Combining attention-based bidirectional gated recurrent neural network and two-dimensional convolutional neural network for document-level sentiment classification
NEUROCOMPUTING(2020)
摘要
Neural networks lately have achieved a great success on sentiment classification due to their ability of feature extraction. However, it remains as an enormous challenge to model long texts in document-level sentiment classification as well as to explore semantics between sentences and dependencies between features. Moreover, most existing methods can hardly differentiate the importance of different contents when constructing a document representation. To tackle these problems, we propose a novel neural network model: AttDR-2DCNN, which mainly consists of two parts. The first part utilizes a two-layer compositional bidirectional Gated Recurrent Unit (GRU) to obtain the compositional semantics of the document, where the first layer learns the feature vector of the sentence, and the second layer learns the document matrix representation consisting of two dimensions of the time-step dimension and feature dimension, from the sentence vectors. The second part applies a two-dimensional convolution operation and two-dimensional max pooling to capture more dependencies between sentences features. We further utilize different types of attention mechanism in these two parts to distinguish the importance of words, sentences and features in the document. Experiments are conducted on four publicly available document-level review datasets and the result shows that the proposed model outperforms some existing models. (C) 2019 Elsevier B.V. All rights reserved.
更多查看译文
关键词
Sentiment classification,Attention network,2DCNN,Bidirectional GRU
AI 理解论文
溯源树
样例
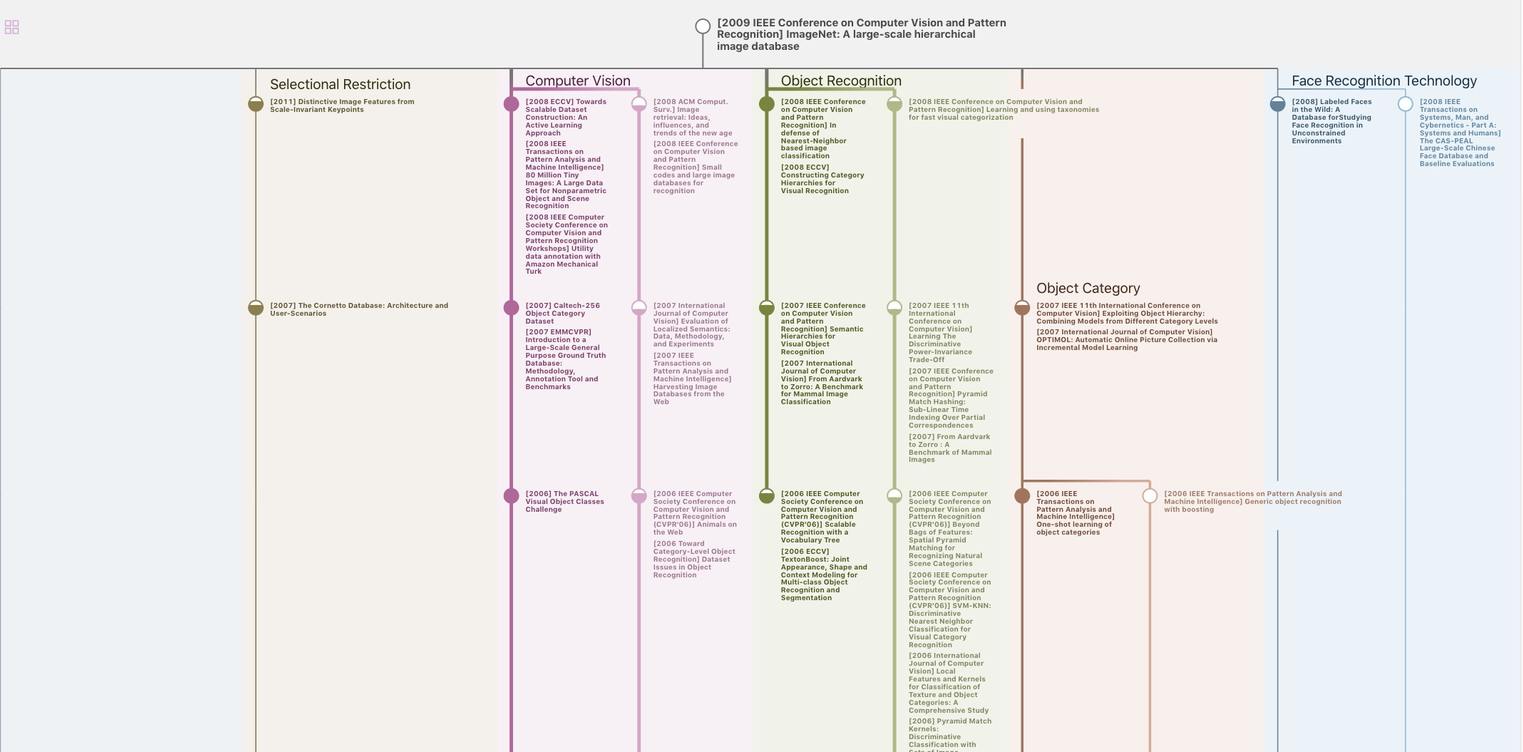
生成溯源树,研究论文发展脉络
Chat Paper
正在生成论文摘要