P3-366: support vector machine analysis of resting-state fmri in cognitive continuum
Alzheimers & Dementia(2019)
摘要
Individuals with Subjective Cognitive Decline (SCD) and amnestic mild cognitive impairment (aMCI) are associated with Alzheimer's disease (AD). However, the heterogeneity of them may limit the clinical applications in dementia researches. Pattern recognition models have been increasingly applied to neuroimaging data over the past few decades. In this study, we used support vector machine (SVM) as a pattern classification technique to employ the features of all groups in cognitive continuum in AD. 334 subjects with functional MRI data and cognitive data were available, including 101 subjects with normal cognition (NC), 66 with SCD, 102 with aMCI and 65 with dementia. Subjects with SCD complained their memory although they were cognitively normal based on neuropsychological tests. We constructed a multidimensional pattern with varieties of brain areas by SVM. More specifically, the multiple kernel learning model of SVM simultaneously learn the contribution of each brain region, previously defined by an atlas, to the classification. Various resting-state fMRI measures, including fractional amplitude of low frequency fluctuations, regional homogeneity and network centrality were used to construct features. The original accuracy of the discrimination between patients with dementia, aMCI, SCD and subjects with NC was 83%, 62%,56%, respectively, after cross-validation. Depending on various features selection and stages, the accuracy of pattern recognition varied much. Among the three measures, classification with features of fractional amplitude of low frequency fluctuations could achieve the best performance. Further, the concordance of key regions for discrimination among indices of brain function was largest in dementia group and smallest in SCD group. Several brain regions showed significant differences among four groups, including Parietal_Sup_L (aal), Frontal_Inf_Oper_L (aal) and Caudate_R (aal). Importantly, after improving features selection according to the key regions of each group, the accuracy could increase 10%∼20% compared with the baseline. The values of these features were significantly correlated with the cognitive test scores. The results showed the concordance and discordance in intrinsic brain activity with various fMRI measures in various stages of cognition continuum. In addition, these results indicated the potential of SVM to be applied into decreasing the heterogeneity in dementia researches.
更多查看译文
关键词
fmri,support vector machine analysis,cognitive,resting-state
AI 理解论文
溯源树
样例
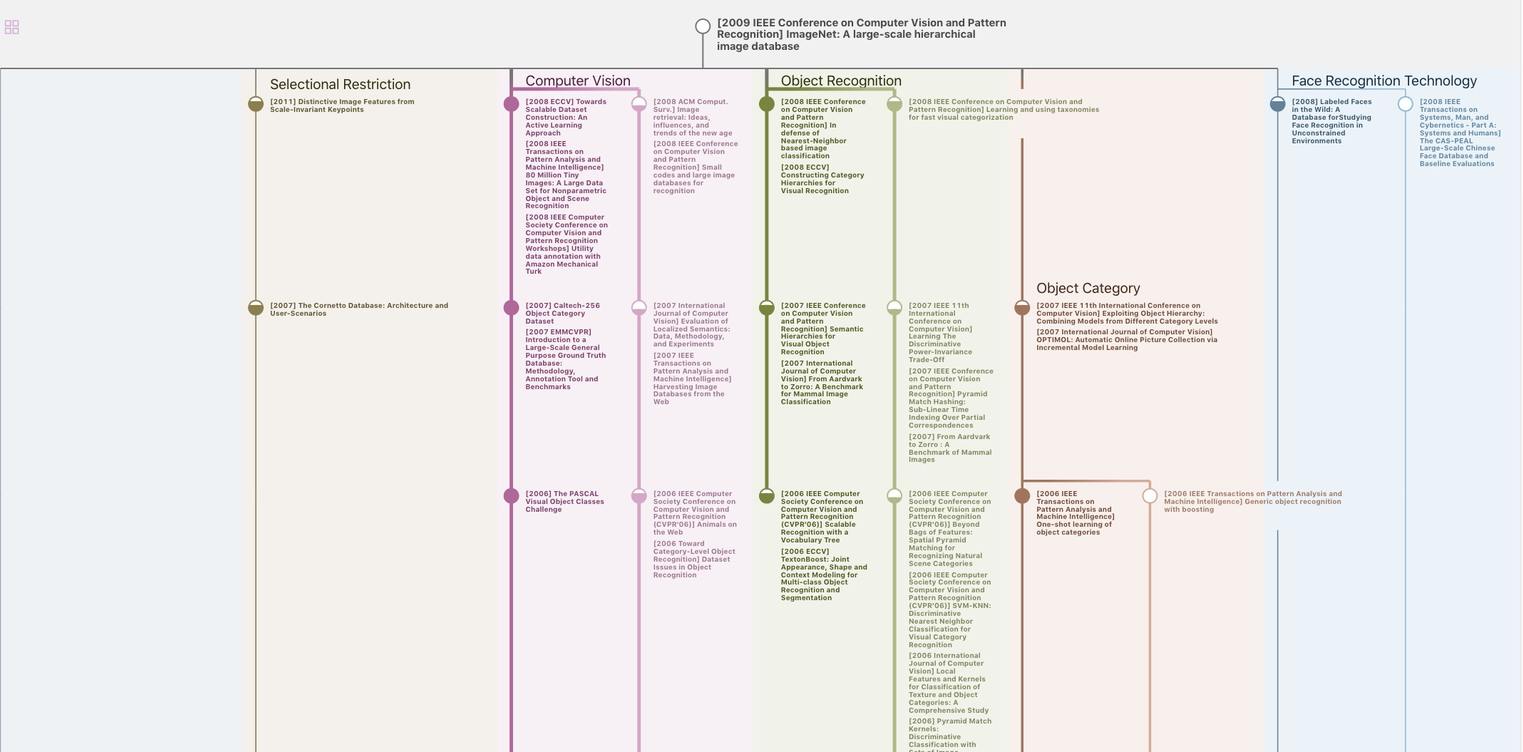
生成溯源树,研究论文发展脉络
Chat Paper
正在生成论文摘要