Generating An Evolving Skills Network From Job Adverts For High-Demand Skillset Discovery
WEB INFORMATION SYSTEMS ENGINEERING - WISE 2019(2019)
摘要
Understanding the needs of highly-dynamic job market sectors is of crucial importance to job seekers, employers, and educational bodies alike. This paper describes efforts to identify skill demand composition and dynamics by constructing and interpreting a time series of skills networks that are routinely identified through an established agglomerative hierarchical clustering with breadth-first search order based on co-word occurrences. We focus on Data Science as an example of a highly dynamic sector. Data collected from job adverts between 2016-2017 is pre-processed to identify distinct evolving skills networks observed over at least 12 months. These result in 40 time-series that are used to track the evolving skills clusters and to define the skillsets in high-demand. To return a quantitative scientific result, we implement three traditional statistical models (Naive, Simple Exponential Smoothing (SES), and Holt's linear trend) to forecast future skills cluster composition. The analysis is done based on the centrality and density indices generated for each evolving cluster within the skills networks. Forecasts based on the previous quarter(s) are then checked against actual observations in terms of positioning within a density- and centrality-based strategic quadrant. The F-measures observed (75% and 73% for two top methods) demonstrate the suitability of our approach to identify core skillsets in the near future based on recent data with a high level of accuracy.
更多查看译文
关键词
Graph mining, Network clustering, Time-evolving network
AI 理解论文
溯源树
样例
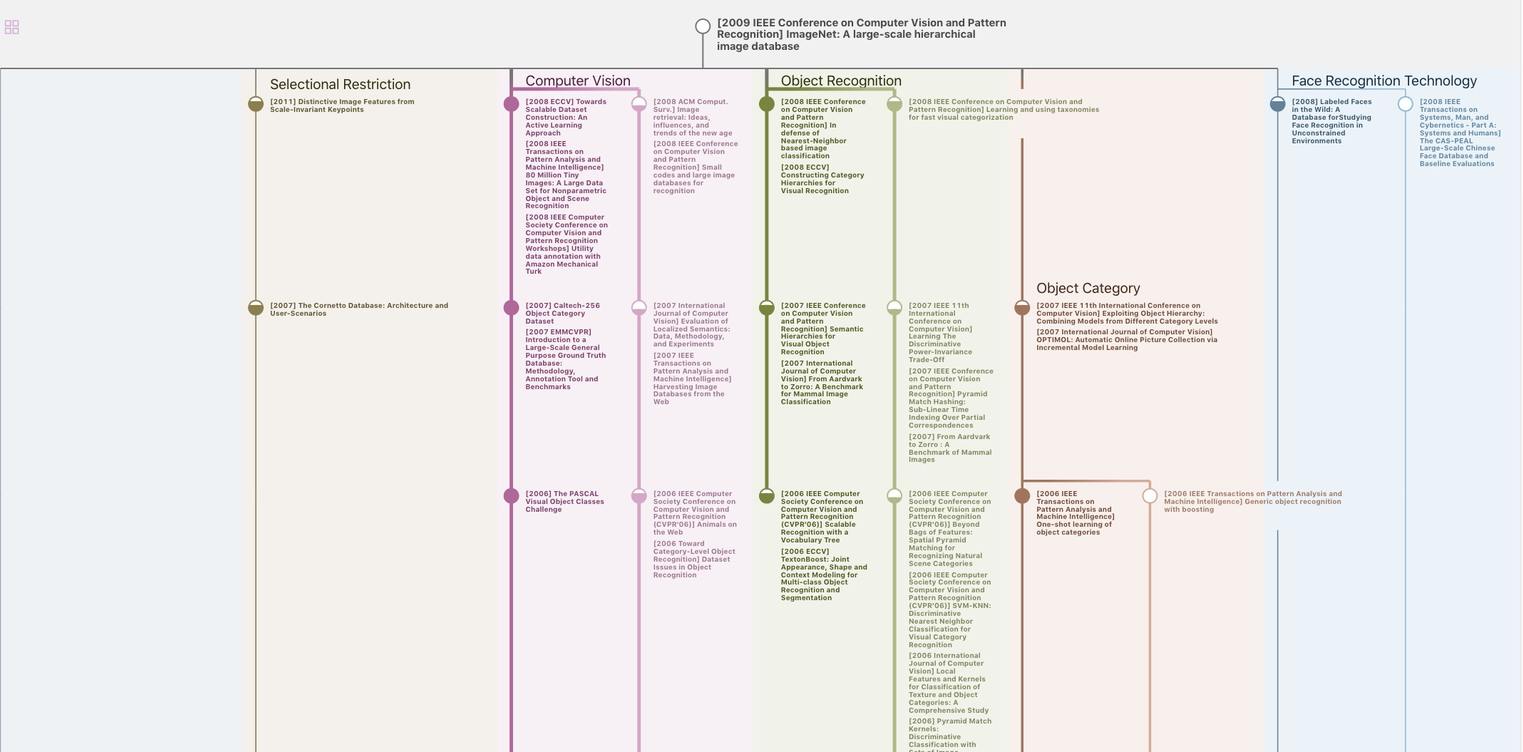
生成溯源树,研究论文发展脉络
Chat Paper
正在生成论文摘要