DZip: Improved General-Purpose Lossless Compression Based on Novel Neural Network Modeling
2020 Data Compression Conference (DCC)(2020)
摘要
We consider lossless compression based on statistical data modeling followed by prediction-based encoding, where an accurate statistical model for the input data leads to substantial improvements in compression. We propose DZip, a general-purpose compressor for sequential data that exploits the well-known modeling capabilities of neural networks (NNs) for prediction, followed by arithmetic coding. DZip uses a novel hybrid architecture based on adaptive and semi-adaptive training. Unlike most NN based compressors, DZip does not require additional training data and is not restricted to specific data types, only needing the alphabet size of the input data. The proposed compressor outperforms general-purpose compressors such as Gzip (on average 26% reduction) on a variety of real datasets, achieves near-optimal compression on synthetic datasets, and performs close to specialized compressors for large sequence lengths, without any human input. The main limitation of DZip in its current implementation is the encoding/decoding time, which limits its practicality. Nevertheless, the results showcase the potential of developing improved general-purpose compressors based on neural networks and hybrid modeling.
更多查看译文
关键词
statistical model,DZip,general-purpose compressor,sequential data,modeling capabilities,semiadaptive training,NN based compressors,additional training data,data types,near-optimal compression,specialized compressors,hybrid modeling,improved general-purpose lossless compression,neural network modeling,statistical data,prediction-based encoding
AI 理解论文
溯源树
样例
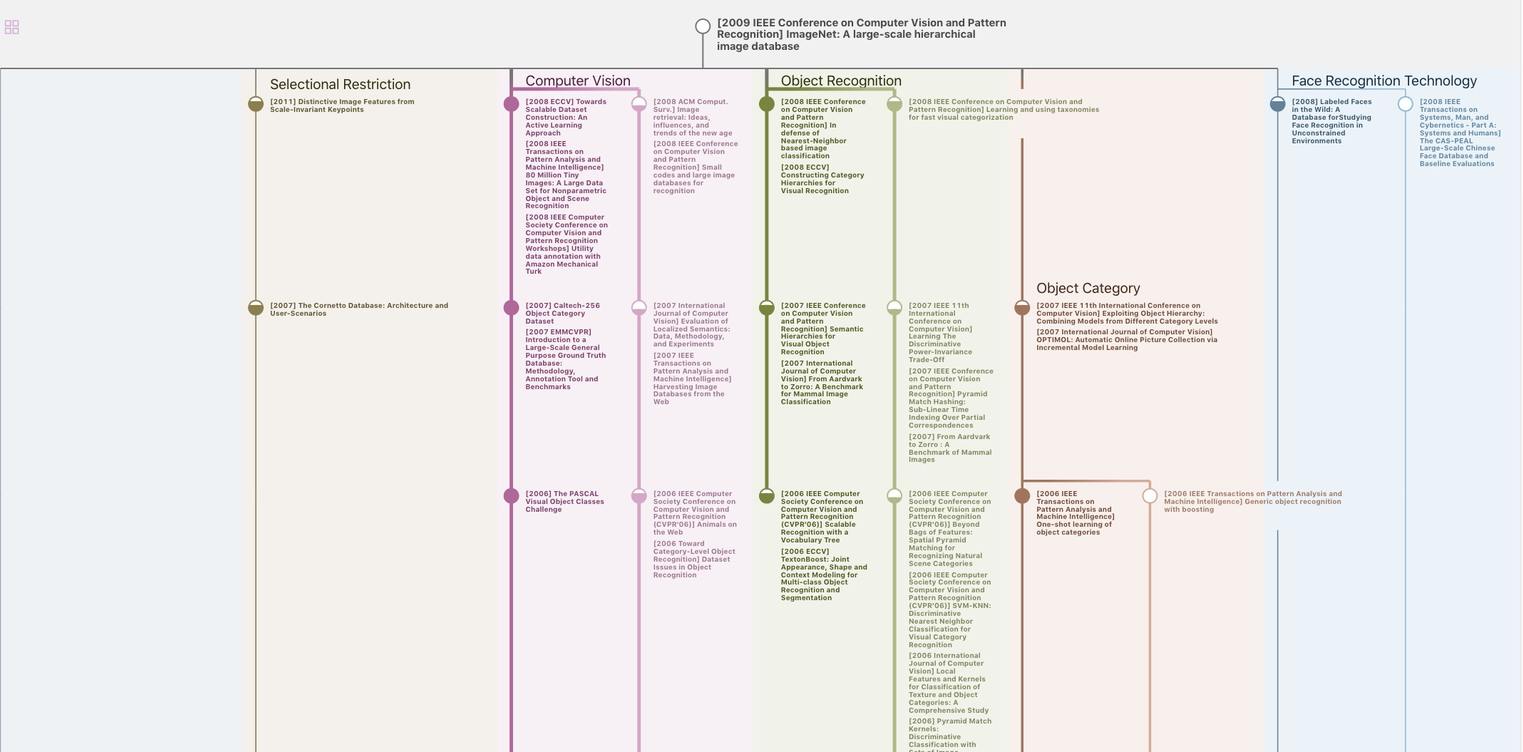
生成溯源树,研究论文发展脉络
Chat Paper
正在生成论文摘要