An Efficient $\varepsilon$-BIC to BIC Transformation and Its Application to Black-Box Reduction in Revenue Maximization
arxiv(2019)
摘要
We consider the black-box reduction from multi-dimensional revenue maximization to virtual welfare maximization. Cai et al.~\cite{CaiDW12a, CaiDW12b,CaiDW13a,CaiDW13b} show a polynomial-time approximation-preserving reduction, however, the mechanism produced by their reduction is only approximately Bayesian incentive compatible ($\varepsilon$-BIC). We provide a new polynomial time transformation that converts any $\varepsilon$-BIC mechanism to an exactly BIC mechanism with only a negligible revenue loss. Our transformation applies to a very general mechanism design setting and only requires sample access to the agents' type distributions and query access to the original $\varepsilon$-BIC mechanism. Other $\varepsilon$-BIC to BIC transformations exist in the literature~\cite{DaskalakisW12, RubinsteinW15, CaiZ17} but all require exponential time to run. As an application of our transformation, we improve the reduction by Cai et al.~\cite{CaiDW12a, CaiDW12b,CaiDW13a,CaiDW13b} to generate an exactly BIC mechanism. Our transformation builds on a novel idea developed in a recent paper by Dughmi et al.~\cite{dughmi2017bernoulli}: finding the maximum entropy regularized matching using Bernoulli factories. The original algorithm by Dughmi et al.~\cite{dughmi2017bernoulli} can only handle graphs with nonnegative edge weights, while our transformation requires finding the maximum entropy regularized matching on graphs with both positive and negative edge weights. The main technical contribution of this paper is a new algorithm that can accommodate arbitrary edge weights.
更多查看译文
AI 理解论文
溯源树
样例
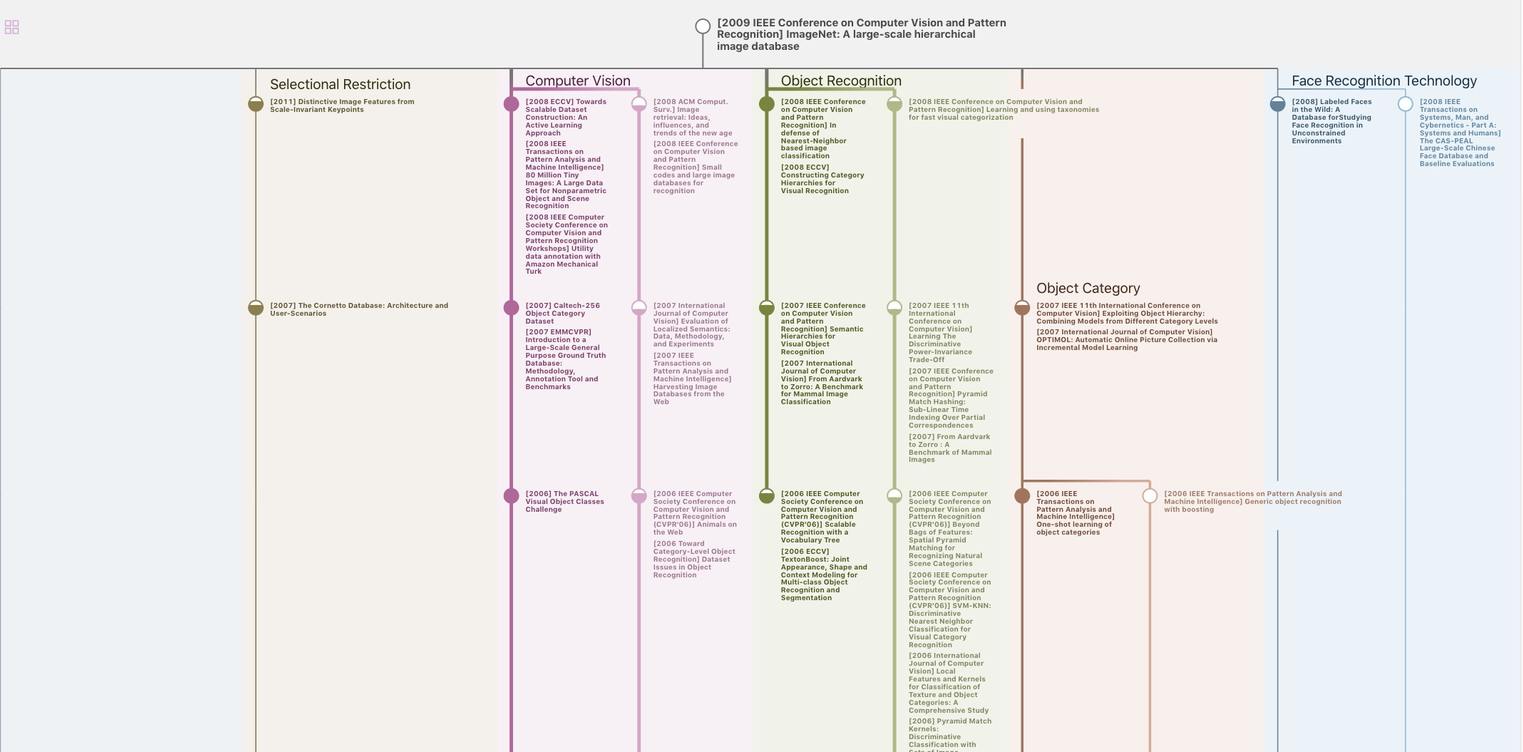
生成溯源树,研究论文发展脉络
Chat Paper
正在生成论文摘要