Evaluation Of Arima Models For Human-Machine Interface State Sequence Prediction
MACHINE LEARNING AND KNOWLEDGE EXTRACTION(2019)
摘要
In this paper, auto-regressive integrated moving average (ARIMA) time-series data forecast models are evaluated to ascertain their feasibility in predicting human-machine interface (HMI) state transitions, which are modeled as multivariate time-series patterns. Human-machine interface states generally include changes in their visually displayed information brought about due to both process parameter changes and user actions. This approach has wide applications in industrial controls, such as nuclear power plant control rooms and transportation industry, such as aircraft cockpits, etc., to develop non-intrusive real-time monitoring solutions for human operator situational awareness and potentially predicting human-in-the-loop error trend precursors.
更多查看译文
关键词
auto-regressive integrated moving average (ARIMA), human factor engineering (HFE), human–, machine interface (HMI), human-in-the-loop (HITL), situational awareness (SA)
AI 理解论文
溯源树
样例
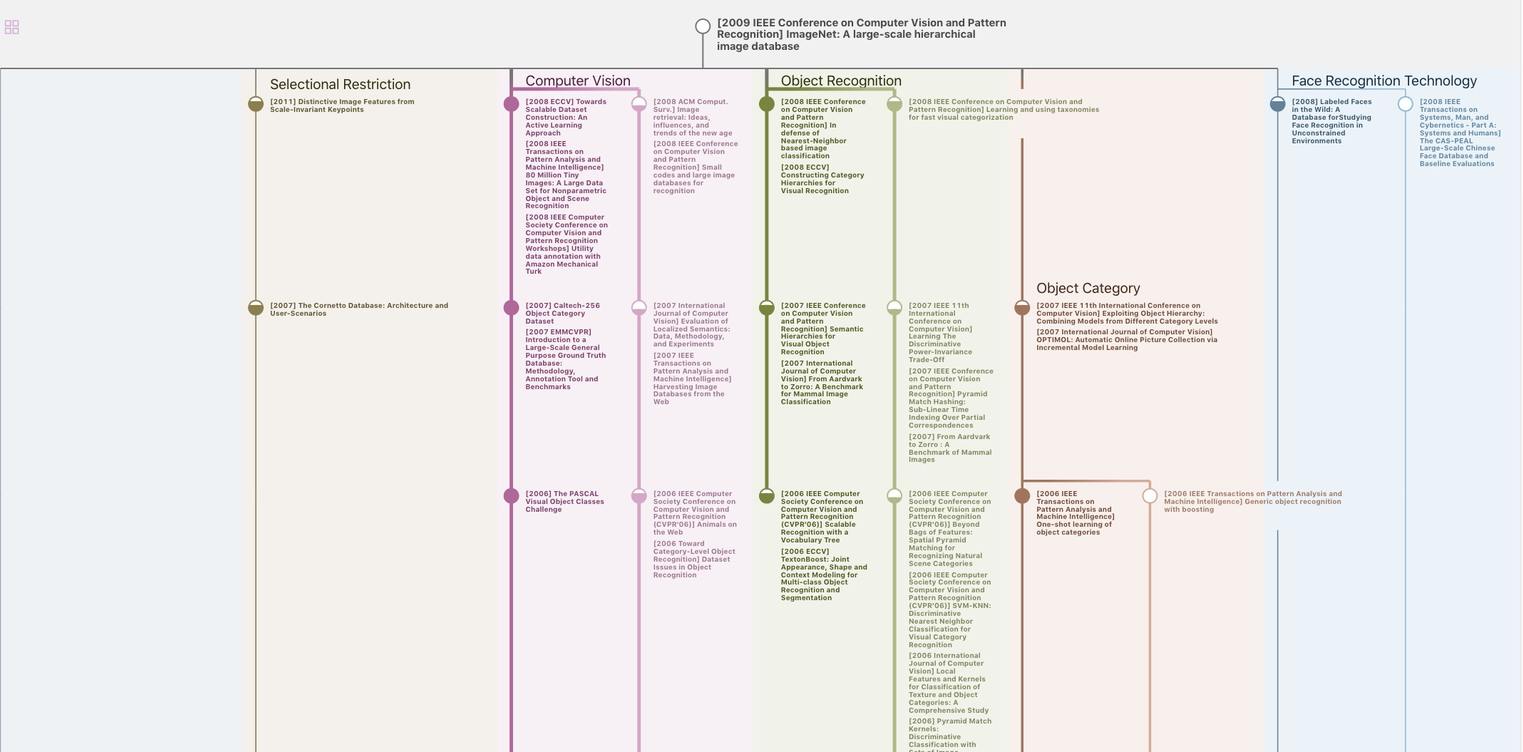
生成溯源树,研究论文发展脉络
Chat Paper
正在生成论文摘要