Fuzzy Ordered c-Means Clustering and Least Angle Regression for Fuzzy Rule-Based Classifier: Study for Imbalanced Data
IEEE Transactions on Fuzzy Systems(2020)
摘要
This article introduces a new classifier designmethod that is based on a modification of the traditional fuzzy clustering. First, a new fuzzy ordered c-means clustering is proposed. This method can be considered as a generalization of the concept of the conditional fuzzy clustering by introducing ordering and weighting distances from data to cluster prototypes. As a result, a more local impact of data on created groups and increased repulsive force between group prototypes are obtained. The proposed method provides a better representation of the data classes, in particular for classes with small cardinality in the training set (imbalanced data). A special initialization of the prototypes is also introduced. Next, the proposed clustering method is used to construct the premises of if-then rules of a fuzzy classifier. The conclusions of the rules are obtained by the least angle regression algorithm, which selects only those rules, that maximize the generalization ability of a classifier. Each if-then rule is represented in easily interpretable Mamdani-Assilian form. Finally, an extensive experimental analysis on 89 benchmark balanced and imbalanced datasets is performed to demonstrate the validity of the introduced classifier. Its competitiveness to state-of-the-art classifiers, with respect to both performance and interpretability, is shown as well.
更多查看译文
关键词
Conditional fuzzy clustering,fuzzy classifier design,imbalanced data,rulebase with good interpretability
AI 理解论文
溯源树
样例
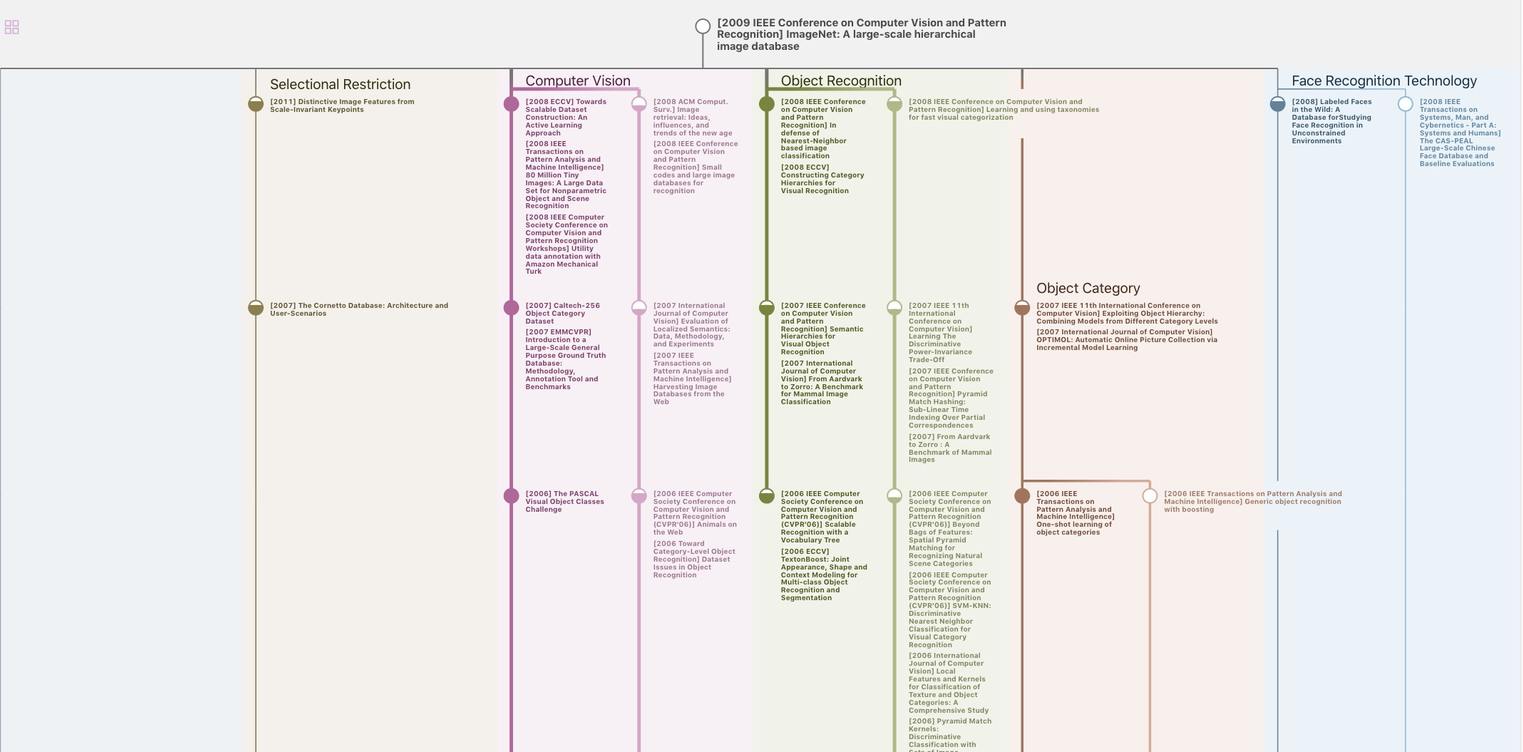
生成溯源树,研究论文发展脉络
Chat Paper
正在生成论文摘要