Hierarchical Reinforcement Learning Method for Autonomous Vehicle Behavior Planning
IROS(2020)
摘要
In this work, we propose a hierarchical reinforcement learning (HRL) structure which is capable of performing autonomous vehicle planning tasks in simulated environments with multiple sub-goals. In this hierarchical structure, the network is capable of 1) learning one task with multiple sub-goals simultaneously; 2) extracting attentions of states according to changing sub-goals during the learning process; 3) reusing the well-trained network of sub-goals for other similar tasks with the same sub-goals. The states are defined as processed observations which are transmitted from the perception system of the autonomous vehicle. A hybrid reward mechanism is designed for different hierarchical layers in the proposed HRL structure. Compared to traditional RL methods, our algorithm is more sample-efficient since its modular design allows reusing the policies of sub-goals across similar tasks. The results show that the proposed method converges to an optimal policy faster than traditional RL methods.
更多查看译文
关键词
behavior planning structure,hierarchical structure,multiple sub-goals,simulated environments,autonomous vehicle planning tasks,behavioral decision making,autonomous vehicle behavior planning,hierarchical reinforcement learning method,RL methods,HRL structure,hierarchical layers,hybrid reward mechanism,learning process
AI 理解论文
溯源树
样例
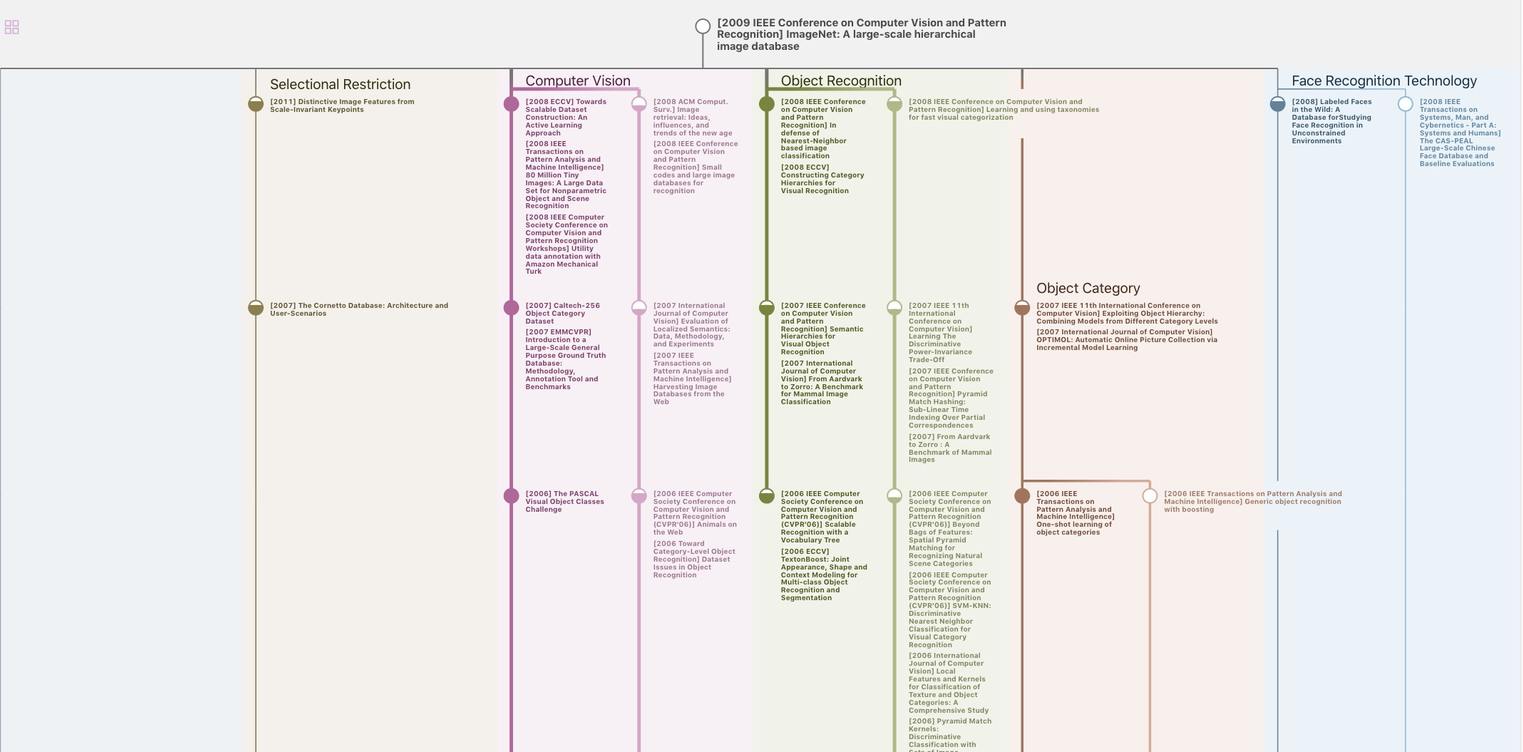
生成溯源树,研究论文发展脉络
Chat Paper
正在生成论文摘要