A Capsule Network-based Model for Learning Node Embeddings
CIKM '20: The 29th ACM International Conference on Information and Knowledge Management Virtual Event Ireland October, 2020(2020)
摘要
In this paper, we focus on learning low-dimensional embeddings for nodes in graph-structured data. To achieve this, we propose Caps2NE -- a new unsupervised embedding model leveraging a network of two capsule layers. Caps2NE induces a routing process to aggregate feature vectors of context neighbors of a given target node at the first capsule layer, then feed these features into the second capsule layer to infer a plausible embedding for the target node. Experimental results show that our proposed Caps2NE obtains state-of-the-art performances on benchmark datasets for the node classification task. Our code is available at: https://github.com/daiquocnguyen/Caps2NE.
更多查看译文
关键词
embeddings,learning,network-based
AI 理解论文
溯源树
样例
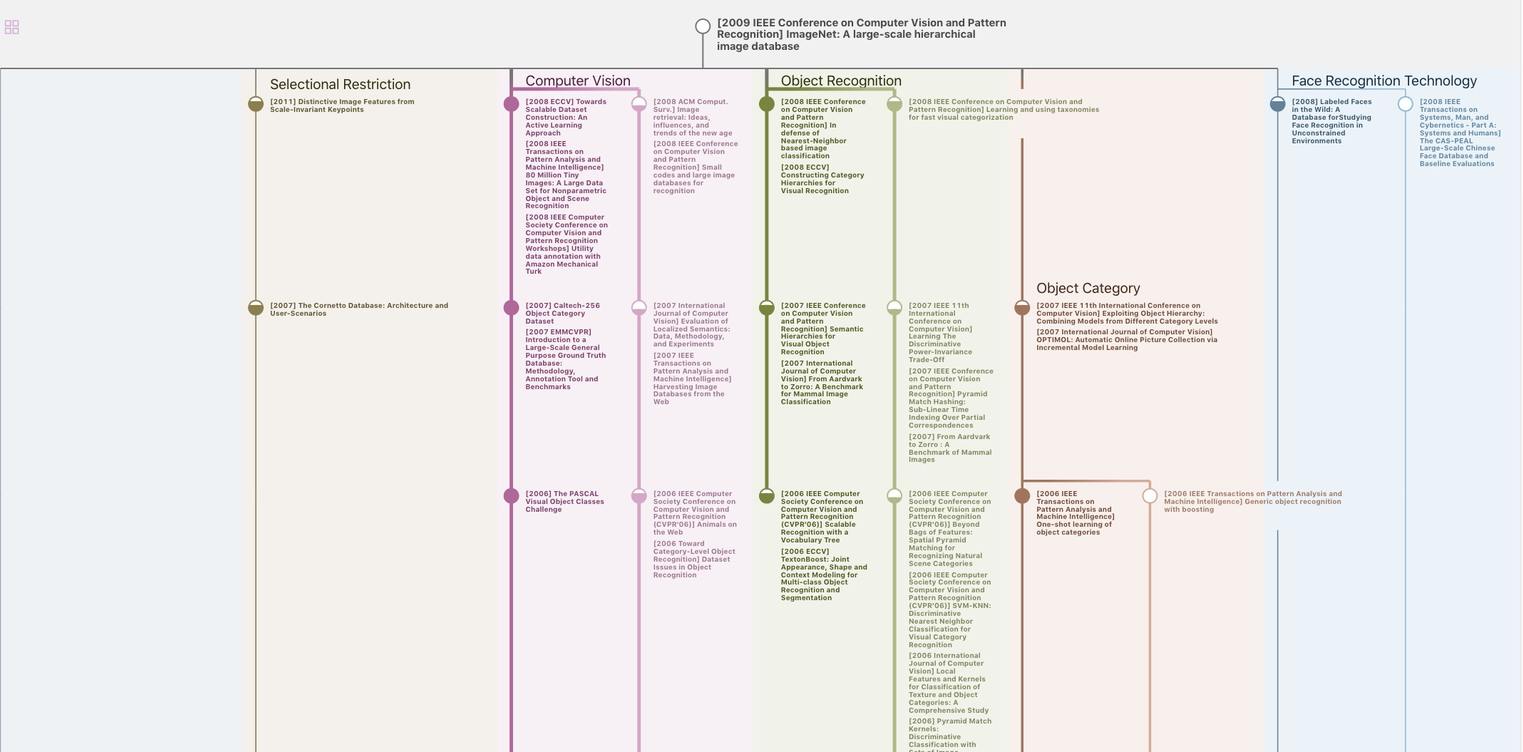
生成溯源树,研究论文发展脉络
Chat Paper
正在生成论文摘要