High-throughput phenotyping with electronic medical record data using a common semi-supervised approach (PheCAP)
NATURE PROTOCOLS(2019)
Abstract
Phenotypes are the foundation for clinical and genetic studies of disease risk and outcomes. The growth of biobanks linked to electronic medical record (EMR) data has both facilitated and increased the demand for efficient, accurate, and robust approaches for phenotyping millions of patients. Challenges to phenotyping with EMR data include variation in the accuracy of codes, as well as the high level of manual input required to identify features for the algorithm and to obtain gold standard labels. To address these challenges, we developed PheCAP, a high-throughput semi-supervised phenotyping pipeline. PheCAP begins with data from the EMR, including structured data and information extracted from the narrative notes using natural language processing (NLP). The standardized steps integrate automated procedures, which reduce the level of manual input, and machine learning approaches for algorithm training. PheCAP itself can be executed in 1–2 d if all data are available; however, the timing is largely dependent on the chart review stage, which typically requires at least 2 weeks. The final products of PheCAP include a phenotype algorithm, the probability of the phenotype for all patients, and a phenotype classification (yes or no).
MoreTranslated text
Key words
Bioinformatics,Biomarkers,Diseases,Software,Life Sciences,general,Biological Techniques,Analytical Chemistry,Microarrays,Computational Biology/Bioinformatics,Organic Chemistry
AI Read Science
Must-Reading Tree
Example
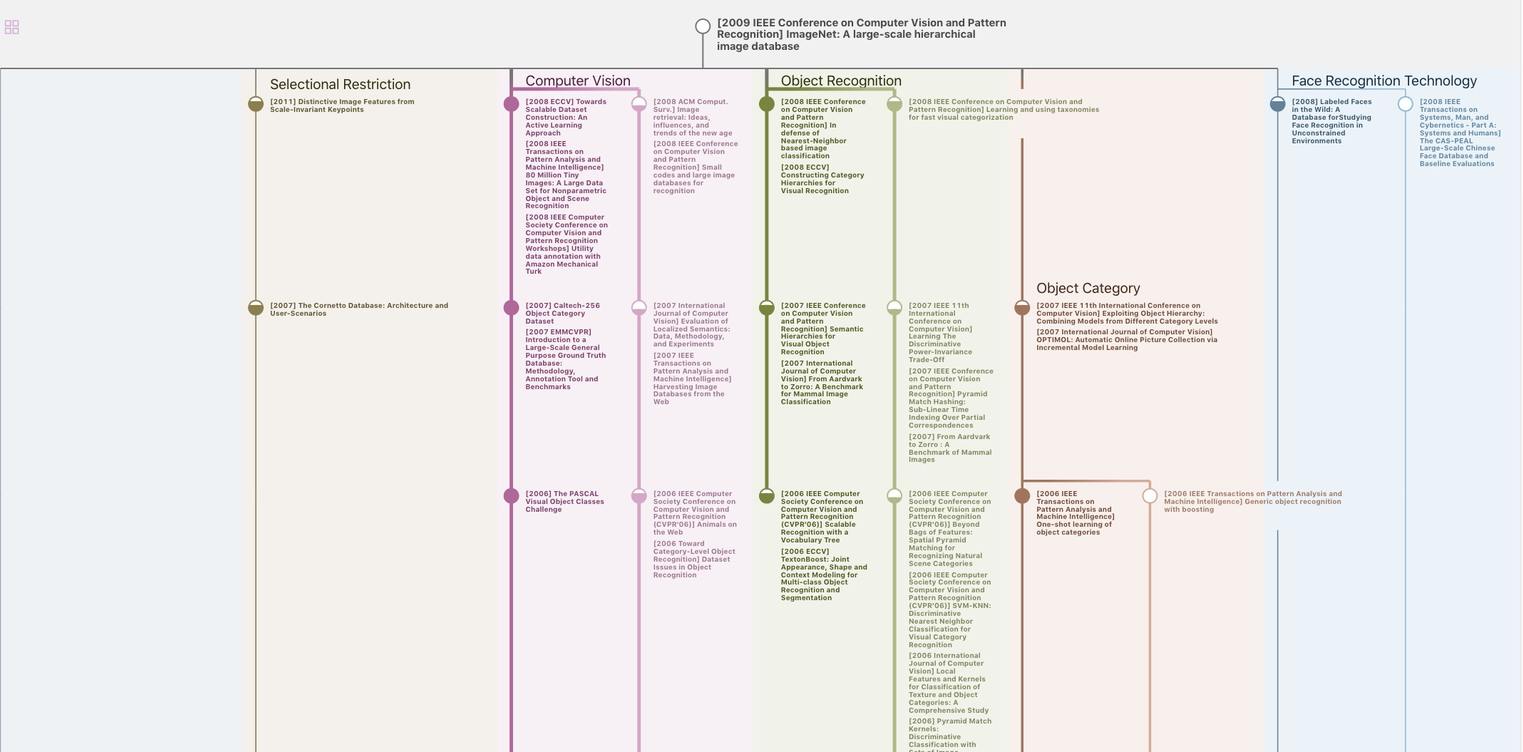
Generate MRT to find the research sequence of this paper
Chat Paper
Summary is being generated by the instructions you defined