Automated Deep Learning For Threat Detection In Luggage From X-Ray Images
ANALYSIS OF EXPERIMENTAL ALGORITHMS, SEA2 2019(2019)
摘要
Luggage screening is a very important part of the airport security risk assessment and clearance process. Automating the threat objects detection from x-ray scans of passengers' luggage can speed-up and increase the efficiency of the whole security procedure. In this paper we investigate and compare several algorithms for detection of firearm parts in x-ray images of travellers' baggage. In particular, we focus on identifying steel barrel bores as threat objects, being the main part of the weapon needed for deflagration. For this purpose, we use a dataset of 22k double view x-ray scans, containing a mixture of benign and threat objects. In the pre-processing stage we apply standard filtering techniques to remove noisy and ambiguous images (i.e., smoothing, black and white thresholding, edge detection, etc.) and subsequently employ deep learning techniques (Convolutional Neural Networks and Stacked Autoencoders) for the classification task. For comparison purposes we also train and simulate shallow Neural Networks and Random Forests algorithms for the objects detection. Furthermore, we validate our findings on a second dataset of double view x-ray scans of courier parcels. We report and critically discuss the results of the comparison on both datasets, showing the advantages of our approach.
更多查看译文
关键词
Baggage screening, Deep learning, Convolutional neural networks, Image filtering, Object detection algorithms, X-ray images
AI 理解论文
溯源树
样例
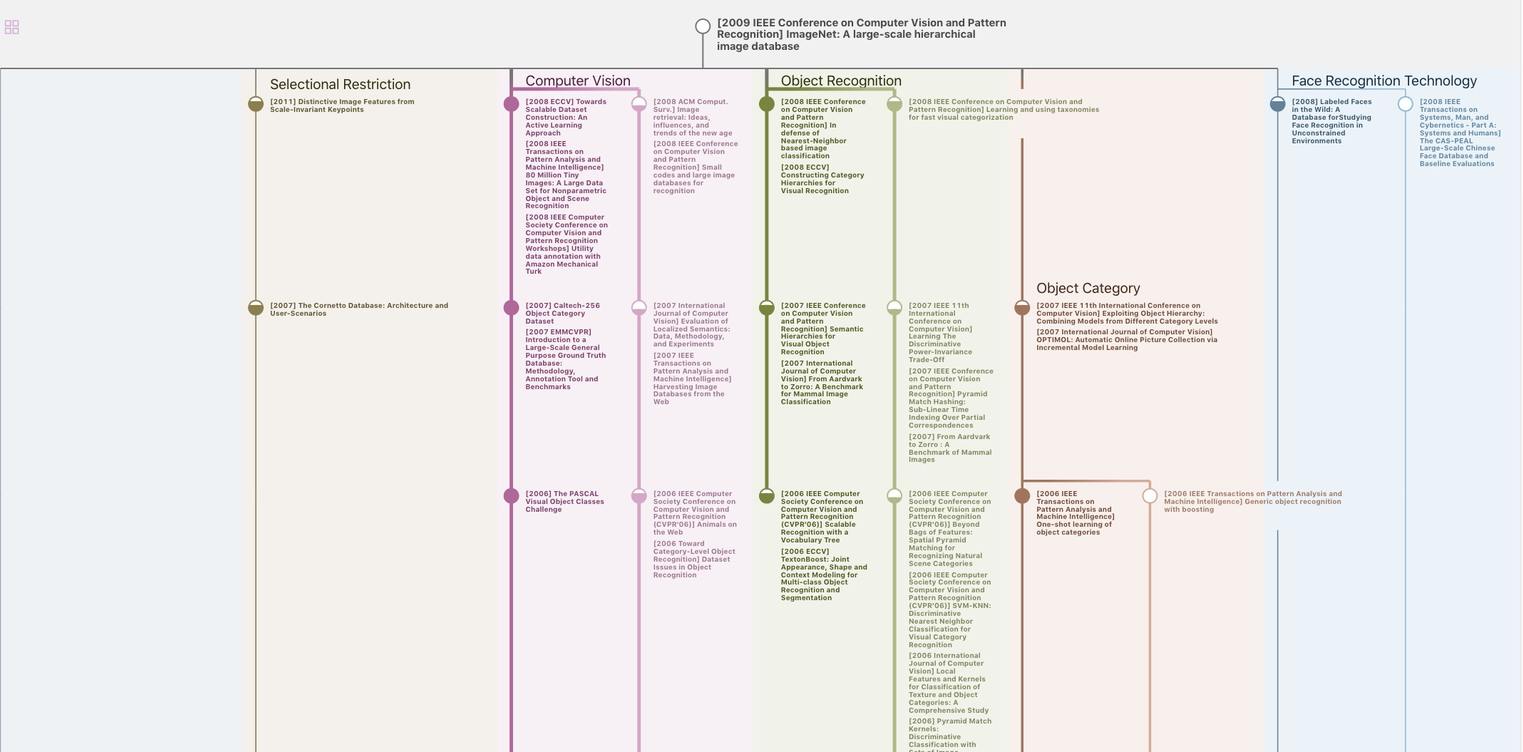
生成溯源树,研究论文发展脉络
Chat Paper
正在生成论文摘要