Comparing Insights From Inductive Qualitative Analysis Versus Automated NLP Algorithms For Analyzing Feedback In Digital Randomized Controlled Trials
2019 45th Euromicro Conference on Software Engineering and Advanced Applications (SEAA)(2019)
摘要
Randomized controlled trials (i.e. A/B testing) is the gold standard for evaluating improvements and accelerating innovations in the digital space. Prior work has shown that qualitative user feedback is an effective and important tool in the analysis of A/B tests. However, manual inductive qualitative analysis of feedback-best practices today-is expensive and unscalable, which may lead to the omission of important insights about quality and user experiences. Prior work has shown various automated NLP algorithms to be effective in extracting insights from feedback in the digital domain. But we lack understanding of the differences in insights gained from a manual inductive qualitative analysis versus automated NLP algorithms, for analyzing digital randomized controlled trials (where a key objective is understanding differences between control and treatment conditions). In this paper, we compare insights from manual inductive qualitative analyses and from six automated NLP algorithms, using data from large-scale real-world digital randomized controlled trials. We find that collocation algorithms (notable, trigrams) are promising, providing similar insights as manual analyses with substantially lower cost; however, issues remain and improvements are needed. We discuss implications for future research and for operationalization.
更多查看译文
关键词
a/b testing,experimentation,text analysis,qualitative analysis,NLP,randomized controlled trials
AI 理解论文
溯源树
样例
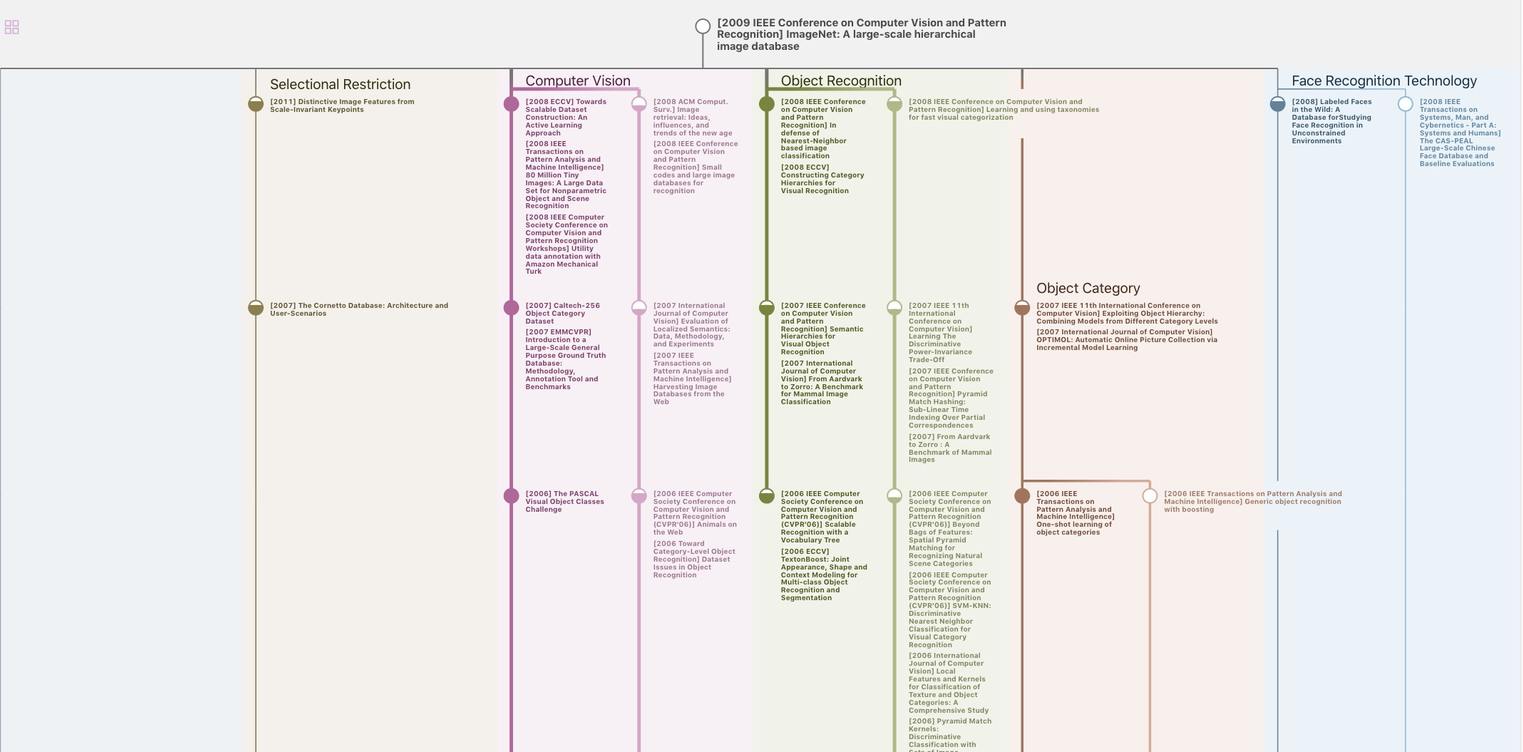
生成溯源树,研究论文发展脉络
Chat Paper
正在生成论文摘要