Adaptive Rank Estimation Based Tensor Factorization Algorithm For Low-Rank Tensor Completion
PROCEEDINGS OF THE 38TH CHINESE CONTROL CONFERENCE (CCC)(2019)
Abstract
As the generalized form of vectors and matrices, tensors can describe the data structures more directly. In the process of acquiring higher-order tensor, some entries may be lost due to various reasons. Low-rank tensor completion (LRTC) is to recover the missing entries according to the low-rank property of tensor. The tensor completion by tensor factorization (TCTF) method was proposed to solve the LRTC problem. However, tubal rank of the original tensor is assumed known in TCTF method. In this paper, we propose an adaptive rank estimation based tensor factorization (ARE-TF) algorithm for low-rank tensor completion. Based on L1-norm regularized rank-one matrix completion algorithm, the estimated rank is obtained by iterative updating procedures. The experiment results in image completion show that the proposed algorithm has better performance than the existing algorithms.
MoreTranslated text
Key words
Low-rank tensor completion, tubal rank, adaptive rank estimation, image completion
AI Read Science
Must-Reading Tree
Example
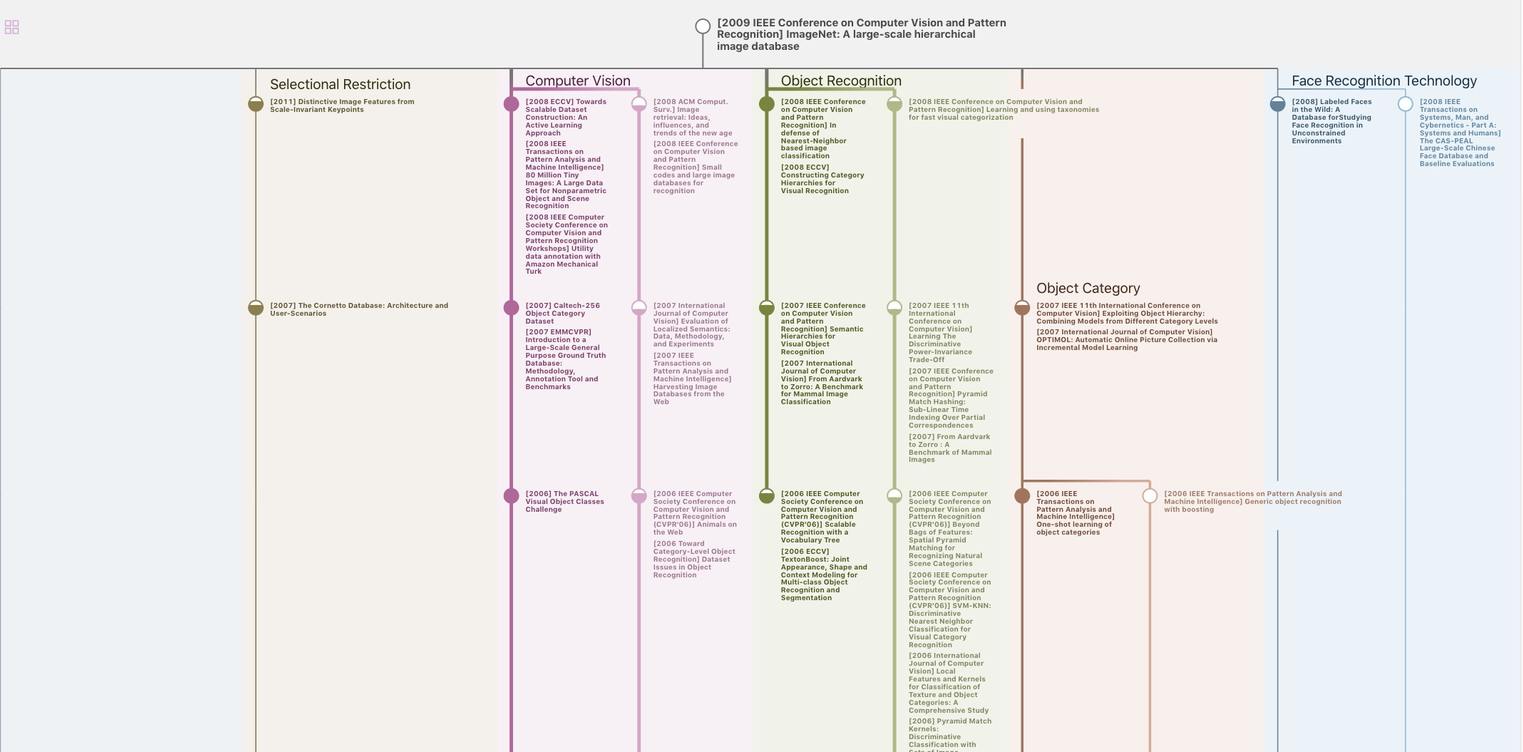
Generate MRT to find the research sequence of this paper
Chat Paper
Summary is being generated by the instructions you defined