Location Attention For Extrapolation To Longer Sequences
58TH ANNUAL MEETING OF THE ASSOCIATION FOR COMPUTATIONAL LINGUISTICS (ACL 2020)(2020)
摘要
Neural networks are surprisingly good at interpolating and perform remarkably well when the training set examples resemble those in the test set. However, they are often unable to extrapolate patterns beyond the seen data, even when the abstractions required for such patterns are simple. In this paper, we first review the notion of extrapolation, why it is important, and how one could hope to tackle it. We then focus on a specific type of extrapolation, which is especially useful for natural language processing: generalization to sequences longer than those seen during training. We hypothesize that models with a separate content- and location-based attention are more likely to extrapolate than those with common attention mechanisms. We empirically support our claim for recurrent seq2seq models with our proposed attention on variants of the Lookup Table task. This sheds light on some striking failures of neural models for sequences and on possible methods to approaching such issues.
更多查看译文
AI 理解论文
溯源树
样例
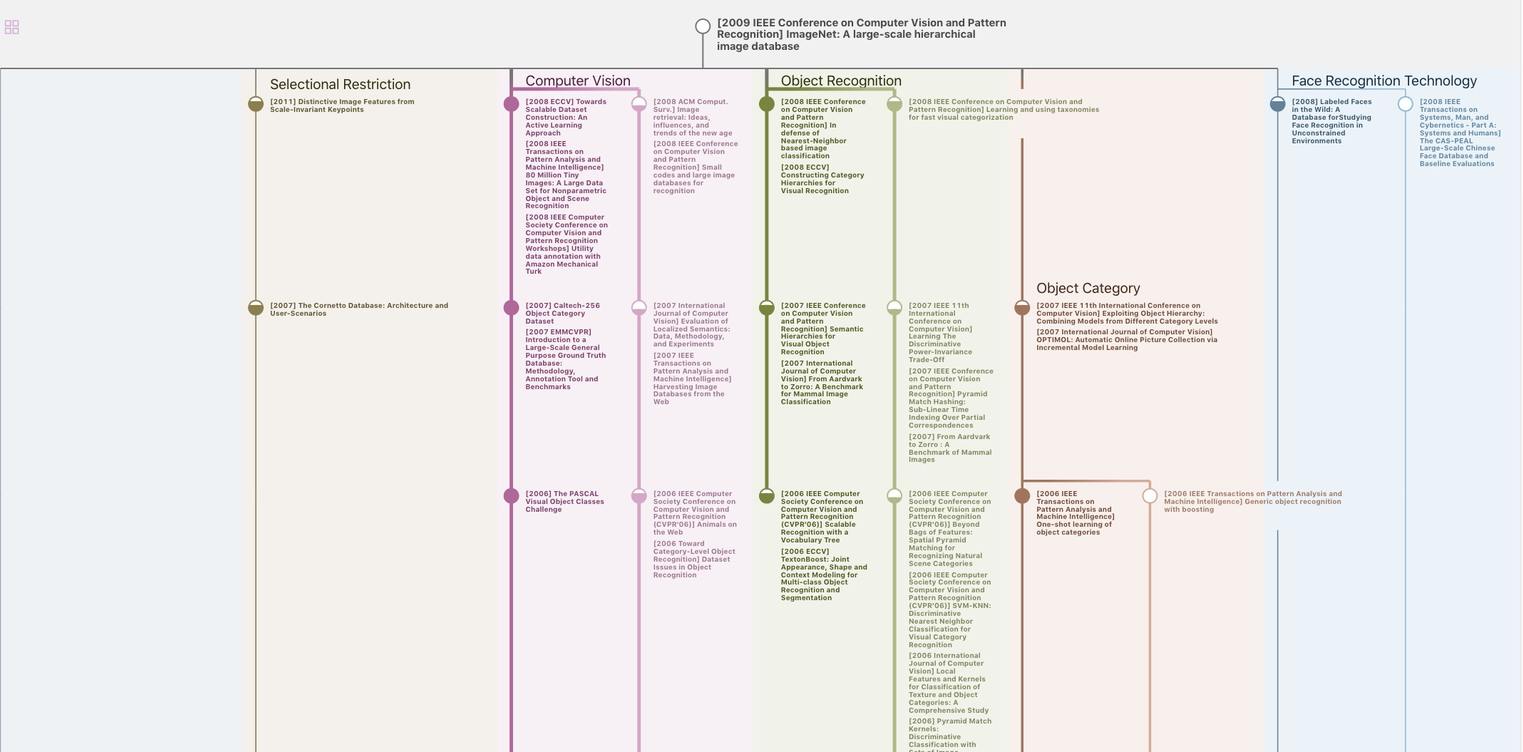
生成溯源树,研究论文发展脉络
Chat Paper
正在生成论文摘要