Clustering-independent analysis of genomic data using spectral simplicial theory.
PLOS COMPUTATIONAL BIOLOGY(2019)
摘要
Author summary Manifold learning methods have emerged as a way of analyzing the large high-dimensional data sets that are currently generated in many areas of science. They assume the data has been sampled from an unknown manifold which is approximated with a graph and utilize spectral graph techniques to perform unsupervised feature selection and dimensionality reduction. However, graphs provide only partial approximations to manifolds, precluding the application to features with a complex combinatorial structure. Relatedly, these methods cannot take into account the topology of the manifold. In this work, we extend spectral methods for feature selection to topological spaces built from data and present a general framework for feature selection. We present specific applications of this framework to clustering-independent analysis of gene expression and multi-modal genomic data. In particular, using these methods, we perform differential expression analysis in situations where samples cannot be grouped into distinct classes, and we disaggregate the results according to topological features of the expression space. In addition, we identify genes with spatial patterns of expression using spatially-resolved transcriptomic data and establish associations between genetic alterations and global expression patterns in large cross-sectional cancer studies. The prevailing paradigm for the analysis of biological data involves comparing groups of replicates from different conditions (e.g. control and treatment) to statistically infer features that discriminate them (e.g. differentially expressed genes). However, many situations in modern genomics such as single-cell omics experiments do not fit well into this paradigm because they lack true replicates. In such instances, spectral techniques could be used to rank features according to their degree of consistency with an underlying metric structure without the need to cluster samples. Here, we extend spectral methods for feature selection to abstract simplicial complexes and present a general framework for clustering-independent analysis. Combinatorial Laplacian scores take into account the topology spanned by the data and reduce to the ordinary Laplacian score when restricted to graphs. We demonstrate the utility of this framework with several applications to the analysis of gene expression and multi-modal genomic data. Specifically, we perform differential expression analysis in situations where samples cannot be grouped into distinct classes, and we disaggregate differentially expressed genes according to the topology of the expression space (e.g. alternative paths of differentiation). We also apply this formalism to identify genes with spatial patterns of expression using fluorescence in-situ hybridization data and to establish associations between genetic alterations and global expression patterns in large cross-sectional studies. Our results provide a unifying perspective on topological data analysis and manifold learning approaches to the analysis of large-scale biological datasets.
更多查看译文
关键词
spectral simplicial theory,genomic data,clustering-independent
AI 理解论文
溯源树
样例
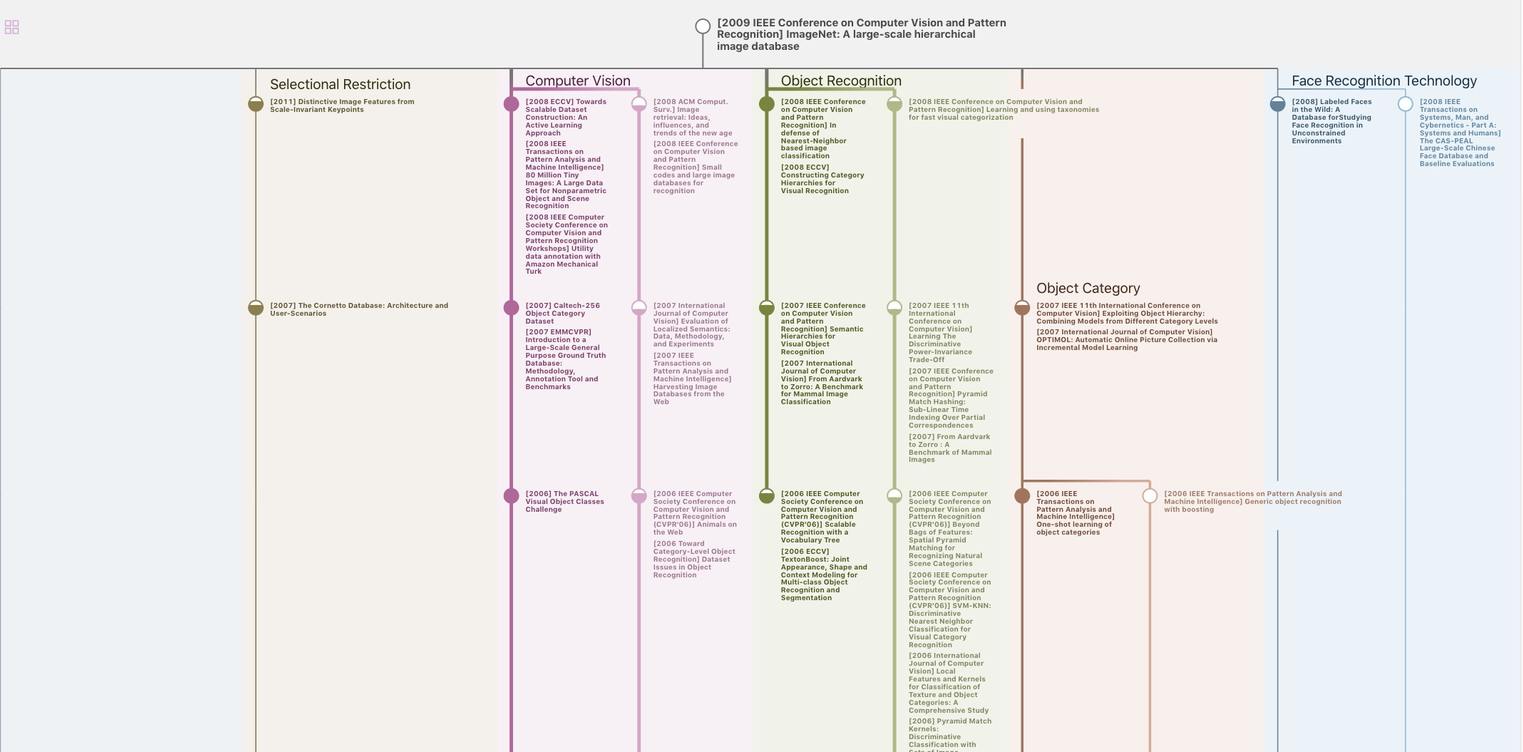
生成溯源树,研究论文发展脉络
Chat Paper
正在生成论文摘要