Global Vectors Representation Of Protein Sequences And Its Application For Predicting Self-Interacting Proteins With Multi-Grained Cascade Forest Model
GENES(2019)
摘要
Self-interacting proteins (SIPs) is of paramount importance in current molecular biology. There have been developed a number of traditional biological experiment methods for predicting SIPs in the past few years. However, these methods are costly, time-consuming and inefficient, and often limit their usage for predicting SIPs. Therefore, the development of computational method emerges at the times require. In this paper, we for the first time proposed a novel deep learning model which combined natural language processing (NLP) method for potential SIPs prediction from the protein sequence information. More specifically, the protein sequence is de novo assembled by k-mers. Then, we obtained the global vectors representation for each protein sequences by using natural language processing (NLP) technique. Finally, based on the knowledge of known self-interacting and non-interacting proteins, a multi-grained cascade forest model is trained to predict SIPs. Comprehensive experiments were performed on yeast and human datasets, which obtained an accuracy rate of 91.45% and 93.12%, respectively. From our evaluations, the experimental results show that the use of amino acid semantics information is very helpful for addressing the problem of sequences containing both self-interacting and non-interacting pairs of proteins. This work would have potential applications for various biological classification problems.
更多查看译文
关键词
self-interacting proteins,de novo protein sequence,global vector representation,multi-grained cascade forest
AI 理解论文
溯源树
样例
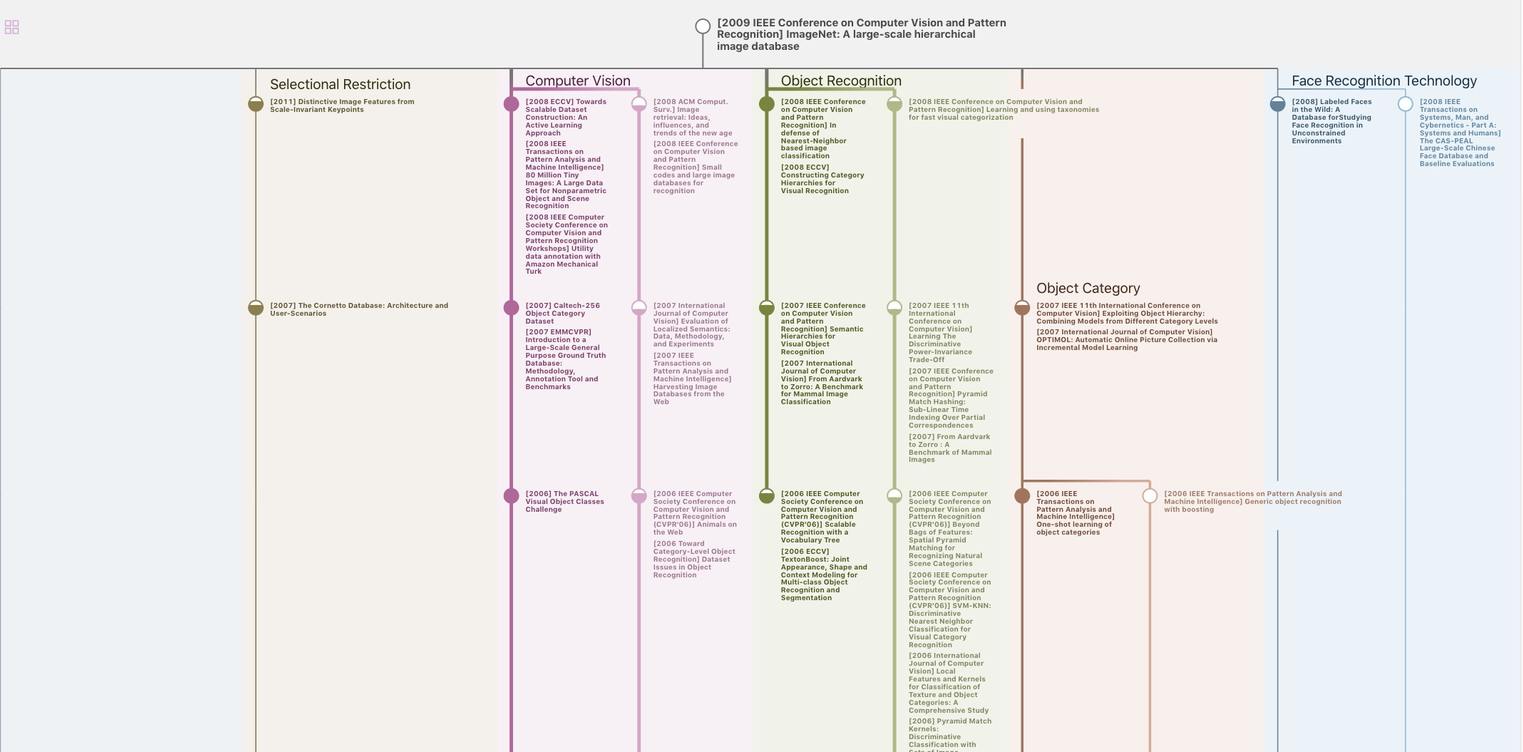
生成溯源树,研究论文发展脉络
Chat Paper
正在生成论文摘要