Clustering Hyperspectral Images Via Sparse Dictionary Learning with Joint Sparsity and Shared Wavelets.
IGARSS(2019)
摘要
Sparse subspace clustering (SSC) algorithm has achieved an impressive performances in hyperspectral images clustering. However, the raw samples contained noises were used to construct the dictionary. Moreover, SSC represented each signal individually ignoring the relationship among hyperspectral pixels. To overcome these problems, we propose a sparse dictionary learning method for hyperspectral images clustering, in which joint sparsity and shared Wavelets are integrated to improve the expressive power of the learnt dictionary. First, we incorporate the shared Wavelets as a base dictionary into a unified joint sparsity constrained optimizing model to learn a structured sparse dictionary from both spectral and contextual characteristics of hyperspectral images. Then, the sparse representation coefficients based on the learnt sparse dictionary are adopted to construct a non-negative affinity matrix of graph. Finally, spectral clustering is employed to the affinity matrix to obtain the final clustering result. Experimental results clearly demonstrate that the proposed algorithm outperforms other state-of-the-art methods on the hyperspectral dataset.
更多查看译文
关键词
sparse subspace clustering, joint sparsity, sparse dictionary, affinity matrix, spectral clustering
AI 理解论文
溯源树
样例
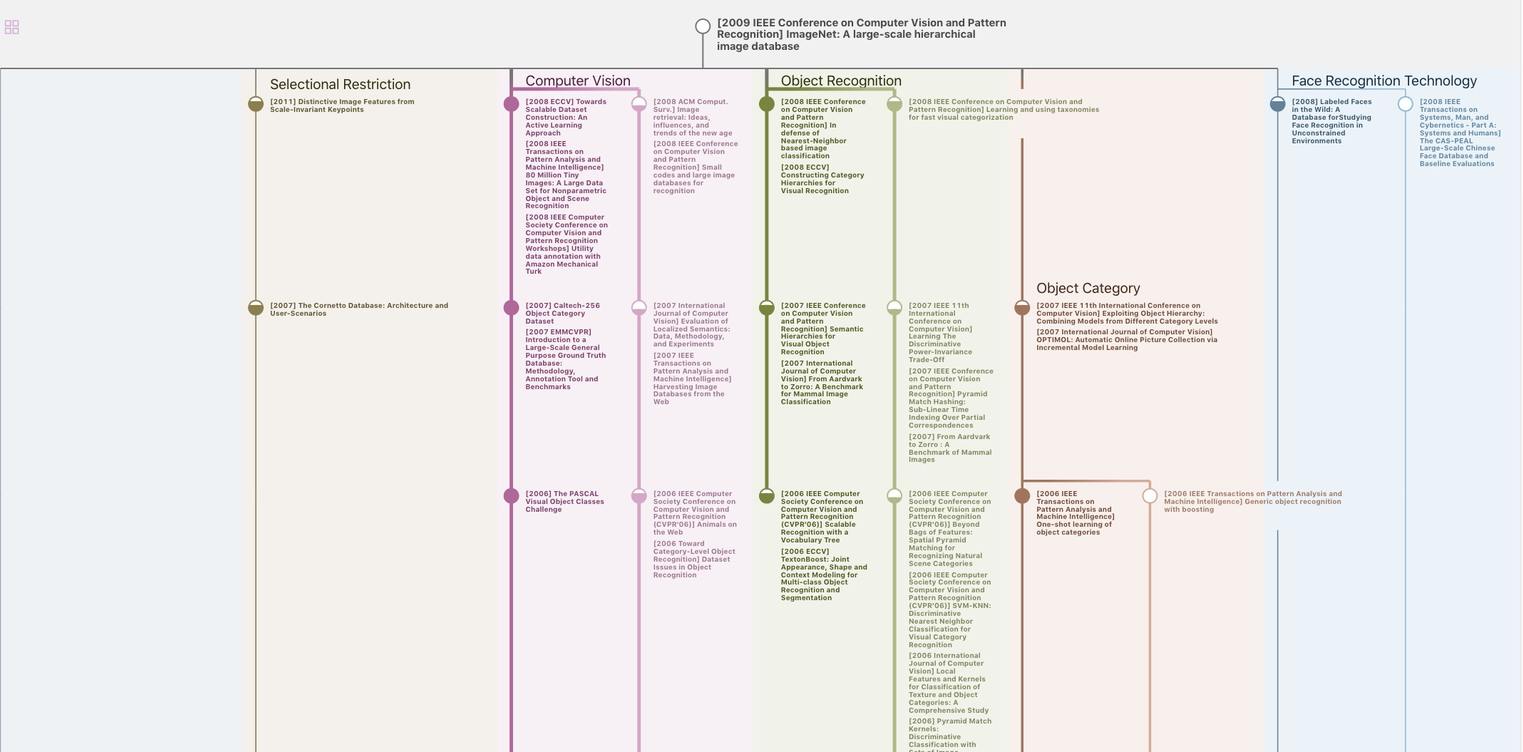
生成溯源树,研究论文发展脉络
Chat Paper
正在生成论文摘要