imputed missing EHR data
AI 理解论文
溯源树
样例
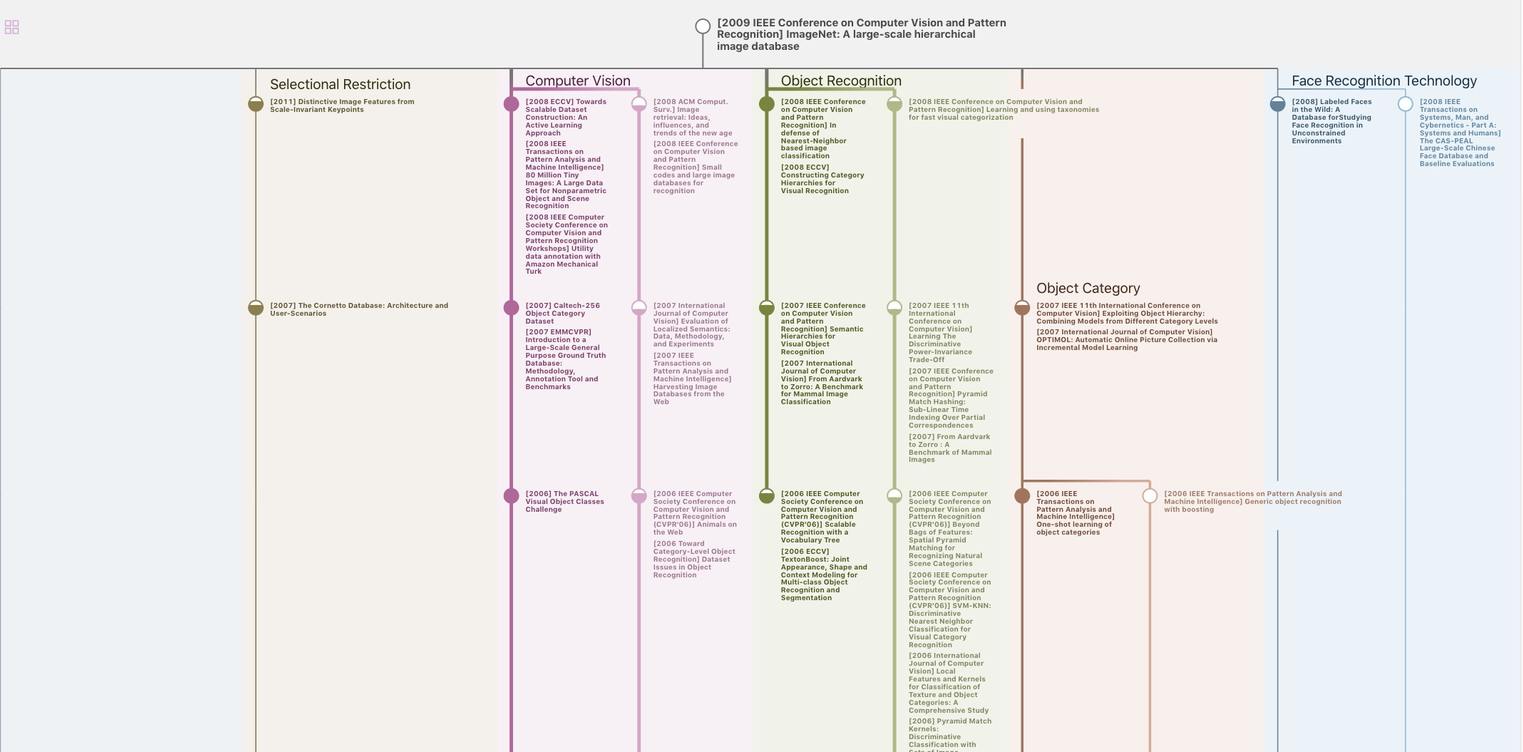
生成溯源树,研究论文发展脉络
Chat Paper
正在生成论文摘要