Epithelium segmentation and automated Gleason grading of prostate cancer via deep learning in label-free multiphoton microscopic images.
JOURNAL OF BIOPHOTONICS(2020)
摘要
In the current clinical care practice, Gleason grading system is one of the most powerful prognostic predictors for prostate cancer (PCa). The grading system is based on the architectural pattern of cancerous epithelium in histological images. However, the standard procedure of histological examination often involves complicated tissue fixation and staining, which are time-consuming and may delay the diagnosis and surgery. In this study, label-free multiphoton microscopy (MPM) was used to acquire subcellular-resolution images of unstained prostate tissues. Then, a deep learning architecture (U-net) was introduced for epithelium segmentation of prostate tissues in MPM images. The obtained segmentation results were then merged with the original MPM images to train a classification network (AlexNet) for automated Gleason grading. The developed method achieved an overall pixel accuracy of 92.3% with a mean F1 score of 0.839 for epithelium segmentation. By merging the segmentation results with the MPM images, the accuracy of Gleason grading was improved from 72.42% to 81.13% in hold-out test set. Our results suggest that MPM in combination with deep learning holds the potential to be used as a fast and powerful clinical tool for PCa diagnosis.
更多查看译文
关键词
deep learning,epithelium segmentation,Gleason Grading,multiphoton microscopy,prostate cancer
AI 理解论文
溯源树
样例
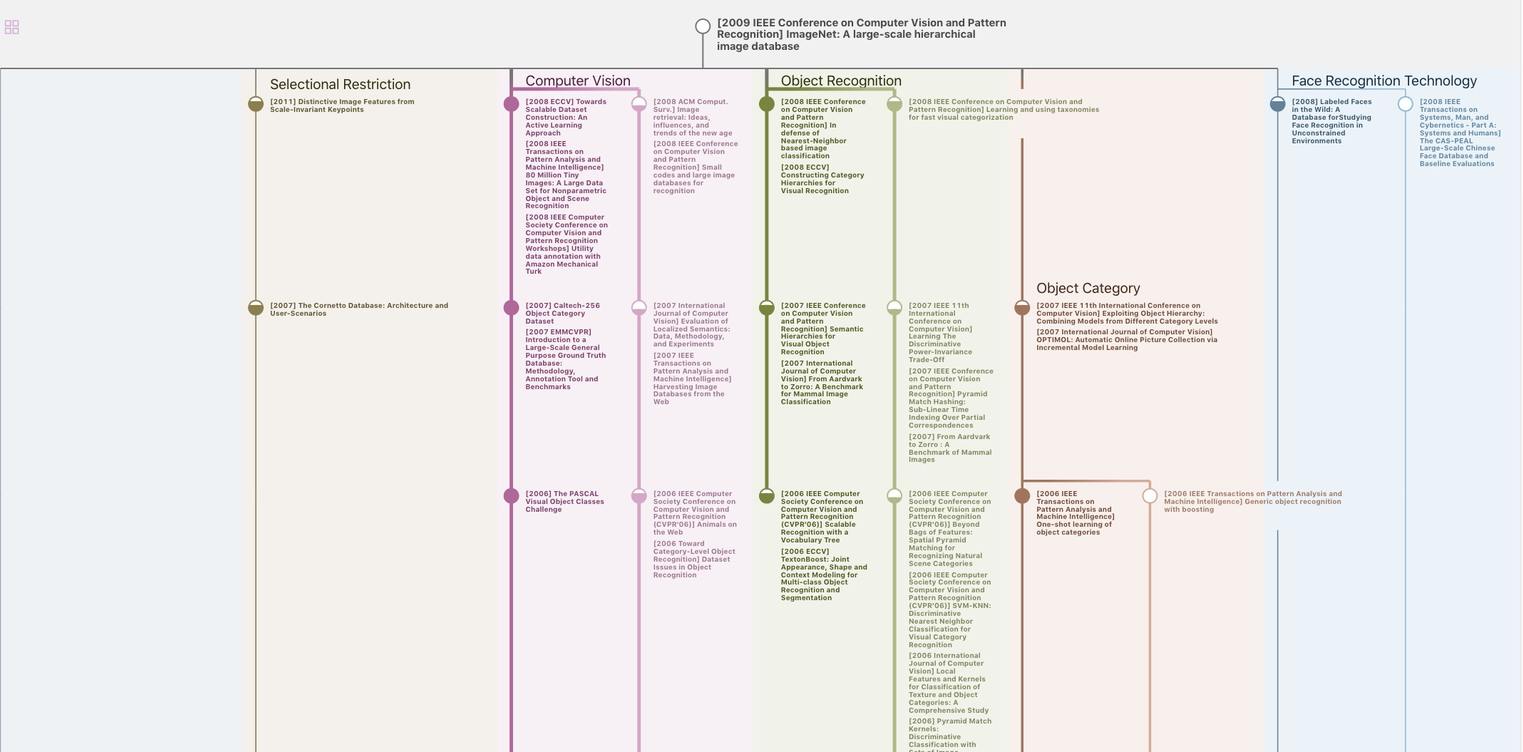
生成溯源树,研究论文发展脉络
Chat Paper
正在生成论文摘要