Deep machine learning for detection of acoustic wave reflections
STRUCTURAL HEALTH MONITORING-AN INTERNATIONAL JOURNAL(2020)
摘要
Acoustic emission signals are information rich and can be used to estimate the size and location of damage in structures. However, many existing algorithms may be deceived by indirectly propagated acoustic emission waves which are modulated by reflection boundaries within the structures. We propose two deep learning models to identify such waves such that existing algorithms for damage detection and localization may be used. The first approach uses long short-term memory recurrent neural networks to learn distinct patterns directly from the time-series data. In the second approach, we transform the time-series data into spectrograms and utilize convolutional neural networks to perform binary classification by leveraging spectro-temporal features. We achieved 80% classification accuracy using long short-term memory and near-perfect accuracy using convolutional neural networks on a dataset of acoustic emission signals generated by the Hsu-Nielsen sources. Both long short-term memory and convolutional neural network models were able to learn general and context-specific features of the direct and reflected acoustic emission waves. Once accurately identified, the indirectly propagating waves are filtered out while the directly propagating waves are used for source location using existing methods.
更多查看译文
关键词
Long short-term memory,recurrent neural network,acoustic emissions,convolutional neural network,structural health monitoring
AI 理解论文
溯源树
样例
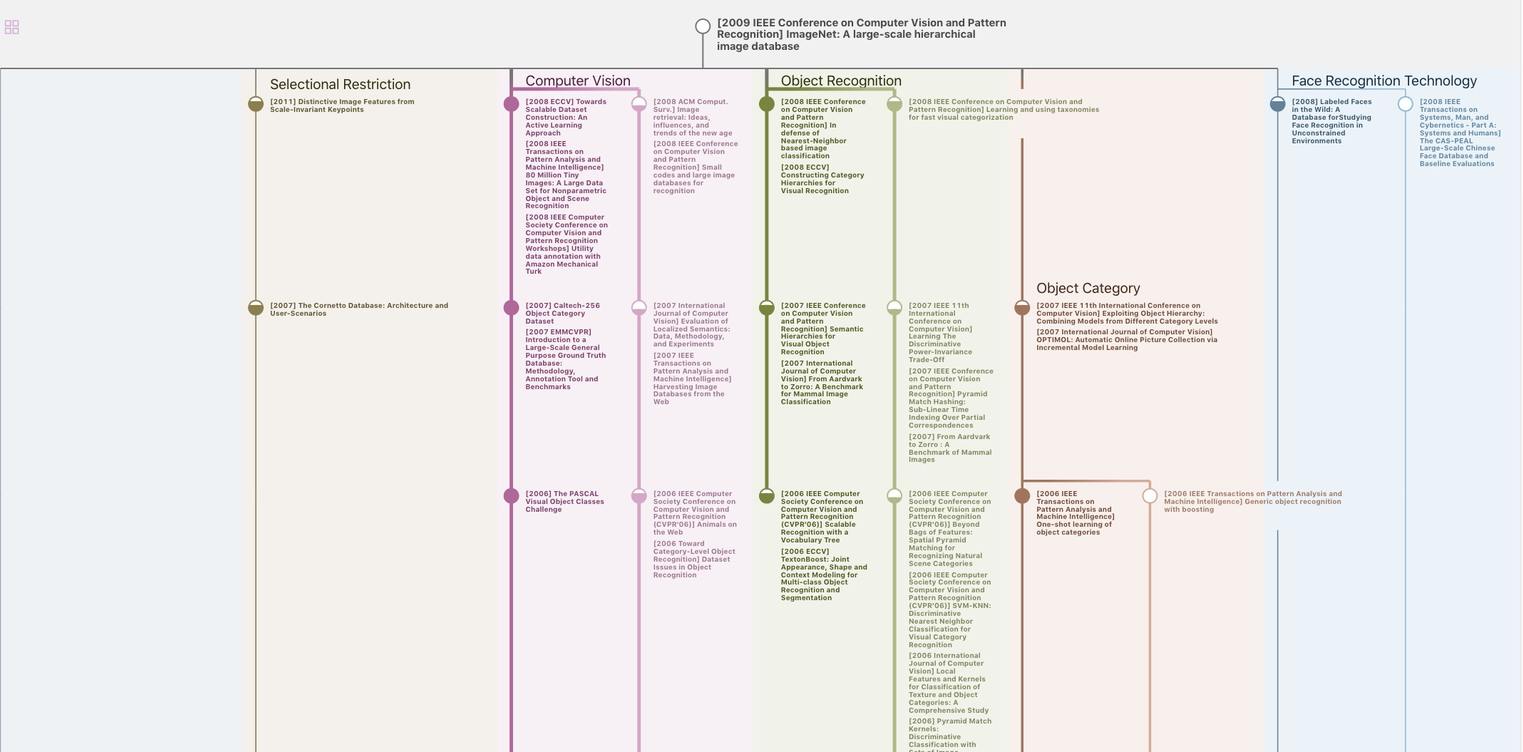
生成溯源树,研究论文发展脉络
Chat Paper
正在生成论文摘要