Aerial Unstructured Road Segmentation Based On Deep Convolution Neural Network
PROCEEDINGS OF THE 38TH CHINESE CONTROL CONFERENCE (CCC)(2019)
摘要
Due to the irregular shape, the blurred edge of the road and the occlusion of obstacles on unstructured roads (rural roads, off-road), some networks that achieve good segmentation effect on structured road images have poor effect on unstructured road images. The segmentation of aerial unstructured roads can obtain information on ground objects and understand the development of the area. The use of deep convolutional neural networks to achieve semantic segmentation of roads has always been a hot research direction. In this paper, it is proposed a semantic segmentation network called RD-Net, which achieves road semantic segmentation. The network includes the reflection padding and the stack of "convolution + pooling" for feature extraction, the dilated residual transition unit to deepen the network and up-sampling for size restore. The proposed network is tested on aerial unstructured road datasets and compared it to other four state of the art deep learning-based road extraction networks. The proposed network performs well on the road segmentation task, and the segmentation accuracy has also improved. This shows that it is effective and available on unstructured road segmentation.
更多查看译文
关键词
Deep convolutional neural network, reflection padding, dilated residual transition unit, unstructured road segmentation
AI 理解论文
溯源树
样例
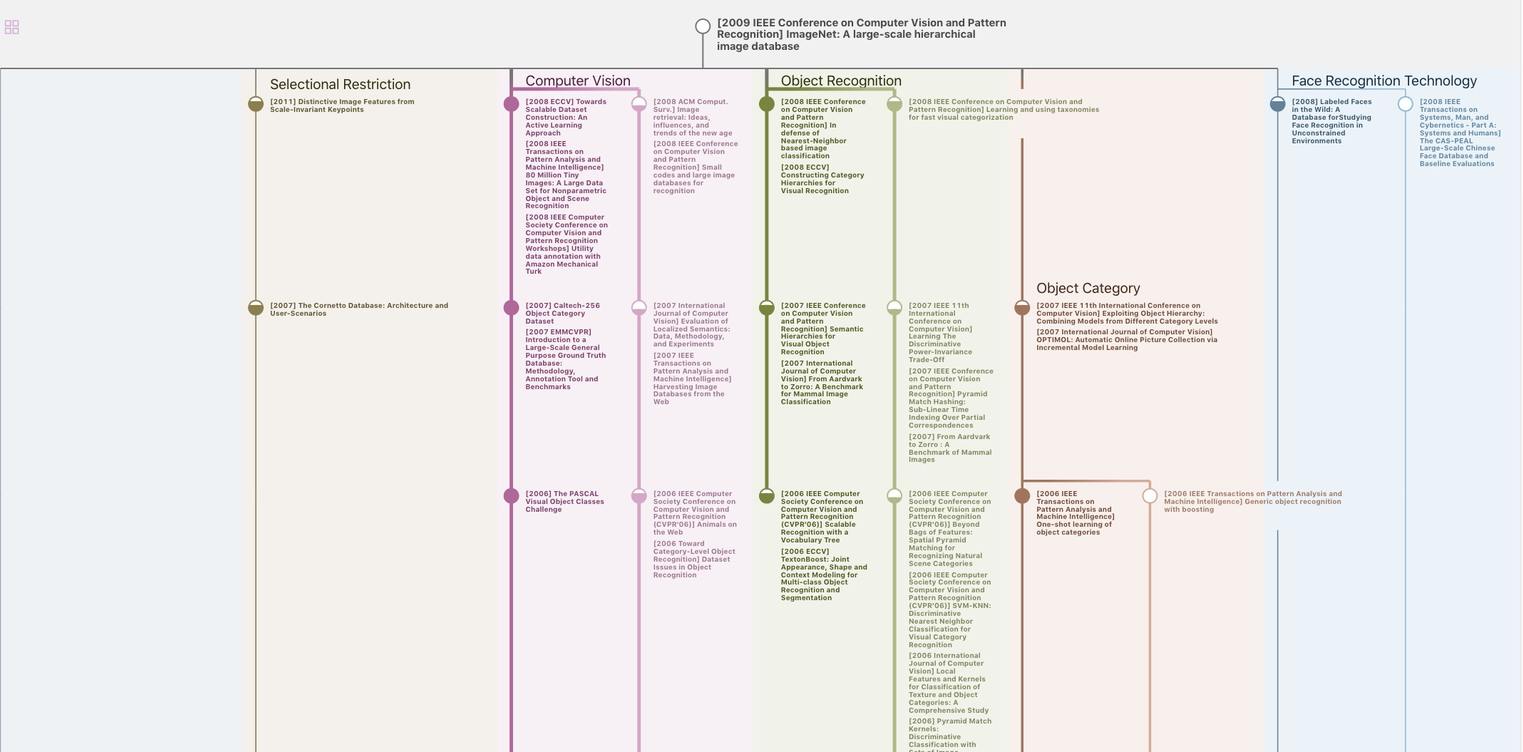
生成溯源树,研究论文发展脉络
Chat Paper
正在生成论文摘要