SCRSR: An Efficient Recursive Convolutional Neural Network for Fast and Accurate Image Super-Resolution
Neurocomputing(2020)
Abstract
Convolutional neural networks have recently demonstrated high-quality reconstruction for single image super-resolution (SR). These CNN networks effectively recover a high-resolution (HR) image from a low-resolution (LR) image, at the cost of enormous parameters and heavy computational burden. In this work, we propose a recursive efficient deep convolutional network for fast and accurate single-image SR with only 0.28M parameters. A Split-Concatenate-Residual (SCR) block is proposed to reduce computation and parameters. With downsampling block and upsampling block, we significantly reduce computational complexity and enlarge the size of the receptive field. Specifically, two-level recursive learning is proposed which can improve accuracy by increasing depth without adding any weight parameters. We also employ local, semi-global and global residual techniques to train our very deep network steadily and improve its performance. Extensive experiments indicate that our proposed method Split-Concatenate-Residual Super Resolution (SCRSR) yields promising SR performance while maintaining shorter running time and fewer parameters.
MoreTranslated text
Key words
Super-resolution,Recursive convolutional neural networks,Efficient model
AI Read Science
Must-Reading Tree
Example
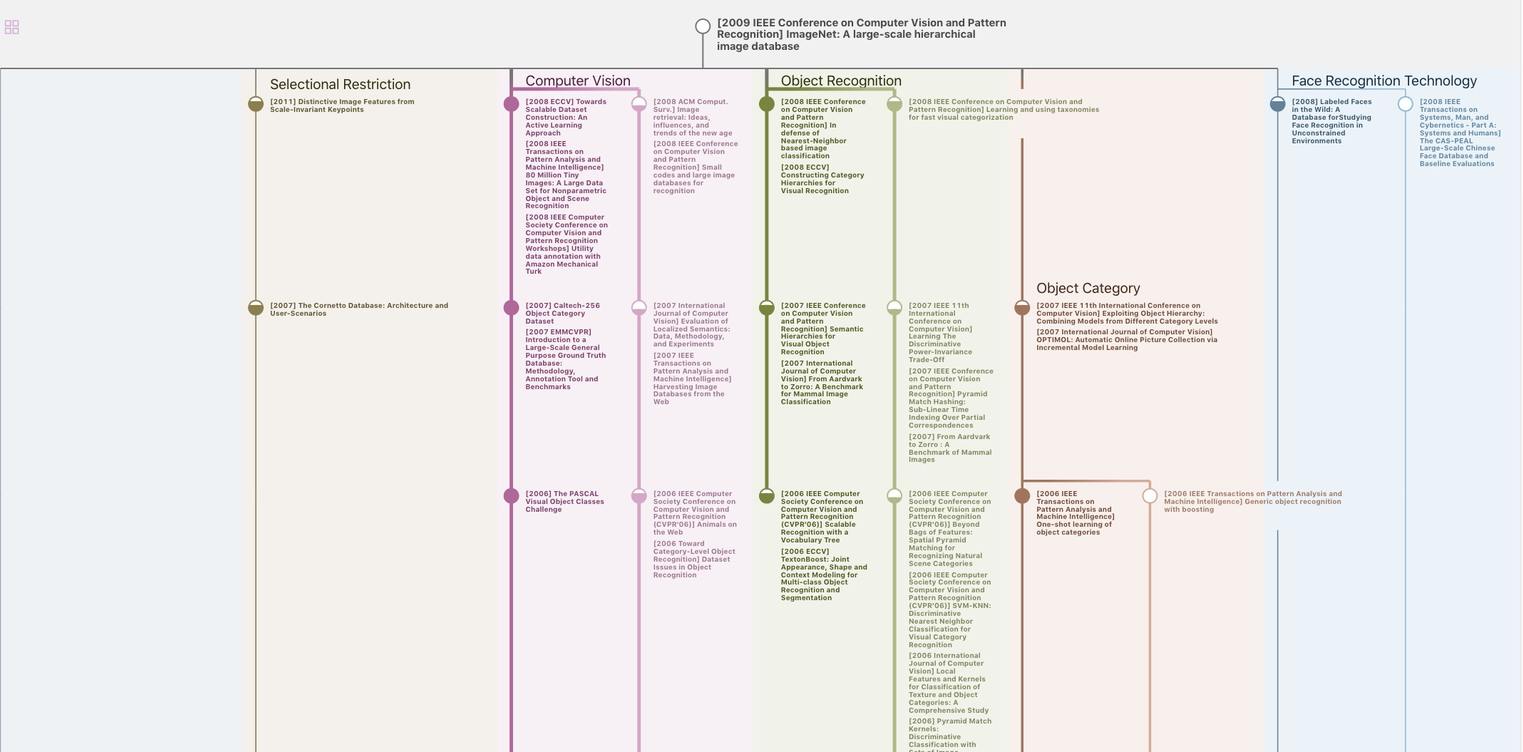
Generate MRT to find the research sequence of this paper
Chat Paper
Summary is being generated by the instructions you defined