CloudFCN: Accurate and Robust Cloud Detection for Satellite Imagery with Deep Learning.
REMOTE SENSING(2019)
摘要
Cloud masking is of central importance to the Earth Observation community. This paper deals with the problem of detecting clouds in visible and multispectral imagery from high-resolution satellite cameras. Recently, Machine Learning has offered promising solutions to the problem of cloud masking, allowing for more flexibility than traditional thresholding techniques, which are restricted to instruments with the requisite spectral bands. However, few studies use multi-scale features (as in, a combination of pixel-level and spatial) whilst also offering compelling experimental evidence for real-world performance. Therefore, we introduce CloudFCN, based on a Fully Convolutional Network architecture, known as U-net, which has become a standard Deep Learning approach to image segmentation. It fuses the shallowest and deepest layers of the network, thus routing low-level visible content to its deepest layers. We offer an extensive range of experiments on this, including data from two high-resolution sensors-Carbonite-2 and Landsat 8-and several complementary tests. Owing to a variety of performance-enhancing design choices and training techniques, it exhibits state-of-the-art performance where comparable to other methods, high speed, and robustness to many different terrains and sensor types.
更多查看译文
关键词
clouds,deep learning,machine learning,computer vision,multispectral,optical
AI 理解论文
溯源树
样例
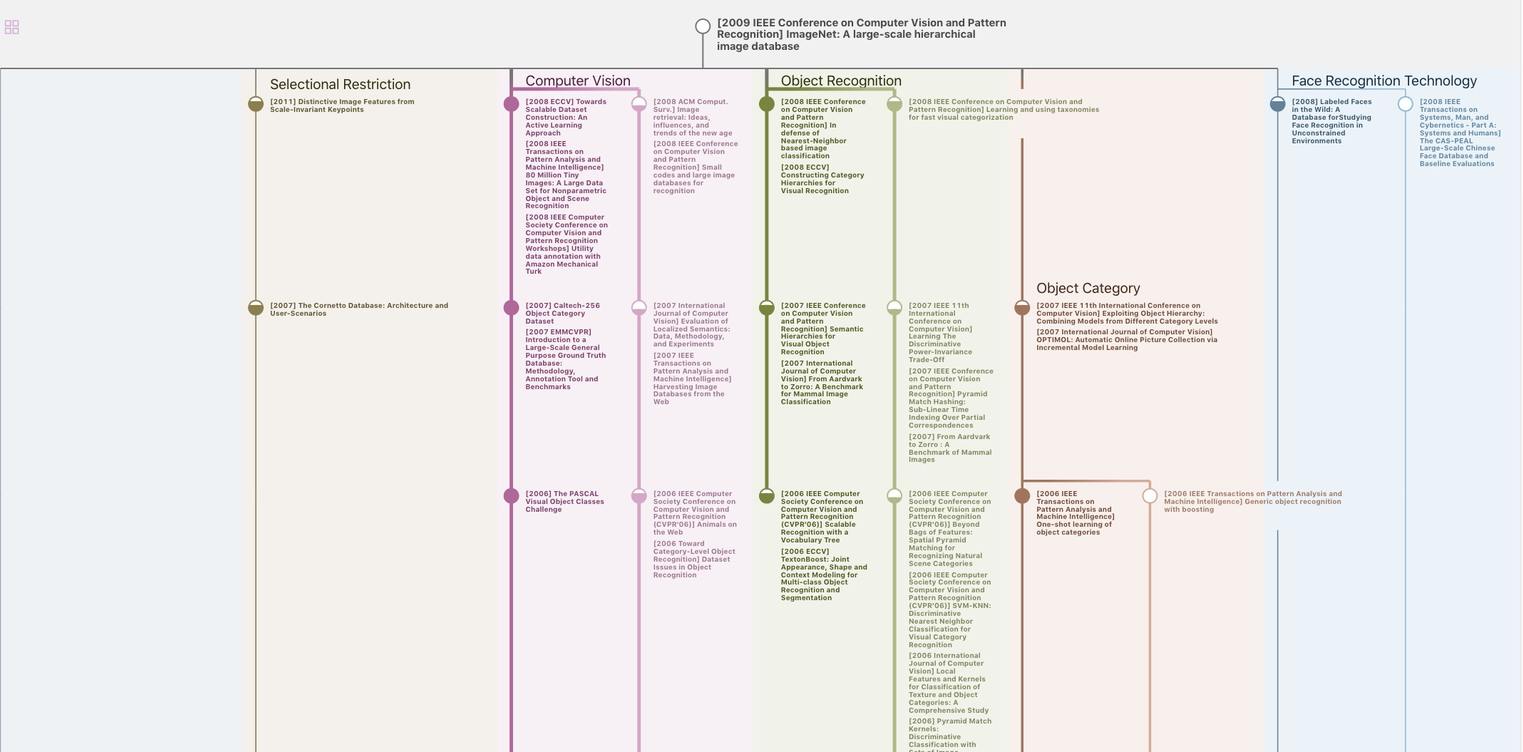
生成溯源树,研究论文发展脉络
Chat Paper
正在生成论文摘要