Compressive Independent Component Analysis
2019 27TH EUROPEAN SIGNAL PROCESSING CONFERENCE (EUSIPCO)(2019)
Abstract
In this paper we investigate the minimal dimension statistic necessary in order to solve the independent component analysis (ICA) problem. We create a compressive learning framework for ICA and show for the first time that the memory complexity scales only quadratically with respect to the number of independent sources n, resulting in a vast improvement over other ICA methods. This is made possible by demonstrating a low dimensional model set, that exists in the cumulant based ICA problem, can be stably embedded into a compressed space from a larger dimensional cumulant tensor space. We show that identifying independent source signals can be achieved with high probability when the compression size m is of the optimal order of the intrinsic dimension of the ICA parameters and propose a iterative projection gradient algorithm to achieve this.
MoreTranslated text
Key words
Compressive learning, random moments, compressive sensing, independent component analysis, statistical learning
AI Read Science
Must-Reading Tree
Example
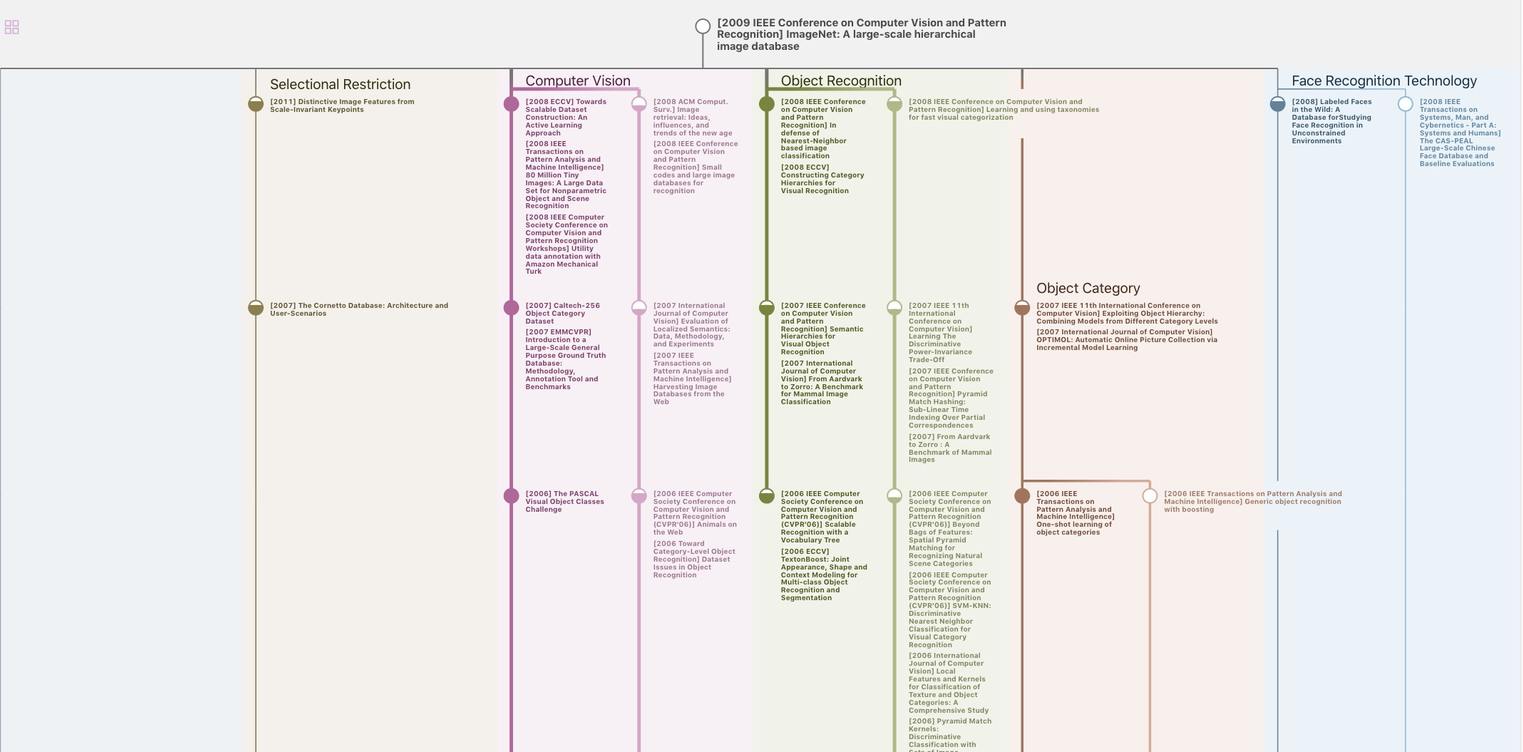
Generate MRT to find the research sequence of this paper
Chat Paper
Summary is being generated by the instructions you defined