Infinite Gaussian Fisher Vector To Support Video-Based Human Action Recognition
ADVANCES IN VISUAL COMPUTING, ISVC 2019, PT II(2019)
Abstract
Human Action Recognition (HAR) is a computer vision task that attempts to monitor, understand, and characterize humans in videos. Here, we introduce an extension to the conventional Fisher Vector encoding technique to support this task. The methodology, based on the Infinite Gaussian Mixture Model (IGMM) seeks to reveal a set of discriminant local spatio-temporal features for enabling the precise codification of visual information. Specifically, it is much simpler to handle the infinite limit from the IGMM, than working with traditional Gaussian Mixture Models (GMMs) with unknown sizes, that will require extensive cross-validation. Under this premise, we developed a fully automatic encoding methodology that avoids heuristically specifying the number of components in the mixture model. This parameter is known to greatly affect the recognition performance, and its inference with conventional methods implies a high computational burden. Moreover, the Markov Chain Monte Carlo implementation of the hierarchical IGMM effectively avoids local minima, which tend to plague mixtures trained by optimization-based methods. Attained results on the UCF50 and HMDB51 databases demonstrate that our proposal outperforms state of the art encoding approaches concerning the trade-off between recognition performance and computational complexity, as it drastically reduces both number of operations and memory requirements.
MoreTranslated text
Key words
Human Action Recognition, Infinite Gaussian Mixture Model, Fisher Vector, Video processing
AI Read Science
Must-Reading Tree
Example
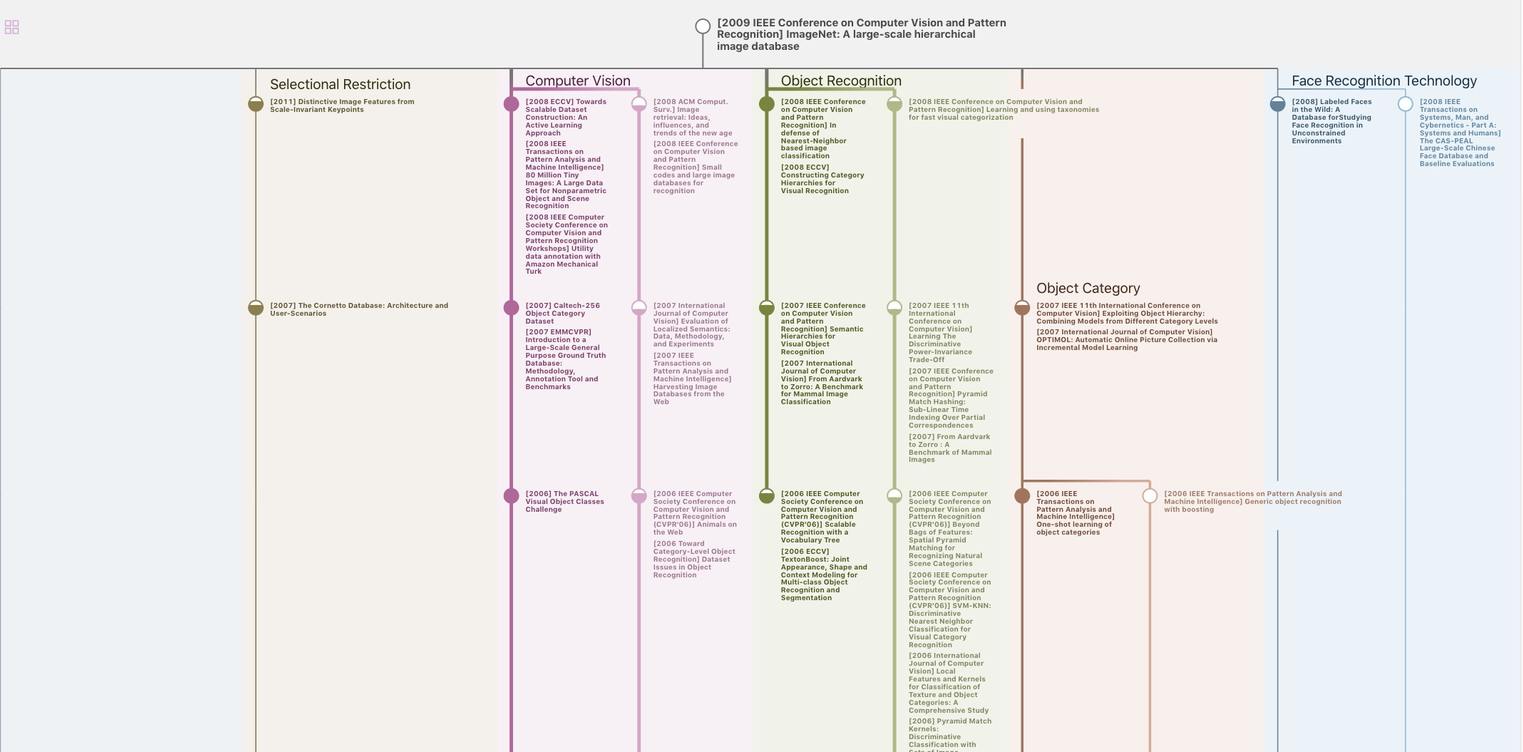
Generate MRT to find the research sequence of this paper
Chat Paper
Summary is being generated by the instructions you defined