A multi-ensemble method based on deep auto-encoders for fault diagnosis of rolling bearings
MEASUREMENT(2020)
Abstract
A multi-ensemble method is proposed based on deep auto-encoder (DAE) for fault diagnosis of rolling bearings. At first, several DAEs with different activation functions are trained to obtain different types of features, which are merged into a feature pool. Then, the features in the feature pool are evaluated and selected. The classifiers are constructed for each feature. The classification accuracy is used as evaluation index and good features are selected. Finally, the train data is cross-divided and multi sample-sets with selected features are constructed. Each sample-set is used to train a classifier, the final diagnosis result is obtained by majority voting among these classification results. The proposed method is validated by two experimental bearing vibrations signals and also compared with other methods. The results revealed that different types of features can be obtained by DAEs with different activation functions. The proposed method has a high accuracy and good generalization ability. (C) 2019 Elsevier Ltd. All rights reserved.
MoreTranslated text
Key words
Rolling bearing,Fault diagnosis,Ensemble strategy,Deep auto-encoder,Multi-ensemble
AI Read Science
Must-Reading Tree
Example
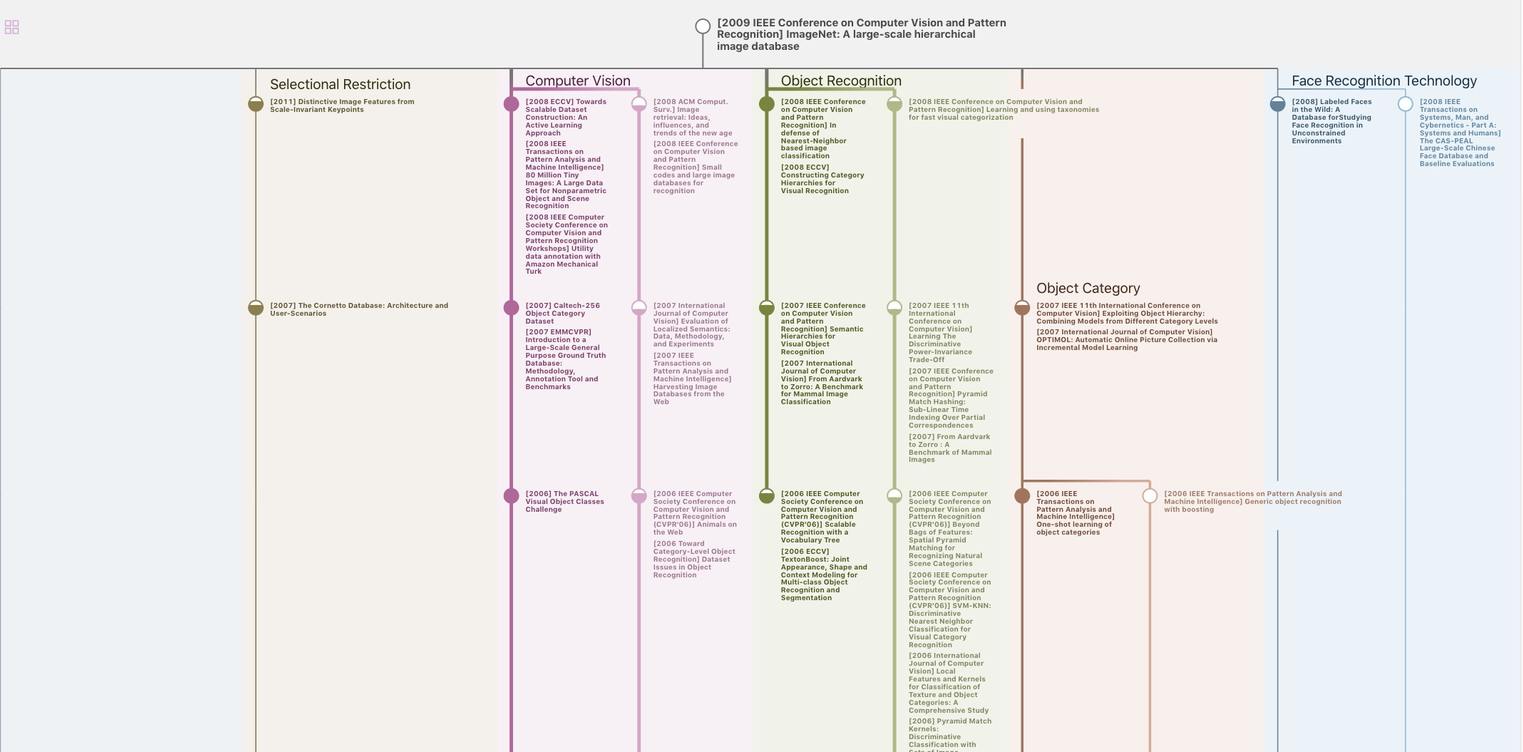
Generate MRT to find the research sequence of this paper
Chat Paper
Summary is being generated by the instructions you defined