Computer-aided classifying and characterizing of methamphetamine use disorder using resting-state EEG
Cognitive Neurodynamics(2019)
摘要
Methamphetamine (meth) is potently addictive and is closely linked to high crime rates in the world. Since meth withdrawal is very painful and difficult, most abusers relapse to abuse in traditional treatments. Therefore, developing accurate data-driven methods based on brain functional connectivity could be helpful in classifying and characterizing the neural features of meth dependence to optimize the treatments. Accordingly, in this study, computation of functional connectivity using resting-state EEG was used to classify meth dependence. Firstly, brain functional connectivity networks (FCNs) of 36 meth dependent individuals and 24 normal controls were constructed by weighted phase lag index, in six frequency bands: delta (1–4 Hz), theta (4–8 Hz), alpha (8–15 Hz), beta (15–30 Hz), gamma (30–45 Hz) and wideband (1–45 Hz).Then, significant differences in graph metrics and connectivity values of the FCNs were used to distinguish the two groups. Support vector machine classifier had the best performance with 93% accuracy, 100% sensitivity, 83% specificity and 0.94 F-score for differentiating between MDIs and NCs. The best performance yielded when selected features were the combination of connectivity values and graph metrics in the beta frequency band.
更多查看译文
关键词
Support vector machine, Weighted phase lag index, Functional brain connectivity network, Electroencephalography, Meth dependence
AI 理解论文
溯源树
样例
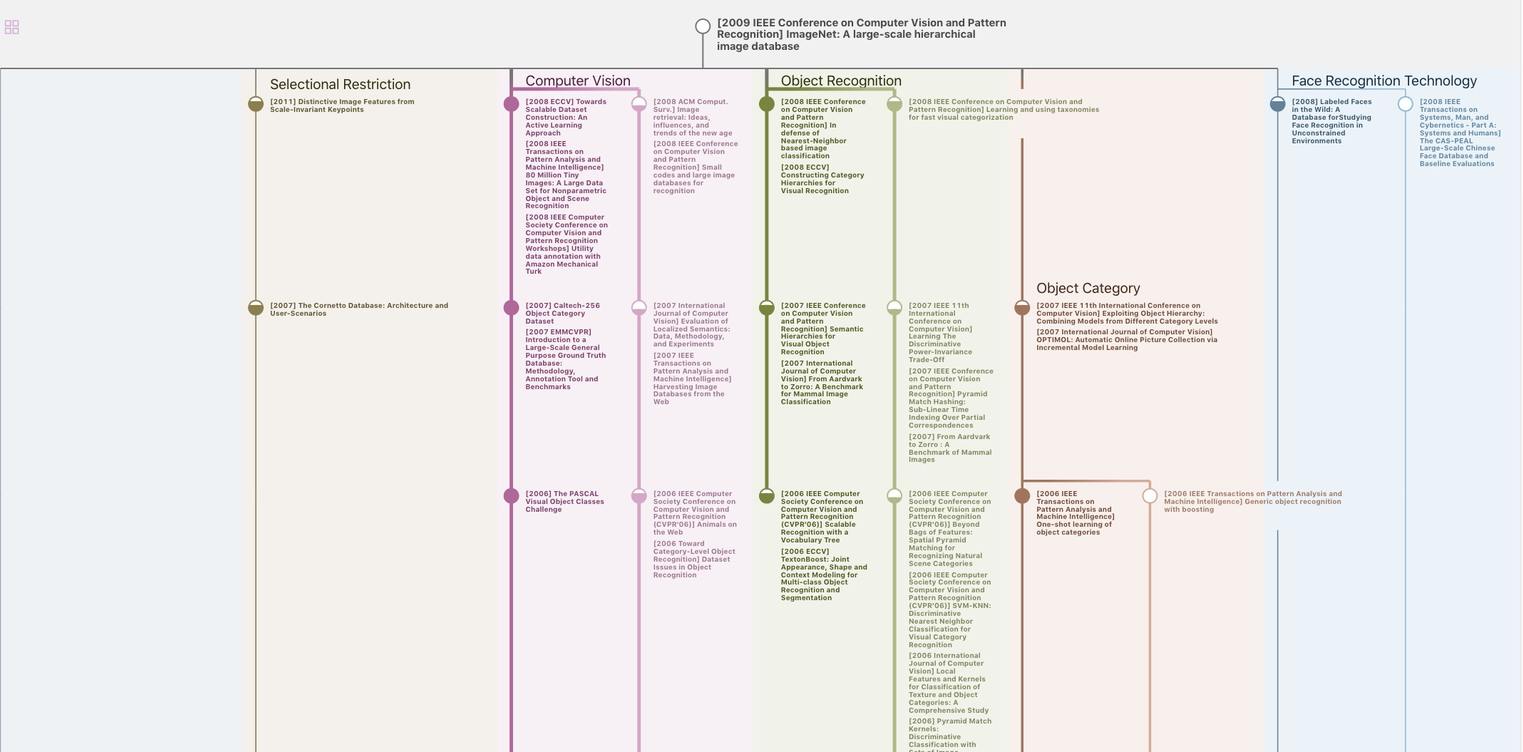
生成溯源树,研究论文发展脉络
Chat Paper
正在生成论文摘要