Accelerating Least Squares Imaging Using Deep Learning Techniques
arxiv(2019)
摘要
Wave equation techniques have been an integral part of geophysical imaging workflows to investigate the Earth's subsurface. Least-squares reverse time migration (LSRTM) is a linearized inversion problem that iteratively minimizes a misfit functional as a function of the model perturbation. The success of the inversion largely depends on our ability to handle large systems of equations given the massive computation costs. The size of the system almost exponentially increases with the demand for higher resolution images in complicated subsurface media. We propose an unsupervised deep learning approach that leverages the existing physics-based models and machine learning optimizers to achieve more accurate and cheaper solutions. We compare different optimizers and demonstrate their efficacy in mitigating imaging artifacts. Further, minimizing the Huber loss with mini-batch gradients and Adam optimizer is not only less memory-intensive but is also more robust. We demonstrate high accuracy predictions on complex synthetic scientific models that can generate noisy data.
更多查看译文
AI 理解论文
溯源树
样例
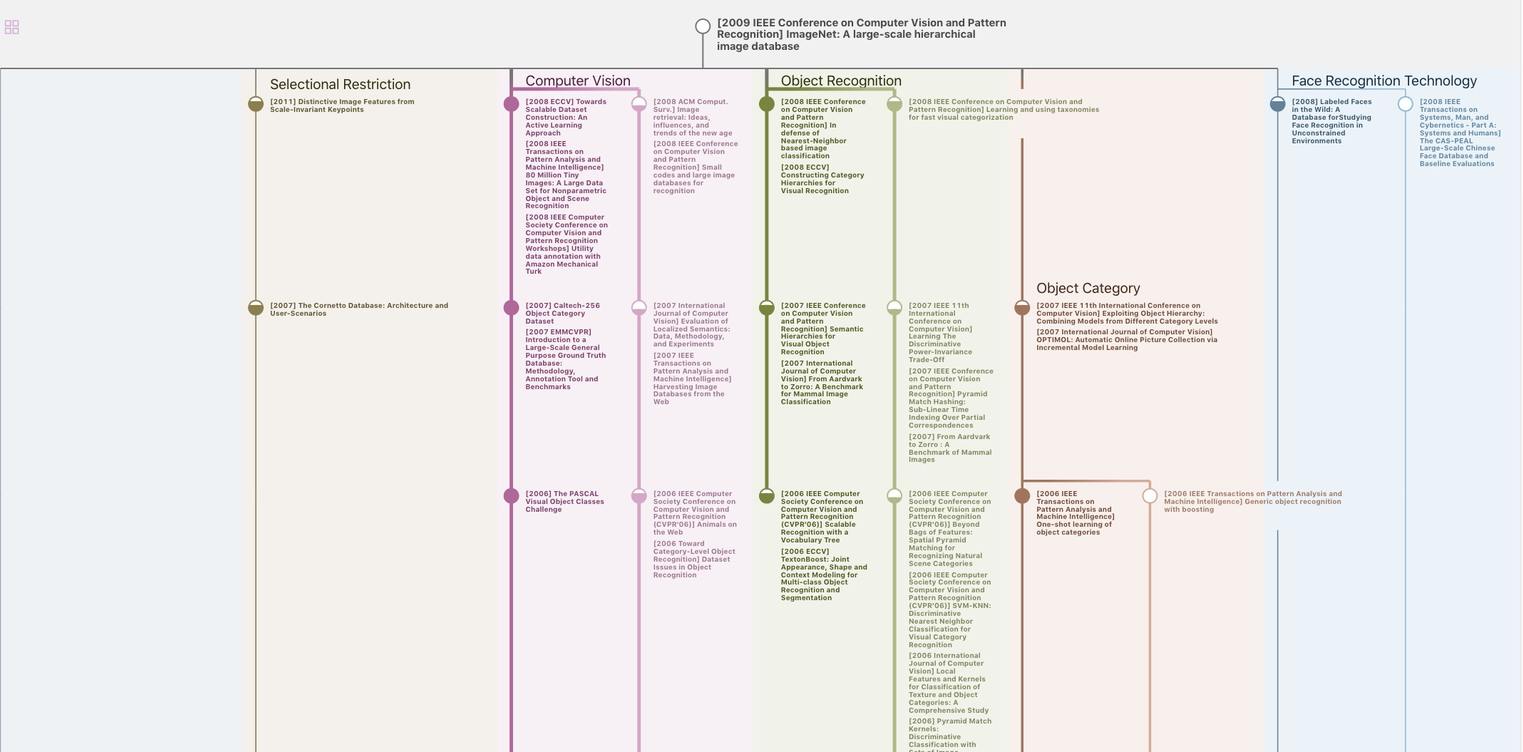
生成溯源树,研究论文发展脉络
Chat Paper
正在生成论文摘要