Learning from a Teacher using Unlabeled Data
CoRR(2019)
Abstract
Knowledge distillation is a widely used technique for model compression. We posit that the teacher model used in a distillation setup, captures relationships between classes, that extend beyond the original dataset. We empirically show that a teacher model can transfer this knowledge to a student model even on an {\it out-of-distribution} dataset. Using this approach, we show promising results on MNIST, CIFAR-10, and Caltech-256 datasets using unlabeled image data from different sources. Our results are encouraging and help shed further light from the perspective of understanding knowledge distillation and utilizing unlabeled data to improve model quality.
MoreTranslated text
AI Read Science
Must-Reading Tree
Example
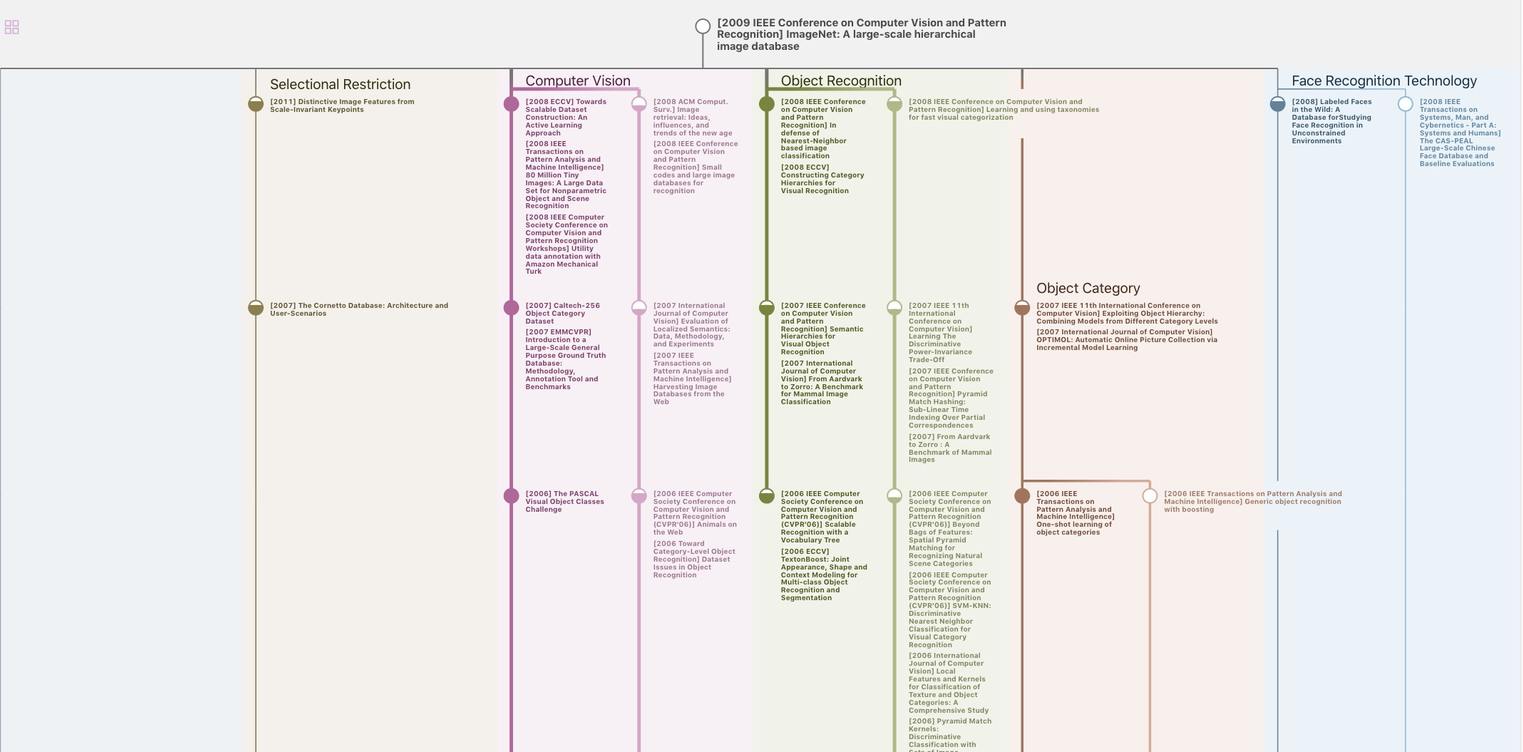
Generate MRT to find the research sequence of this paper
Chat Paper
Summary is being generated by the instructions you defined