Recognizing Novel Tumor Suppressor Genes Using A Network Machine Learning Strategy
IEEE ACCESS(2019)
摘要
Extensive research on tumor suppressor genes (TSGs) is helpful to understand the pathogenesis of cancer and design effective treatments. However, using traditional experiments to identify TSGs is of high costs and time-consuming. It is an alternative way to design effective computational methods for screening out latent TSGs. Up to now, some computational methods have been proposed to predict new TSGs. However, these methods did not contain a learning procedure to extract essential properties of validated TSGs, reducing their efficiencies. In this study, a novel computational method was proposed to identify latent TSGs. To this end, we downloaded the validated TSGs from the TSGene database (Version 1.0). These TSGs together with other genes were represented by features that were extracted from protein-protein interaction networks in STRING via a powerful network embedding method, Mashup. Then, thirty random forest models were constructed and used to predict latent TSGs. 135 inferred TSGs were obtained, where 28 genes have been included in the TSGene database (Version 2.0). Our method had better performance than some previous methods according to the validated TSGs in the TSGene database (Version 2.0). For the rest 107 inferred TSGs, some of them can be confirmed to be TSGs with solid literature support. Finally, our method can overcome the defects that only genes with strong associations to validated TSGs can be identified because we obtained several inferred TSGs that had weak associations to validated TSGs and can be novel TSGs with high probabilities.
更多查看译文
关键词
Tumor suppressor gene,network embedding method,mashup,machine learning,random forest
AI 理解论文
溯源树
样例
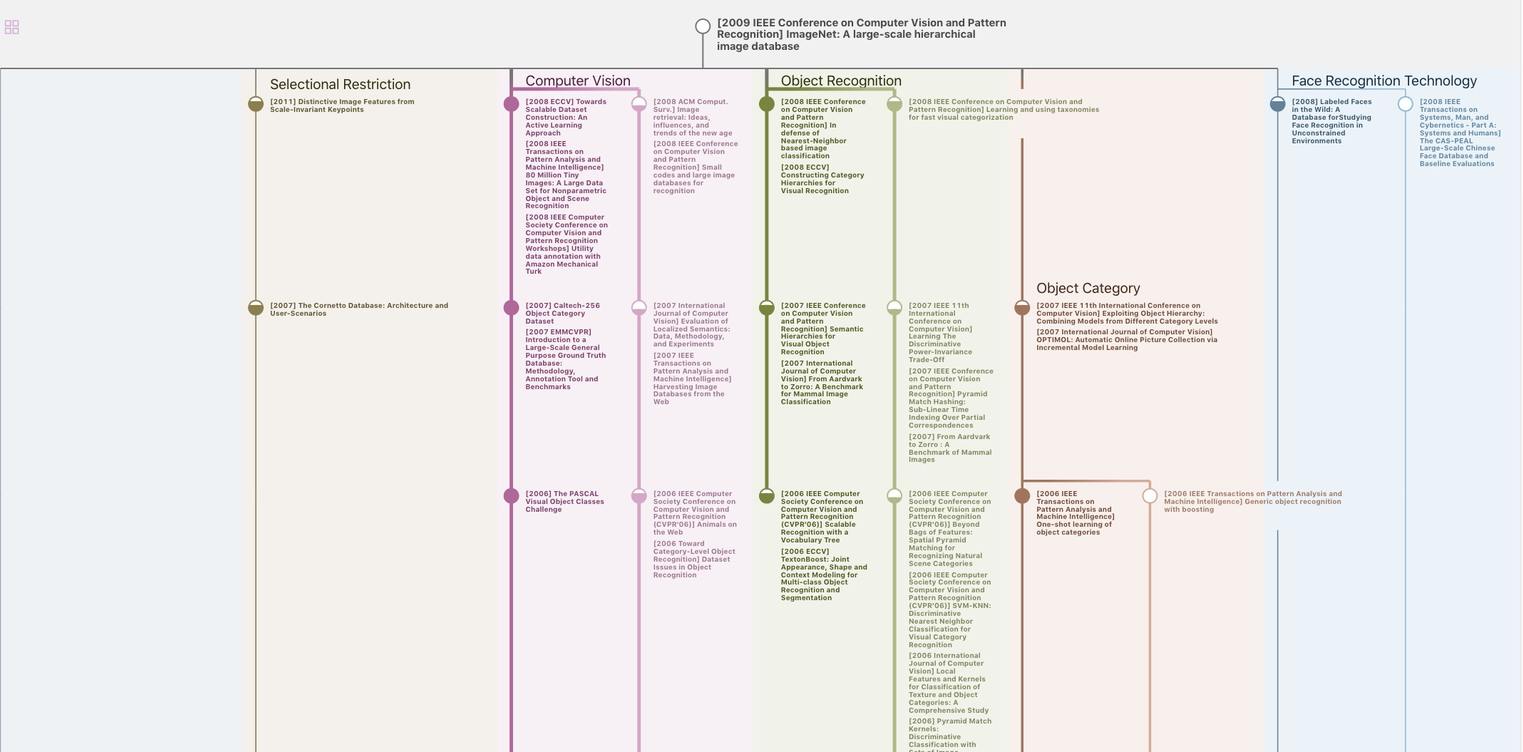
生成溯源树,研究论文发展脉络
Chat Paper
正在生成论文摘要