Beyond Statistical Relations - Integrating Knowledge Relations into Style Correlations for Multi-Label Music Style Classification.
PROCEEDINGS OF THE 13TH INTERNATIONAL CONFERENCE ON WEB SEARCH AND DATA MINING (WSDM '20)(2020)
摘要
Automatically labeling multiple styles for every song is a comprehensive application in all kinds of music websites. Recently, some researches explore review-driven multi-label music style classification and exploit style correlations for this task. However, their methods focus on mining the statistical relations between different music styles and only consider shallow style relations. Moreover, these statistical relations suffer from the underfitting problem because some music styles have little training data. To tackle these problems, we propose a novel knowledge relations integrated framework (KRF) to capture the complete style correlations, which jointly exploits the inherent relations between music styles according to external knowledge and their statistical relations. Based on the two types of relations, we use graph convolutional network to learn the deep correlations between styles automatically. Experimental results show that our framework significantly outperforms the state-of-the-art methods. Further studies demonstrate that our framework can effectively alleviate the underfitting problem and learn meaningful style correlations.
更多查看译文
关键词
music genre classification, label correlations, graph convolutional network, multi-label classification, knowledge graph application, deep learning
AI 理解论文
溯源树
样例
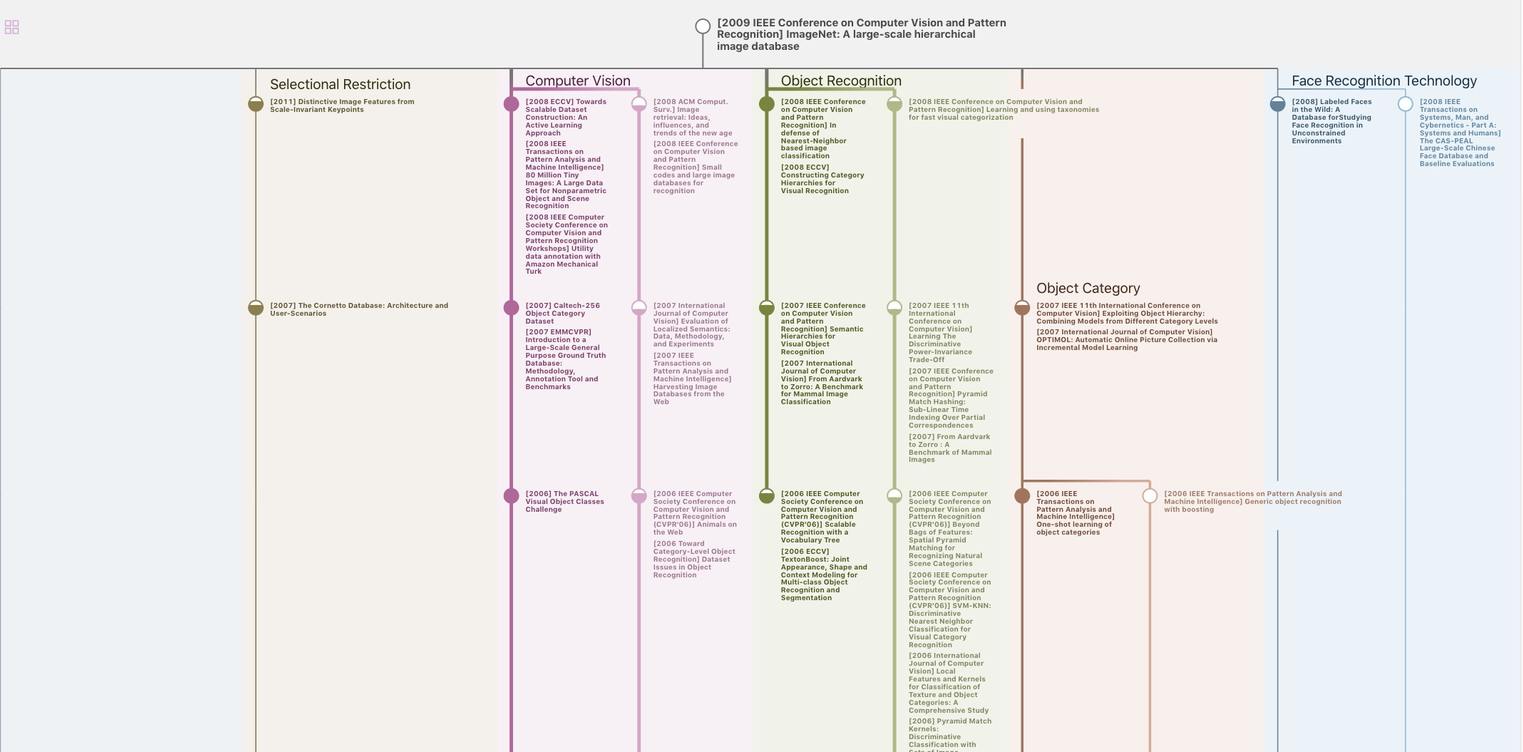
生成溯源树,研究论文发展脉络
Chat Paper
正在生成论文摘要