Learning Weighted Top-$k$ Support Vector Machine.
ACML(2019)
摘要
Top-k error ratio is a popular performance measure for multi-category classification in which the number of categories is large. With the aim of obtaining the multi-category classifier minimizing the top-k error, Lapin et al. has developed the top-k support vector machine (top-k SVM) which is trained with the top-k hinge loss. Although top-k hinge is designed to be suitable for the top-k error, another loss or the top-k' hinge loss with k' ≠ k often yields a smaller top-k error ratio than the top-k hinge loss. This suggests that the top-k hinge loss is not always the optimal choice for the top-k error, which motivates us to explore variants of the top-k hinge loss. In this paper, we studied a weighted variant of the top-k hinge loss, and refer to the learning machine as the weighted top-k SVM. We developed a new optimization algorithm based on the Frank-Wolfe algorithm that requires no step size, enjoys the clear stopping criterion, and is never solicitous for computational instability. The Frank-Wolfe algorithm repeats the direction finding step and the line search step. The discoveries in this study are that both the steps can be given in a closed form. By smoothing the loss function, geometrical convergence can be achieved. Experimental results reveal that the weighted top-k SVM often achieved the better pattern recognition performance compared to the unweighted top-k SVM.
更多查看译文
关键词
support vector machine,learning
AI 理解论文
溯源树
样例
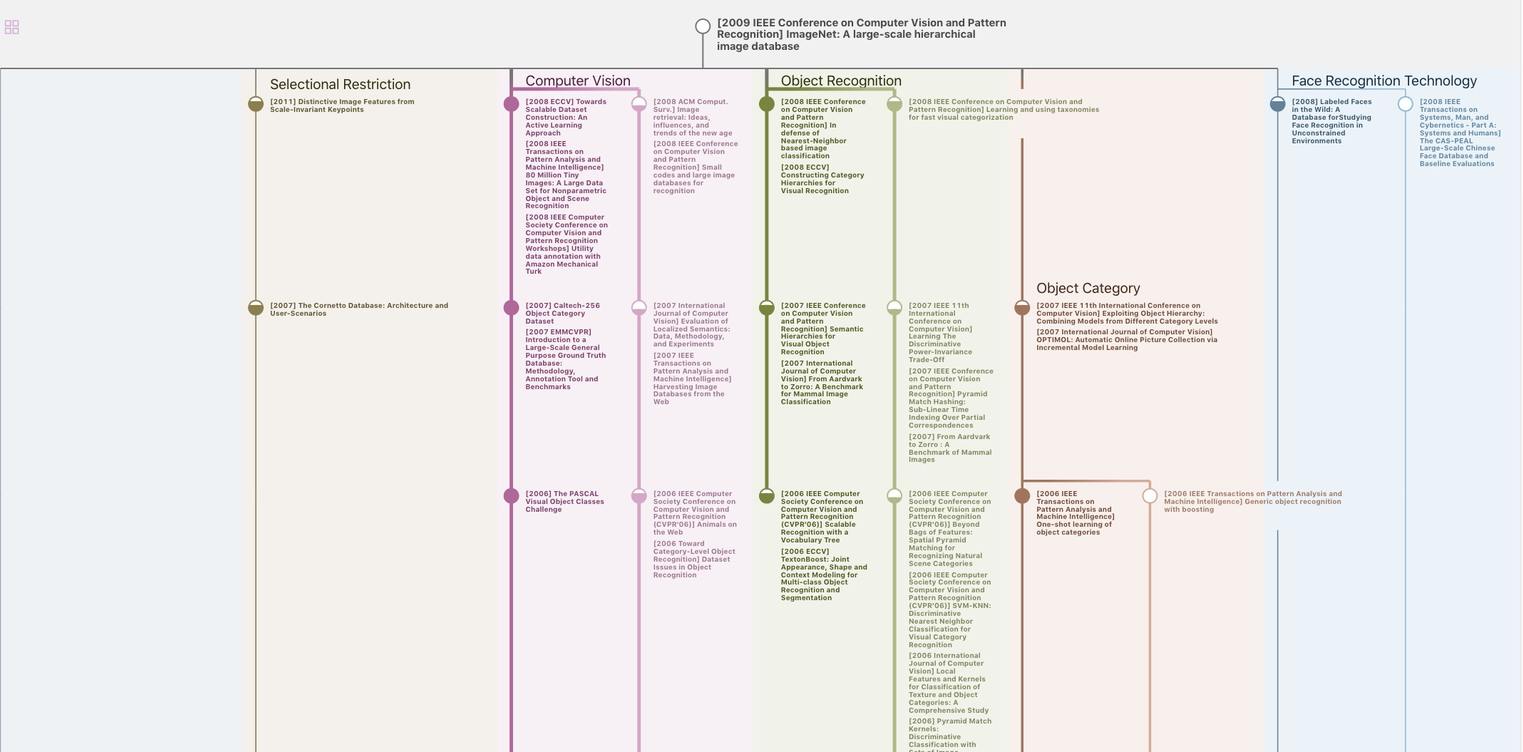
生成溯源树,研究论文发展脉络
Chat Paper
正在生成论文摘要