Exploiting Oxide Based Resistive RAM Variability for Probabilistic AI Hardware Design
arxiv(2019)
摘要
Uncertainty plays a key role in real-time machine learning. As a significant shift from standard deep networks, which do not overtly represent any uncertainty in its structure or output, Bayesian deep networks are being currently investigated where the network is envisaged as a set of plausible models learnt by the Bayes' formulation in response to uncertainties in sensory data. Bayesian deep networks formulate each synaptic weight as a sample drawn from a probability distribution with learnt mean and variance. This paper elaborates on a hardware design that exploits cycle-to-cycle variability of oxide based Resistive Random Access Memories (RRAMs) as a means to realize such a probabilistic sampling function, instead of viewing it as a disadvantage.
更多查看译文
关键词
probabilistic ai hardware design
AI 理解论文
溯源树
样例
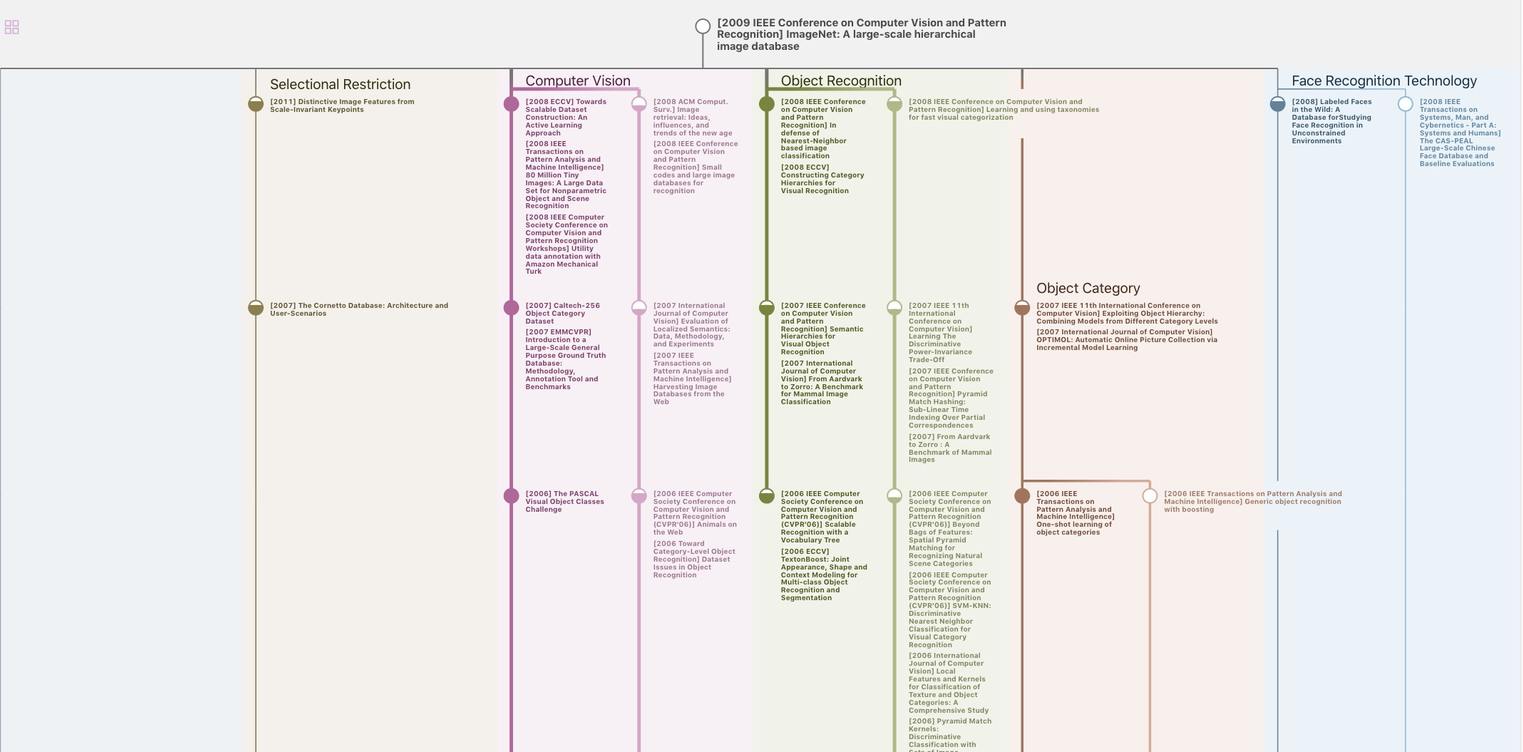
生成溯源树,研究论文发展脉络
Chat Paper
正在生成论文摘要