Neural Network based End-to-End Query by Example Spoken Term Detection
IEEE-ACM TRANSACTIONS ON AUDIO SPEECH AND LANGUAGE PROCESSING(2020)
摘要
This article focuses on the problem of query by example spoken term detection (QbE-STD) in zero-resource scenario. State-of-the-art approaches primarily rely on dynamic time warping (DTW) based template matching techniques using phone posterior or bottleneck features extracted from a deep neural network (DNN). We use both monolingual and multilingual bottleneck features, and show that multilingual features perform increasingly better with more training languages. Previously, it has been shown that the DTW based matching can be replaced with a CNN based matching while using posterior features. Here, we show that the CNN based matching outperforms DTW based matching using bottleneck features as well. In this case, the feature extraction and pattern matching stages of our QbE-STD system are optimized independently of each other. We propose to integrate these two stages in a fully neural network based end-to-end learning framework to enable joint optimization of those two stages simultaneously. The proposed approaches are evaluated on two challenging multilingual datasets: Spoken Web Search 2013 and Query by Example Search on Speech Task 2014, demonstrating in each case significant improvements.
更多查看译文
关键词
Feature extraction,Task analysis,Neural networks,Training,Hidden Markov models,Neurons,Speech processing,Spoken term detection,query by example,deep neural network,bottleneck features,end-to-end,subsequence detection
AI 理解论文
溯源树
样例
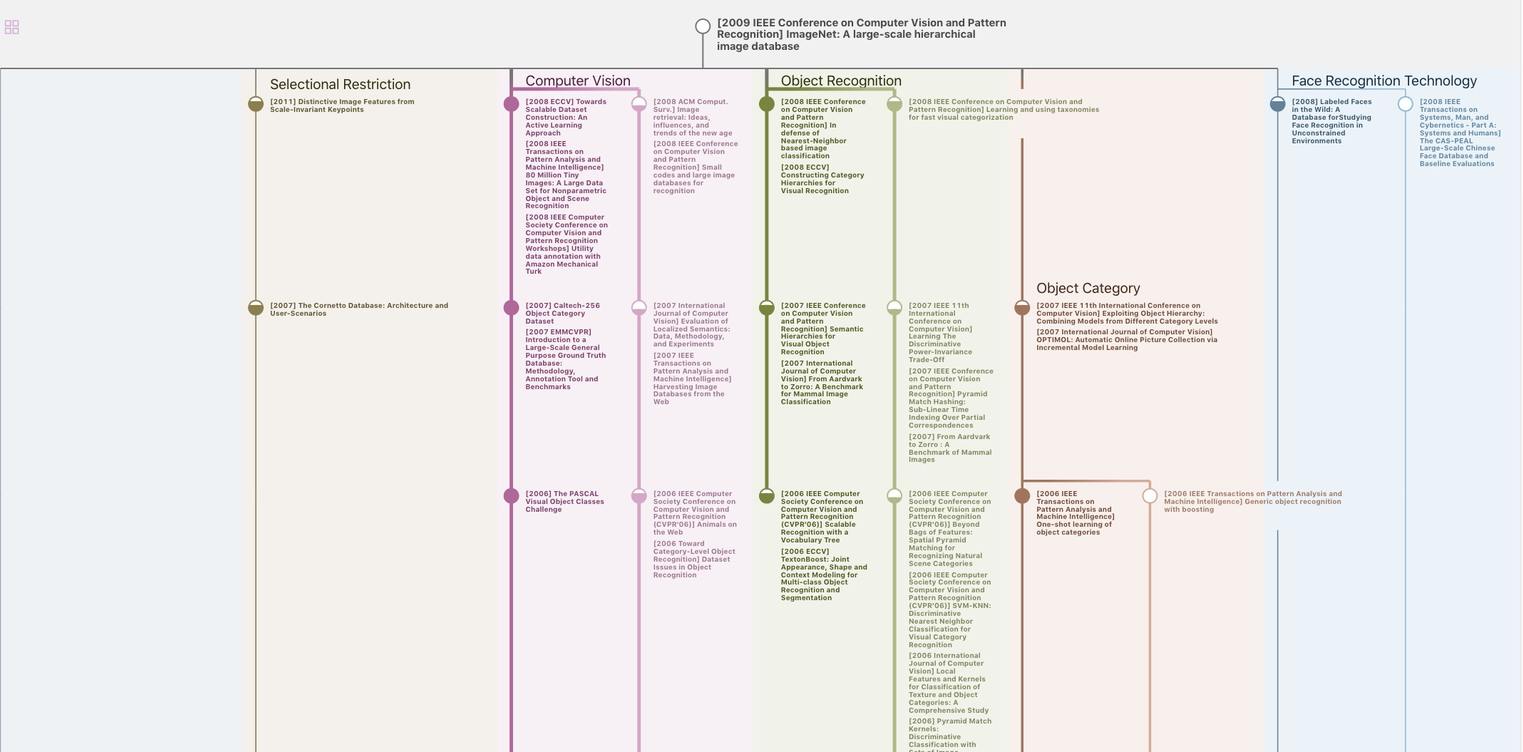
生成溯源树,研究论文发展脉络
Chat Paper
正在生成论文摘要