Queens are Powerful too: Mitigating Gender Bias in Dialogue Generation
Conference on Empirical Methods in Natural Language Processing(2020)
摘要
Social biases present in data are often directly reflected in the predictions of models trained on that data. We analyze gender bias in dialogue data, and examine how this bias is not only replicated, but is also amplified in subsequent generative chit-chat dialogue models. We measure gender bias in six existing dialogue datasets before selecting the most biased one, the multi-player text-based fantasy adventure dataset LIGHT, as a testbed for bias mitigation techniques. We consider three techniques to mitigate gender bias: counterfactual data augmentation, targeted data collection, and bias controlled training. We show that our proposed techniques mitigate gender bias by balancing the genderedness of generated dialogue utterances, and find that they are particularly effective in combination. We evaluate model performance with a variety of quantitative methods—including the quantity of gendered words, a dialogue safety classifier, and human assessments—all of which show that our models generate less gendered, but equally engaging chit-chat responses.
更多查看译文
关键词
dialogue generation,gender bias
AI 理解论文
溯源树
样例
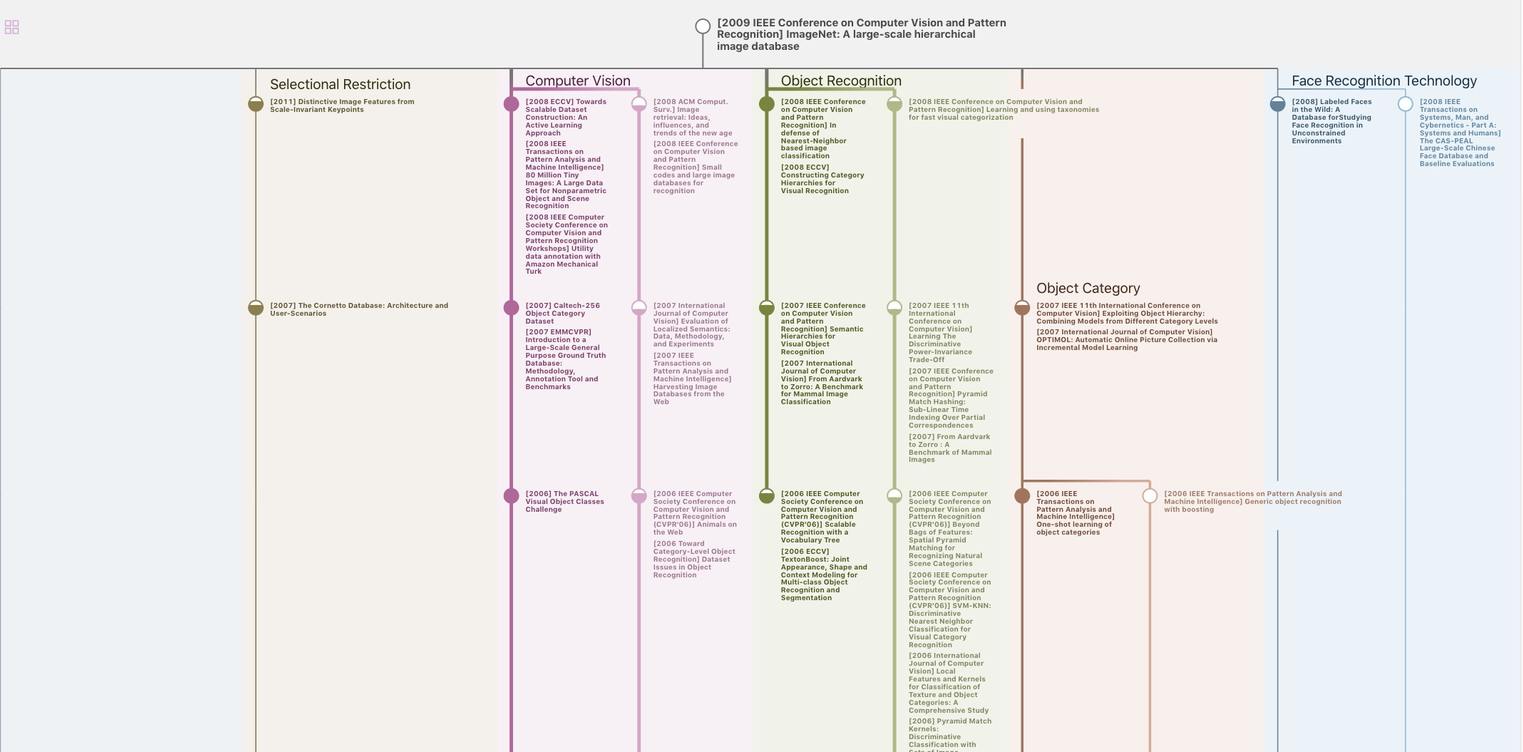
生成溯源树,研究论文发展脉络
Chat Paper
正在生成论文摘要