Generative Autoregressive Networks For 3d Dancing Move Synthesis From Music
IEEE ROBOTICS AND AUTOMATION LETTERS(2020)
摘要
This letter proposes a framework which is able to generate a sequence of three-dimensional human dance poses for a given music. The proposed framework consists of three components: a music feature encoder, a pose generator, and a music genre classifier. We focus on integrating these components for generating a realistic 3D human dancing move from music, which can be applied to artificial agents and humanoid robots. The trained dance pose generator, which is a generative autoregressive model, is able to synthesize a dance sequence longer than 1,000 pose frames. Experimental results of generated dance sequences from various songs show how the proposed method generates human-like dancing move to a given music. In addition, a generated 3D dance sequence is applied to a humanoid robot, showing that the proposed framework can make a robot to dance just by listening to music.
更多查看译文
关键词
Three-dimensional displays, Generators, Task analysis, Multiple signal classification, Skeleton, Training, Music, Gesture, posture and facial expressions, novel deep learning methods, entertainment robotics
AI 理解论文
溯源树
样例
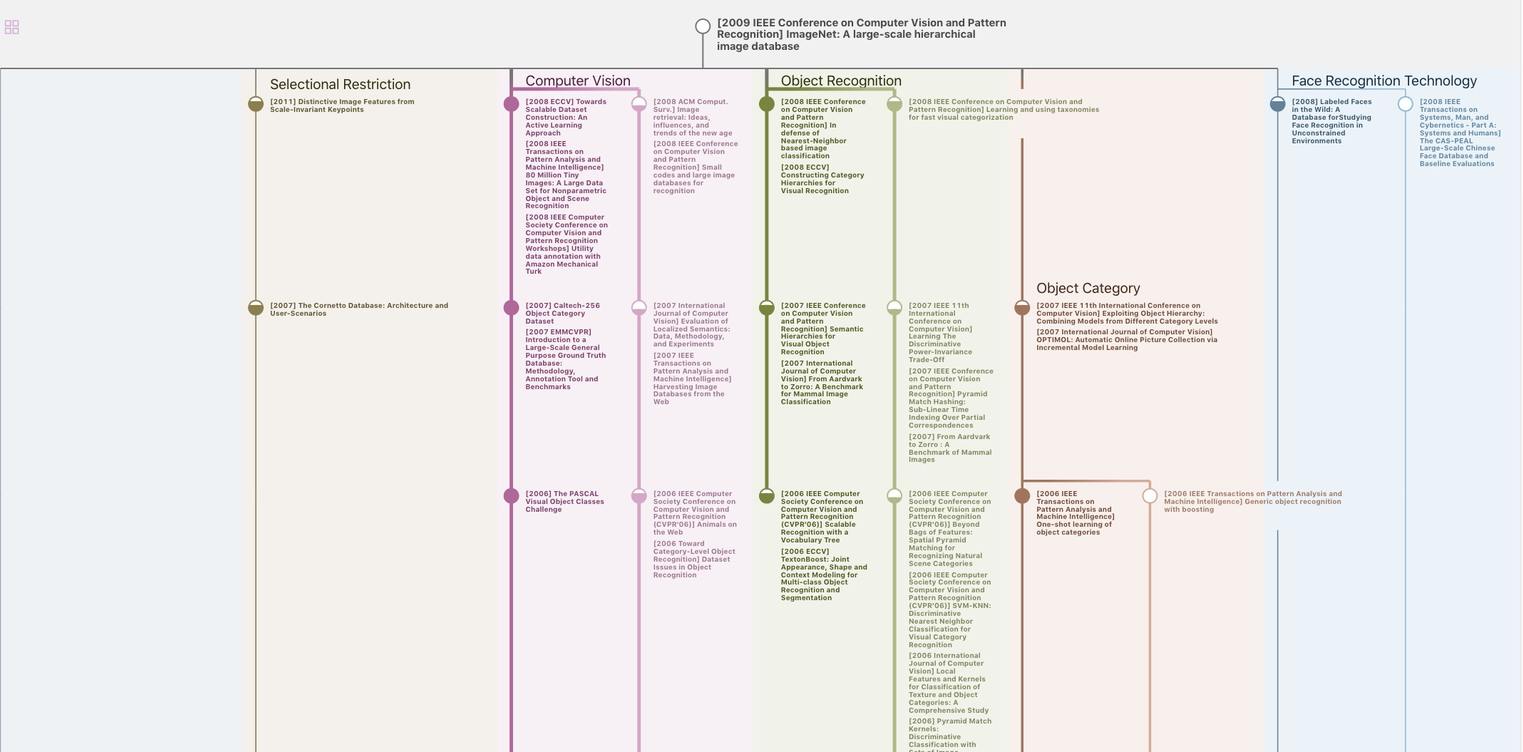
生成溯源树,研究论文发展脉络
Chat Paper
正在生成论文摘要